K-STTN: Knowledge-induced Spatio-Temporal Transformer Networks for Traffic Forecasting
2023 IEEE INTERNATIONAL CONFERENCE ON WEB INTELLIGENCE AND INTELLIGENT AGENT TECHNOLOGY, WI-IAT(2023)
摘要
Accurate traffic forecasting is essential to improve traffic efficiency, ensure urban traffic safety, and promote sustainable urban development. In recent years, extensive deep-learning methods have been proposed to deal with the highly nonlinear and dynamic spatio-temporal dependencies in traffic forecasting problems. However, few of them have considered the underlying correlations between different traffic factors, such as the correlation between traffic flow and speed. Based on knowledge derived from the field of transportation, in this paper, we propose a knowledge-induced spatio-temporal transformer Network (K-STTN) to simultaneously forecast both traffic flow and speed. By introducing Greenshields' traffic model in a spatio-temporal transformer network, we aim to automatically investigate both spatio-temporal dependencies and flow-speed correlations in an end-to-end learning manner. To evaluate the performance of the proposed K-STTN model, we conduct experiments on two California highway public datasets (i.e., PeMS04 and PeMS08). Experimental results show that the K-STTN model outperforms the state-of-the-art baseline models in terms of forecasting accuracy. In summary, the practice of knowledge-induced neural networks in this paper offers new insights into the design of neural networks by integrating knowledge from specific domains.
更多查看译文
关键词
Traffic forecasting,Spatio-temporal transformer networks,Spatio-temporal dependencies,Greenshields' traffic model
AI 理解论文
溯源树
样例
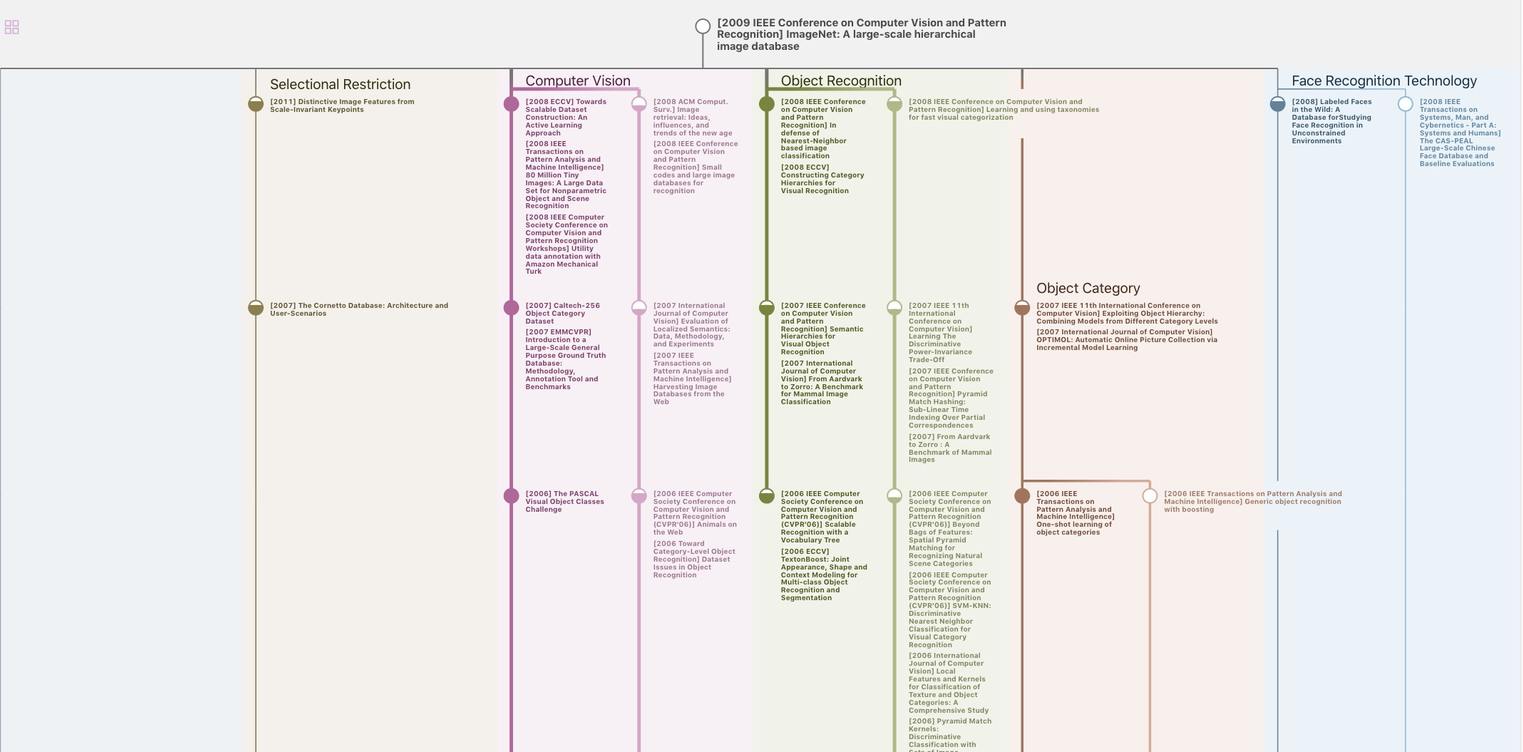
生成溯源树,研究论文发展脉络
Chat Paper
正在生成论文摘要