Exploring the resilience of supplementary cementitious materials-based concrete to elevated temperatures via modern computing techniques
MATERIALS TODAY COMMUNICATIONS(2024)
Abstract
Researchers are focused on the production of sustainable materials in order to reduce the negative environmental impact of conventional concrete. Utilizing industrial by-products as alternative cementitious materials in concrete is an appropriate strategy for promoting sustainability in construction. This research employed supervised machine learning techniques, including gradient boosting (GB), random forest (RF), and extreme gradient boosting (X-GB), in order to predict the compressive strength of sustainable concrete when exposed to high temperatures. SHapley Additive exPlanation (SHAP) analysis provided input component relevance. The comparison of these methodologies indicated that X-GB performed remarkably well and had a superior R2 value of 0.94 when compared to GB and RF. The results were further supported by a Taylor diagram, which demonstrated that the X-GB model best fitted the data, surpassing both the GB and RF models. This study's findings demonstrated the potential of machine learning, and more particularly X-GB, for making accurate predictions of compressive strength in high-temperature concrete applications. The results of SHAP analysis may be useful for selecting the proportions of raw materials in the mix design to achieve the desired properties.
MoreTranslated text
Key words
Supplementary cementitious materials,Elevated temperature,Compressive strength,SHapley Additive exPlanation (SHAP) analysis,Resilient material
AI Read Science
Must-Reading Tree
Example
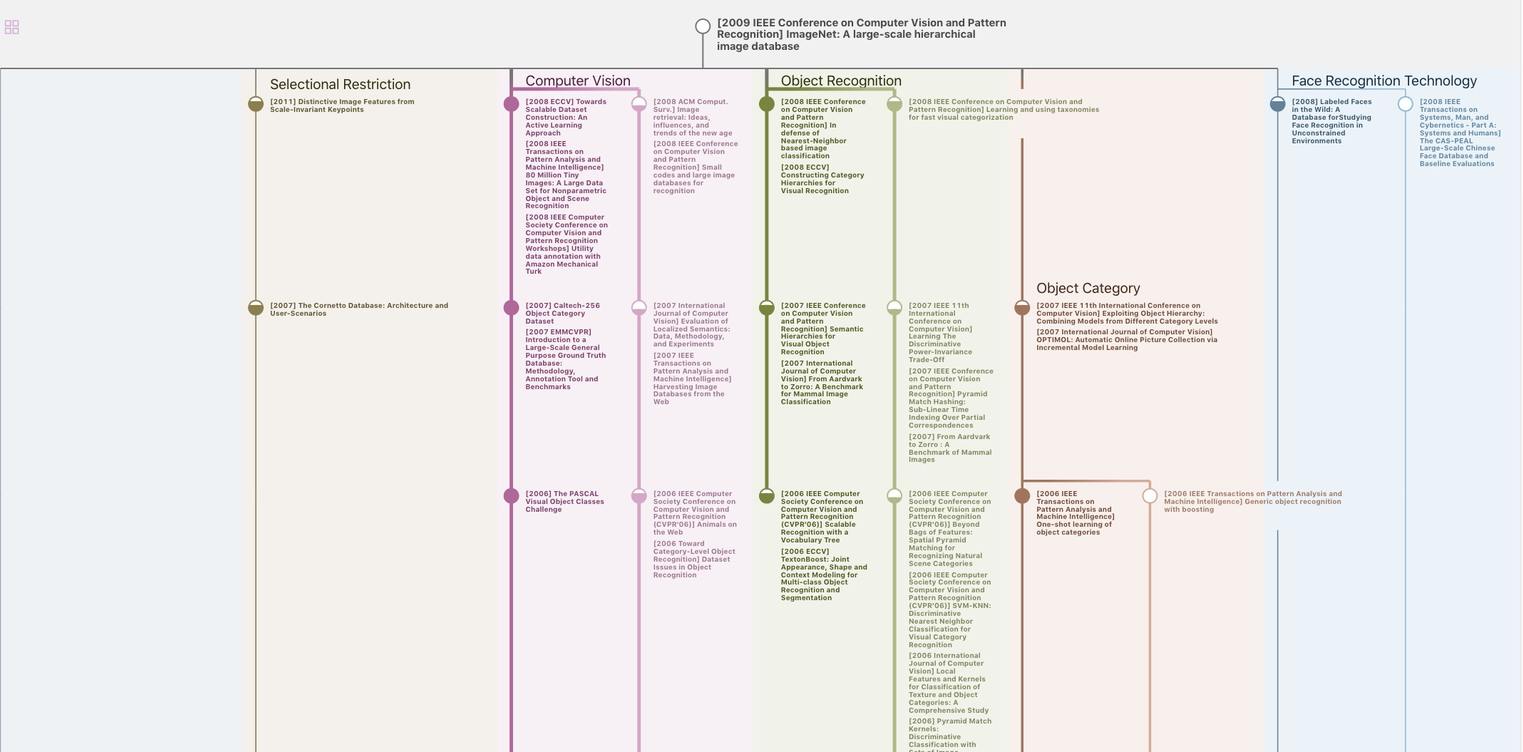
Generate MRT to find the research sequence of this paper
Chat Paper
Summary is being generated by the instructions you defined