Enhancing Time Series Representation Learning with Spatiotemporal Dynamic Encoding
2023 IEEE INTERNATIONAL CONFERENCE ON WEB INTELLIGENCE AND INTELLIGENT AGENT TECHNOLOGY, WI-IAT(2023)
摘要
With the proliferation of time series data across diverse domains, achieving accurate and high-performance time series representation has become paramount. The intricacies of large-scale datasets characterized by nonlinear and heterogeneous distributions have prompted the exploration of attention models, such as the Transformer architecture, in time series analysis. However, these models often neglect temporal positional relationships among sequences, leading to suboptimal capture of long-term dependencies. This paper introduces an innovative approach that integrates temporal decay positional encoding with ordinary differential equations (ODEs) to formulate decaying position encodings, thereby enhancing time series representation. Additionally, a Spatial Dynamic Correlation Subgraph Module (SDCSM) is proposed to dynamically capture evolving interactions among observations. The presented research significantly boosts representation performance, while also addressing training time and memory space constraints, making it applicable across domains reliant on precise time series representation.
更多查看译文
关键词
Time Series Representation Learning,Temporal Position Encoding,Temporal Decay,Multi-Head Attention Mechanism,Dynamic Correlation Subgraphs
AI 理解论文
溯源树
样例
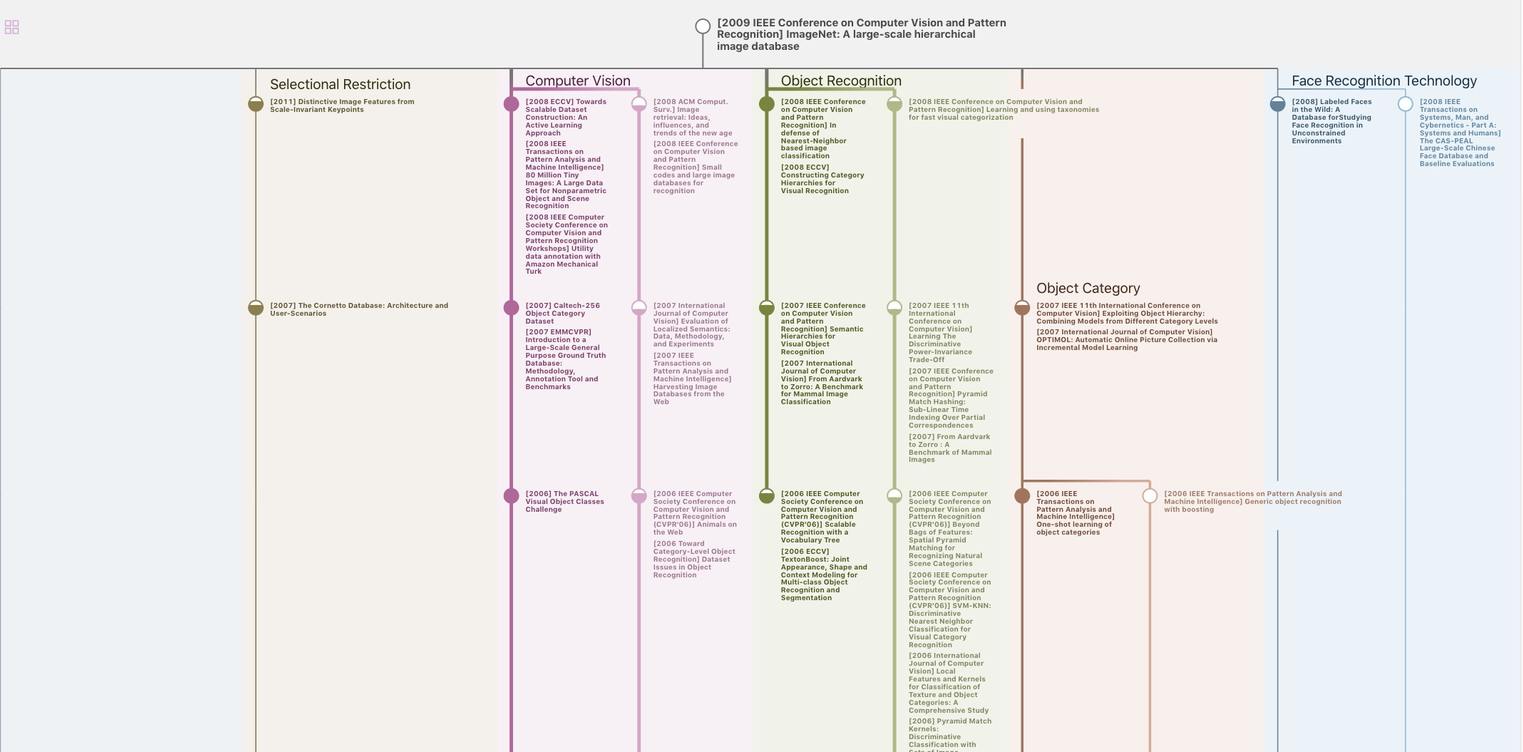
生成溯源树,研究论文发展脉络
Chat Paper
正在生成论文摘要