Identification of active compounds as novel dipeptidyl peptidase-4 inhibitors through machine learning and structure-based molecular docking simulations
JOURNAL OF BIOMOLECULAR STRUCTURE & DYNAMICS(2023)
Abstract
The enzyme dipeptidyl peptidase 4 (DPP4) is a potential therapeutic target for type 2 diabetes (T2DM). Many synthetic anti-DPP4 medications are available to treat T2DM. The need for secure and efficient medicines has been unmet due to the adverse side effects of existing DPP4 medications. The present study implemented a combined approach to machine learning and structure-based virtual screening to identify DPP4 inhibitors. Two ML models were trained based on DPP4 IC50 datasets. The ML models random forest (RF) and multilayer perceptron (MLP) neural network showed good accuracy, with the area under the curve being 0.93 and 0.91, respectively. The natural compound library was screened through ML models, and 1% (217) of compounds were selected for further screening. Structure-based virtual screening was performed along with positive control sitagliptin to obtain more specific and selective leads for DPP4. Based on binding affinity, drug-likeness properties, and interaction with DPP4, Z-614 and Z-997 compounds showed high binding affinity and specificity in the catalytic pocket of DPP4. Finally, the stability conformation of the DPP4 enzyme complex was checked by a molecular dynamics (MD) simulation. The MD simulation showed that both compounds bind better in the catalytic pocket, but the Z-614 compound altered the DPP4 native conformation. Therefore, Z-614 showed a high deviation in the backbone. This combined approach (ML and structure-based) study reported that Z-997 binds most stably to DPP4 in their catalytic pocket with a binding free energy of -70.3 kJ/mol, suggesting its therapeutic potential as a treatment option for T2DM disease.Communicated by Ramaswamy H. Sarma
MoreTranslated text
Key words
Machine learning,type 2 diabetes mellitus,DPP4,MD simulation,natural compounds
AI Read Science
Must-Reading Tree
Example
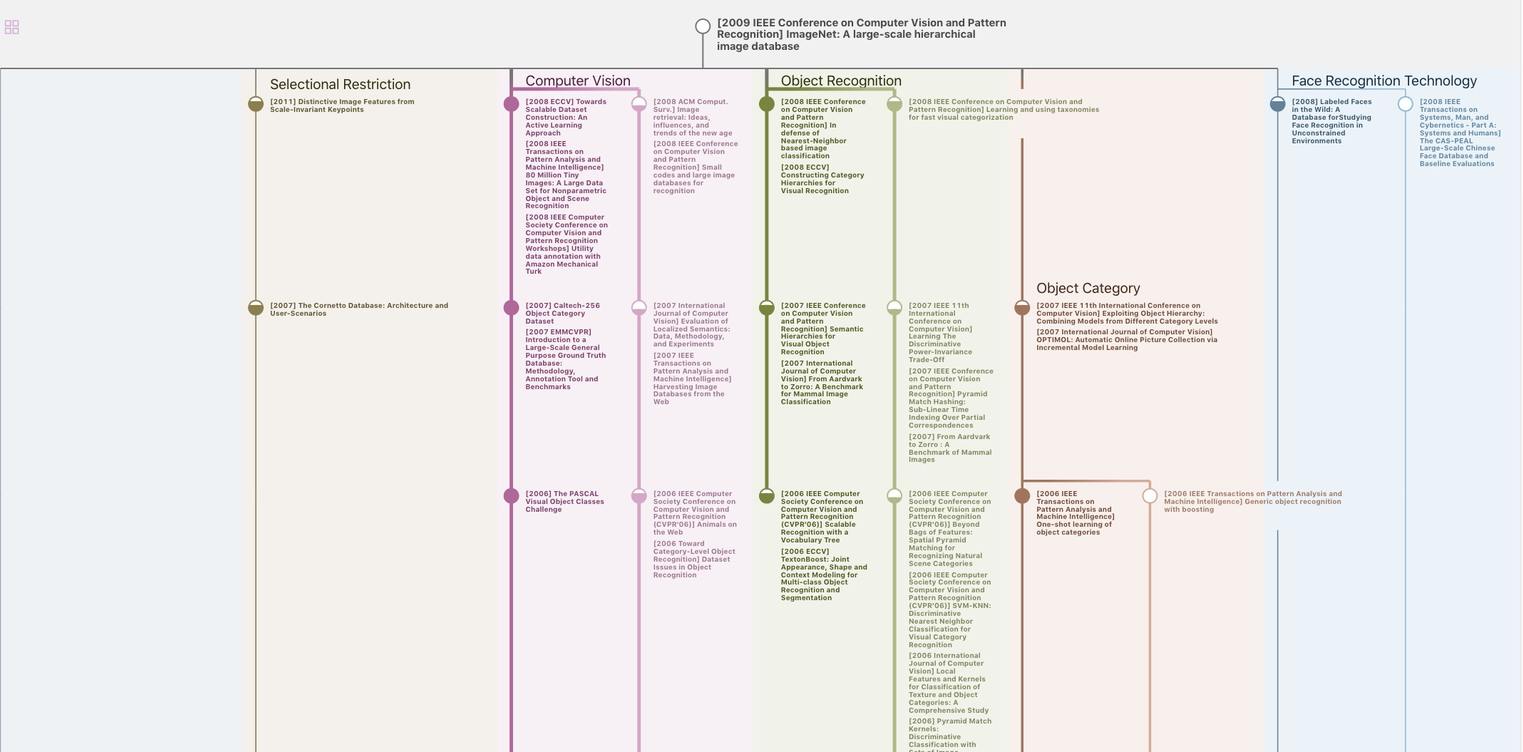
Generate MRT to find the research sequence of this paper
Chat Paper
Summary is being generated by the instructions you defined