MKGS: Maintains the original structure of Knowledge Graph
2023 IEEE INTERNATIONAL CONFERENCE ON WEB INTELLIGENCE AND INTELLIGENT AGENT TECHNOLOGY, WI-IAT(2023)
摘要
In the field of graph-generated text, one of the core issues commonly explored is how to maintain the structural information of the graph to the maximum extent and reduce the problem of knowledge loss during training. Current research has mainly focused on exploring the ability of models to learn graph structures by increasing model size and refining the graph representation. In contrast, our work emphasizes the importance of perceiving and exploring edges in the graph itself. Edges provide a wide variety of structures to the graph structure, offering it freedom and diversity. Therefore, improving the model's ability to perceive edges could potentially enhance the task metrics of graph generation for text. To address this, we propose a graph-generated text model MKGS that maintains the original structure of the knowledge graph, effectively reducing knowledge loss during the learning process. Our approach achieves this at three levels: reorganizing the knowledge sequence as input to the model, enhancing edge perception during processing, and incorporating a graph rational activation function at the output. We validate our method using the Kg-to-text benchmark dataset WebNLG, where MKGS achieves a score of 66.22%. Additionally, the model exhibits fewer syntactic errors and produces smoother expressions in the generated text.
更多查看译文
关键词
Graph-generated Text,Knowledge Representation,Knowledge Graphs,Graph Theory,Edge-aware Attention
AI 理解论文
溯源树
样例
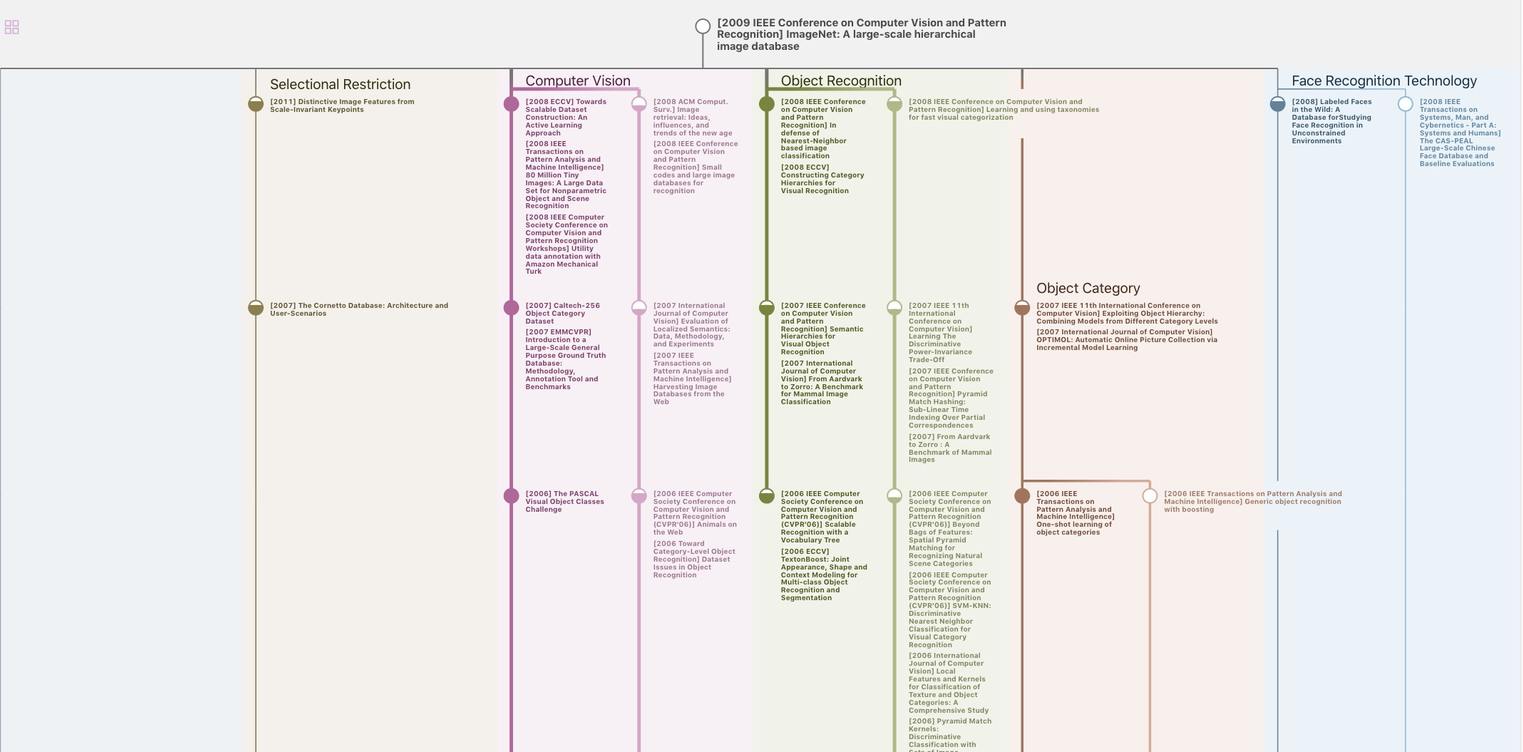
生成溯源树,研究论文发展脉络
Chat Paper
正在生成论文摘要