MLPCC-MLMAE-Based Early Stopping Strategy for Unsupervised 3-D Seismic Data Reconstruction
IEEE GEOSCIENCE AND REMOTE SENSING LETTERS(2024)
摘要
Unsupervised methods for single 3-D seismic data reconstruction, such as deep image prior (DIP) and Bernoulli sampling (BS) frameworks, have achieved promising results. However, they suffer from expensive computational costs caused by a large number of iterations. Moreover, in the absence of labels, the final reconstructed results often require visual inspection to pick out, which inevitably introduces subjective errors. To address the above problems, we introduce local Pearson correlation coefficient (LPCC) and local mean absolute error (LMAE) to assess the correlation and difference between seismic traces. The mean values of LPCC (MLPCC) and LMAE (MLMAE) for all nonedge traces in the reconstructed result can evaluate the quality of the reconstructed result based on the internal similarity and internal difference of 3-D seismic data without labels. We further develop an MLPCC-MLMAE-based early stopping strategy. The training process will stop once the number of values behind the highest MLPCC has reached the patience value, and the MLMAE is used as one of the indispensable constraints for the highest MLPCC. We apply the proposed early stopping strategy to the DIP and BS frameworks and demonstrate that it has the ability to significantly reduce computational costs through experiments on synthetic and field data, which makes the DIP and BS frameworks a major step toward practical production. Furthermore, the quantitative evaluation metrics allow the network to automatically and intelligently monitor the training process, and the prediction at the end of iteration is used as the final reconstructed result, thus eliminating the need for human intervention.
更多查看译文
关键词
3-D seismic data,early stopping strategy,reduced computational costs,unsupervised reconstruction
AI 理解论文
溯源树
样例
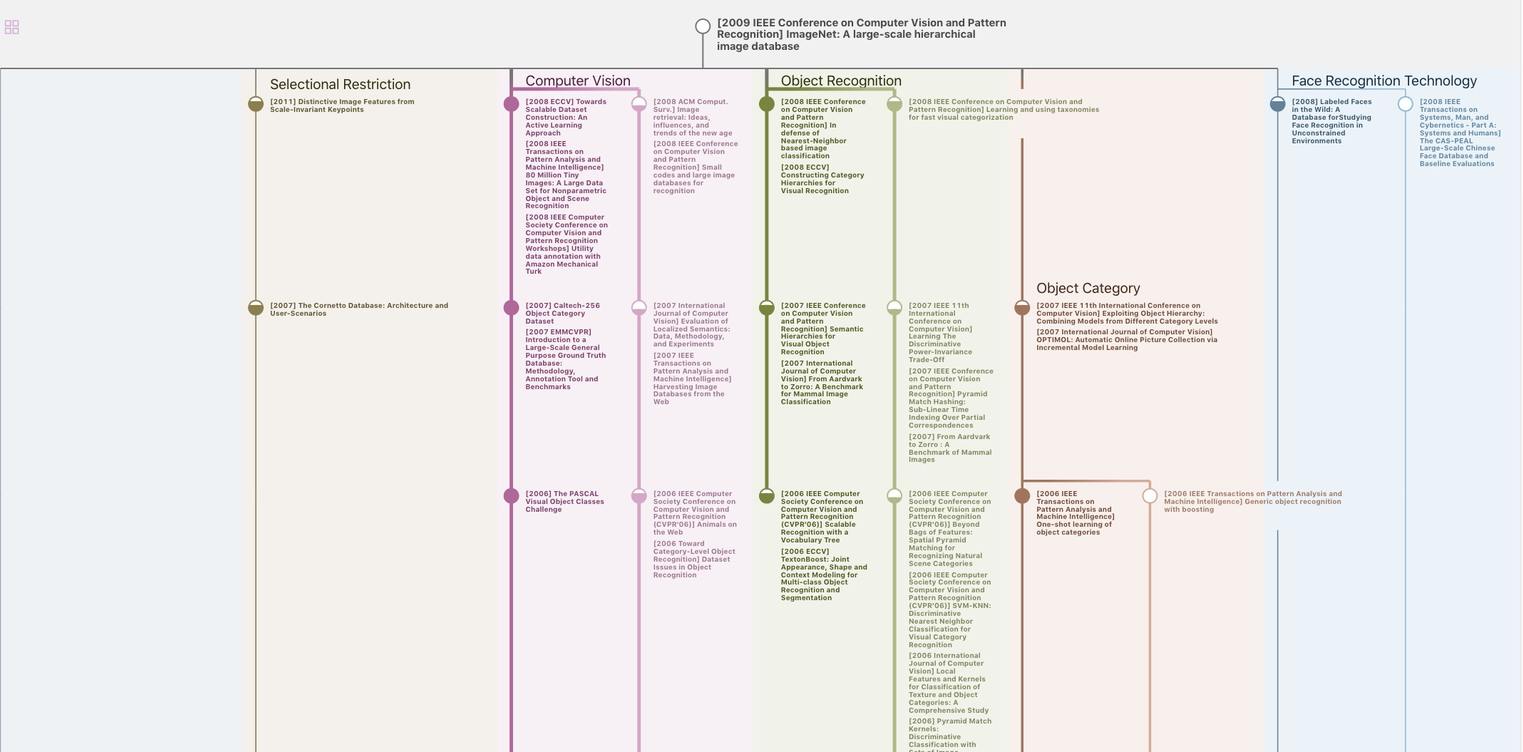
生成溯源树,研究论文发展脉络
Chat Paper
正在生成论文摘要