SCED-Net: Coupling Dual-Branch and Encoder-Decoder Network for Identification and Delineation of Municipal Solid Waste From VHR Images
IEEE GEOSCIENCE AND REMOTE SENSING LETTERS(2024)
摘要
Monitoring municipal solid waste (MSW) with remote sensing images is of great significance to the urban environment and the well-being of city residents. Existing studies have employed very high-resolution (VHR) images for identifying MSWs. However, automating such a task is challenging due to the complex and irregular spectra and geometry characteristics of MSWs. In this letter, a novel approach that adopts a dual-branch network and an encoder-decoder architecture, named SCED-Net, to improve the identification and delineation of MSW is proposed. First, a dual-branch network consisting of a convolutional neural network (CNN) and a Swin-Transformer (S-T) is proposed for classifying image patches and improving the accuracy of MSW identification. Second, an encoder-decoder architecture, in which the encoder shares the above dual-branch network while the decoder uses upsampling layers, is proposed for segmenting image patches and delineating MSW boundaries. The proposed method was applied to identifying and delineating MSW boundaries in Guangzhou City, Guangdong Province, China. Evaluations showed that our proposed network achieved an overall accuracy (OA) of 87.6% in MSW identification, and a pixel accuracy (PA) of 96.2% in MSW delineation, significantly better than existing methods. Our code and dataset are available at https://github.com/GUO-Zhou/SCED-Net-for-MSW-Extraction.
更多查看译文
关键词
Deep learning,dual branch network,municipal solid waste (MSW),remote sensing image
AI 理解论文
溯源树
样例
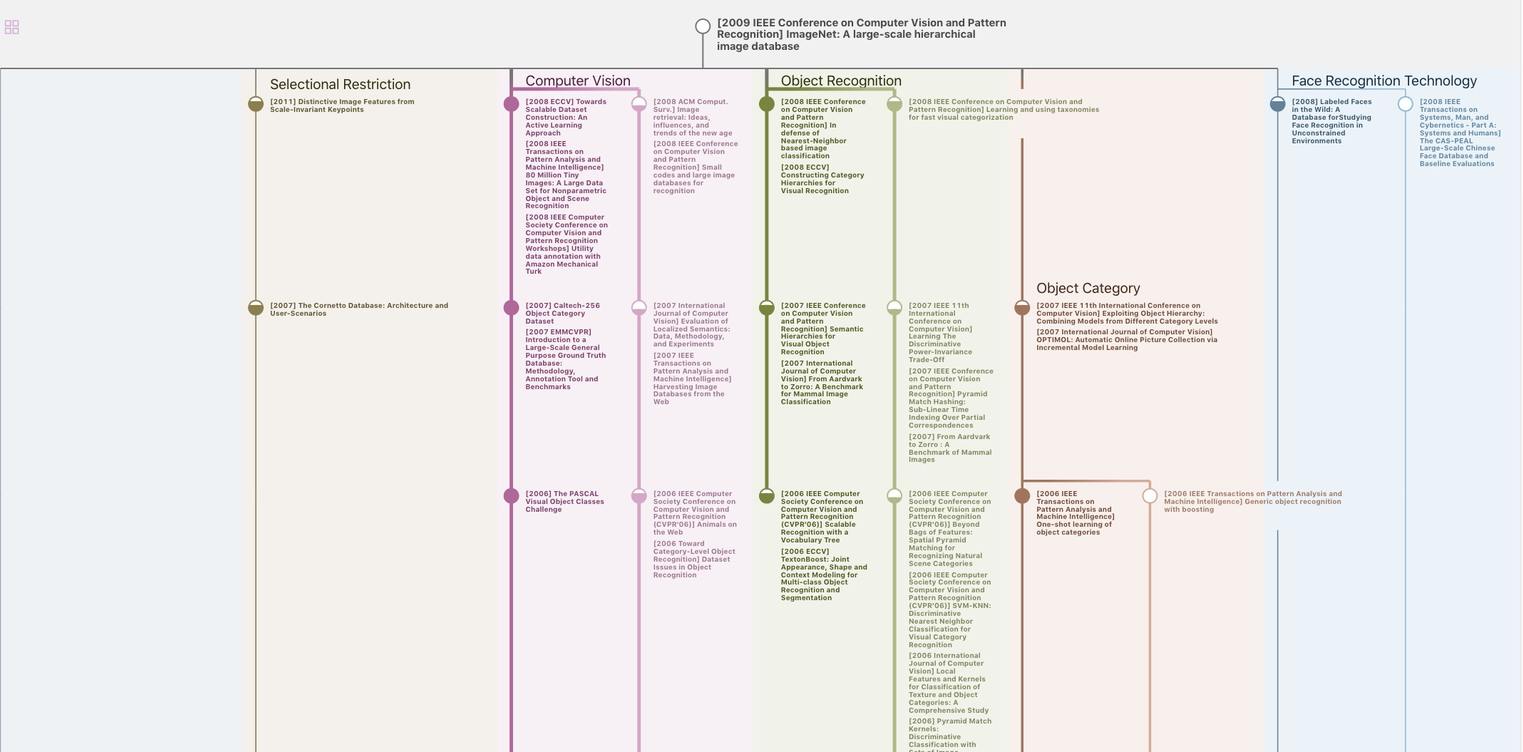
生成溯源树,研究论文发展脉络
Chat Paper
正在生成论文摘要