Training multi-source domain adaptation network by mutual information estimation and minimization
NEURAL NETWORKS(2024)
摘要
We address the problem of Multi-Source Domain Adaptation (MSDA), which trains a neural network using multiple labeled source datasets and an unlabeled target dataset, and expects the trained network to well classify the unlabeled target data. The main challenge in this problem is that the datasets are generated by relevant but different joint distributions. In this paper, we propose to address this challenge by estimating and minimizing the mutual information in the network latent feature space, which leads to the alignment of the source joint distributions and target joint distribution simultaneously. Here, the estimation of the mutual information is formulated into a convex optimization problem, such that the global optimal solution can be easily found. We conduct experiments on several public datasets, and show that our algorithm statistically outperforms its competitors. Video and code are available at https://github.com/sentaochen/ Mutual-Information-Estimation-and-Minimization.
更多查看译文
关键词
Convex optimization,Multi-source domain adaptation,Mutual information,Statistical learning
AI 理解论文
溯源树
样例
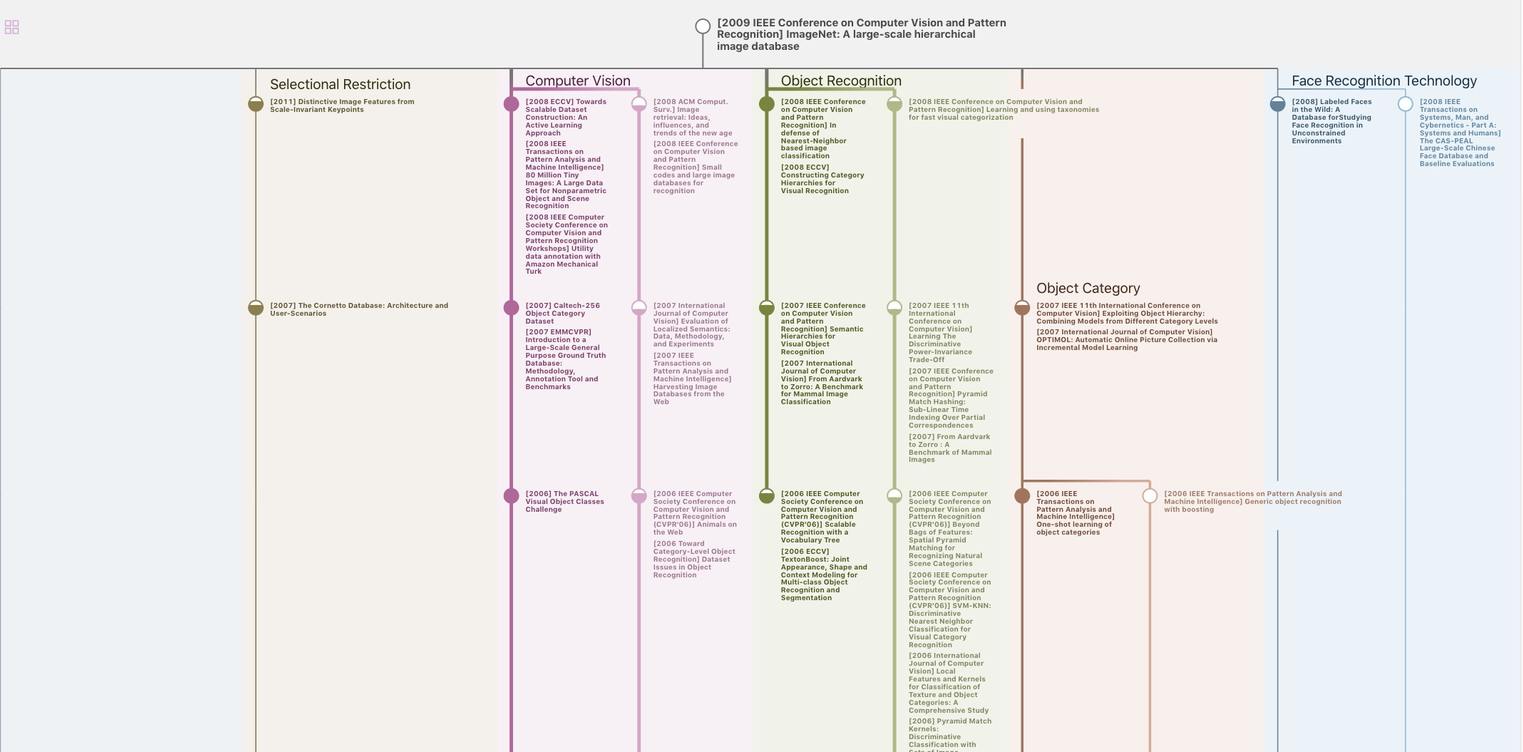
生成溯源树,研究论文发展脉络
Chat Paper
正在生成论文摘要