Full-Mode-Augmentation Tensor-Train Rank Minimization for Hyperspectral Image Inpainting
IEEE TRANSACTIONS ON GEOSCIENCE AND REMOTE SENSING(2024)
摘要
Hyperspectral image (HSI) inpainting is a fundamental task in remote sensing image processing, which is helpful for subsequent applications such as classification and unmixing. Recently, tensor-train decomposition (TTD)-based low-rank methods have achieved great success in image inpainting because of the balanced tensor unfolding and the use of tensor augmentation (TA). However, the TTD algorithm only performs TA on the third mode, and cannot effectively mine the spectral domain information of HSIs. Aiming at this problem, this article extends TA to each mode of the tensor and proposes a full-mode-augmentation TT decomposition (FTTD). More precisely, the HSI is cast along each mode to get a 3-D N th-order tensor sequence, and all the tensors in the sequence are performed TTD to get $3N$ factor tensors that model the correlation on various modes. Then, the full-mode-augmentation tensor unfolding method is given by performing TT unfolding on each $N$ th-order tensor. We implement FTTD by minimizing the rank of the unfolding matrix and thus provide the tensor completion framework based on full-mode-augmentation tensor-train rank minimization. Finally, using the framework, we optimize the current two classical iterative algorithms nuclear norm minimization and parallel matrix decomposition. These two algorithms utilize the unfolding matrices in the framework to effectively mine spatial and spectral information, thereby making the restored tensor more approximate to the original HSI. Experiments on various HSIs have shown that the proposed method outperforms compared methods in terms of visual and quantitative measures.
更多查看译文
关键词
Full-mode-augmentation,hyperspectral image (HSI) inpainting,low-rank tensor completion,tensor augmentation (TA),tensor-train
AI 理解论文
溯源树
样例
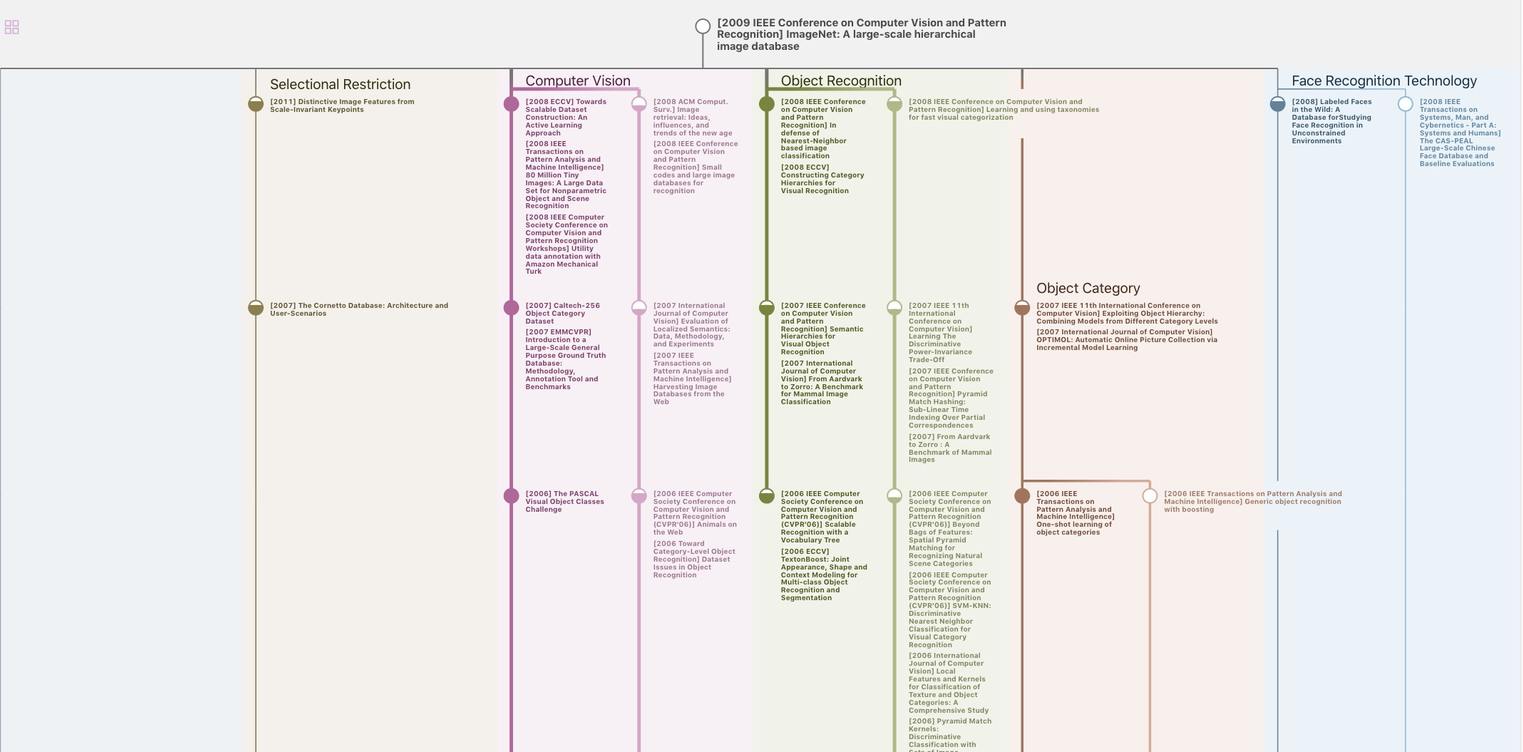
生成溯源树,研究论文发展脉络
Chat Paper
正在生成论文摘要