Weakly Supervised Learning for Pixel-Level Sea Ice Concentration Extraction Using AI4Arctic Sea Ice Challenge Dataset
IEEE GEOSCIENCE AND REMOTE SENSING LETTERS(2024)
Abstract
High-resolution sea ice concentration (SIC) maps are critical to support various applications, e.g., climate modeling, ship navigation, and activities in Northern communities. However, operational mapping of SIC based on expert annotations is coarse in spatial resolution and time-consuming to prepare. Although many convolutional neural network (CNN)-based methods have been proposed for automated sea ice mapping from synthetic aperture radar (SAR) imagery in recent years, the lack of pixel-based labels for model training hinders them from producing high-resolution reliable mapping results. To overcome this challenge, this letter presents a novel weakly supervised learning approach that generates pixel-level SIC prediction using coarse region/polygon-level SIC ground truth. Specifically, a novel region-level loss function is designed to enable direct use of regional/polygon SIC values in ice charts for the training of a U-Net-based model. This avoids the errors in transferring region-level SIC values to pixel-level ground-truth SIC values effectively and allows the generation of pixel-level SIC and sea ice extent (SIE) estimates. The proposed approach is evaluated on the recently published AI4Arctic Sea Ice Challenge Dataset with over 500 Sentinel-1 SAR scenes, ancillary data, and associated ice charts. The results demonstrate the effectiveness of the weakly supervised model in producing pixel-level high-resolution SIC maps that are consistent with ice charts and visual interpretation.
MoreTranslated text
Key words
AI4Arctic dataset,convolutional neural networks (CNNs),sea ice concentration (SIC),super-resolution,weakly supervised learning
AI Read Science
Must-Reading Tree
Example
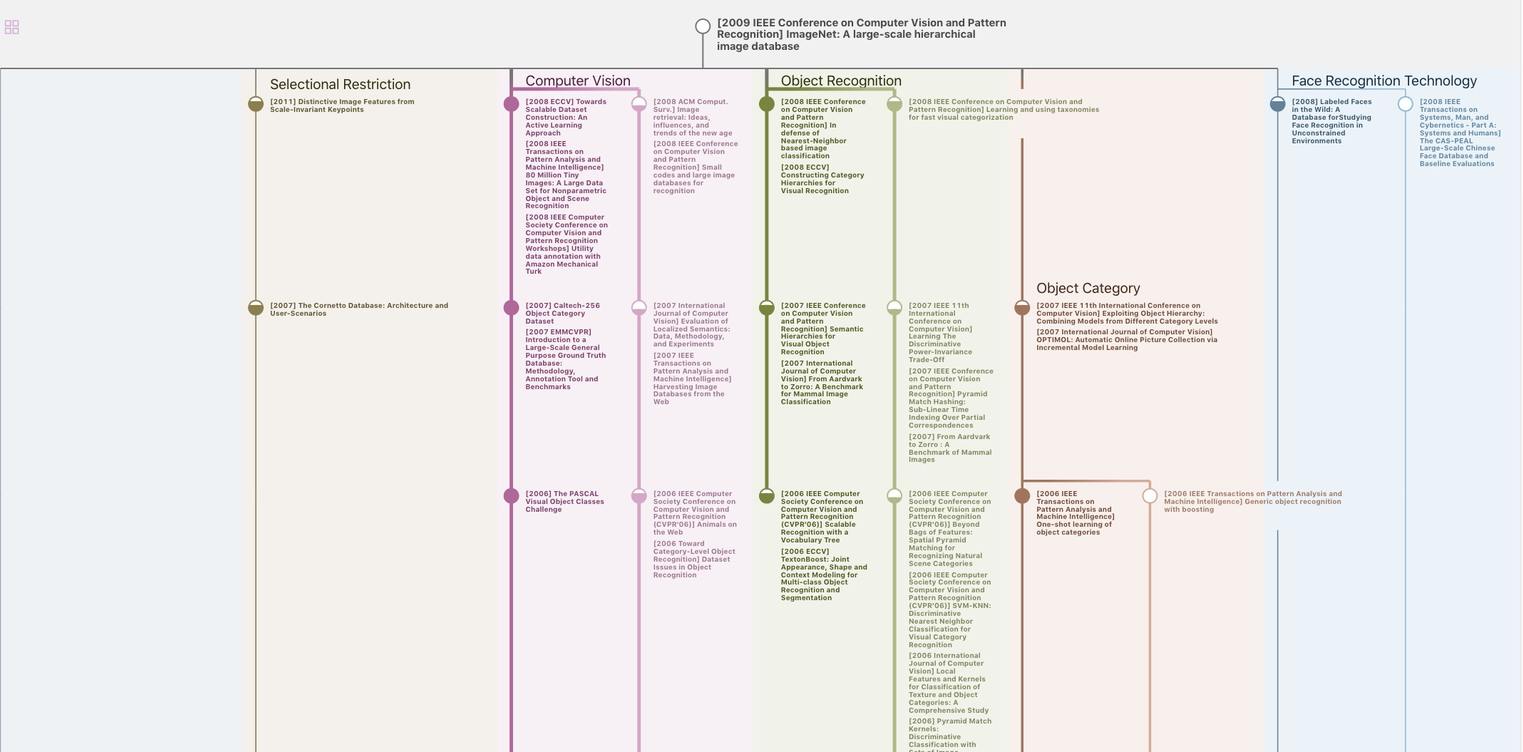
Generate MRT to find the research sequence of this paper
Chat Paper
Summary is being generated by the instructions you defined