Predicting the pair correlation functions of silicate and borosilicate glasses using machine learning
PHYSICAL CHEMISTRY CHEMICAL PHYSICS(2024)
摘要
Glasses offer a broad range of tunable thermophysical properties that are linked to their compositions. However, it is challenging to establish a universal composition-property relationship of glasses due to their enormous compositions and chemical space. Here, we address this problem and develop a metamodel of the composition-atomistic structure relationship of a class of glassy materials via a machine learning (ML) approach. Within this ML framework, an unsupervised deep learning technique, viz., a convolutional neural network (CNN) autoencoder, and a regression algorithm, viz. random forest (RF), are integrated into a fully automated pipeline to predict the spatial distribution of atoms in a glass. The RF regression model predicts the pair correlation function of a glass in a latent space. Subsequently, the decoder of the CNN converts the latent space representation to the actual pair correlation function of the given glass. The atomistic structures of silicate (SiO2) and sodium borosilicate (NBS) based glasses with varying compositions and dopants are collected from molecular dynamics (MD) simulations to establish and validate this ML pipeline. The model is found to predict the atom pair correlation functions for many unknown glasses very accurately. This method is very generic and can accelerate the design, discovery, and fundamental understanding of the composition-atomistic structure relationship of glasses and other materials. We report a machine learning method for predicting the atom pair correlation functions of a class of glassy materials.
更多查看译文
AI 理解论文
溯源树
样例
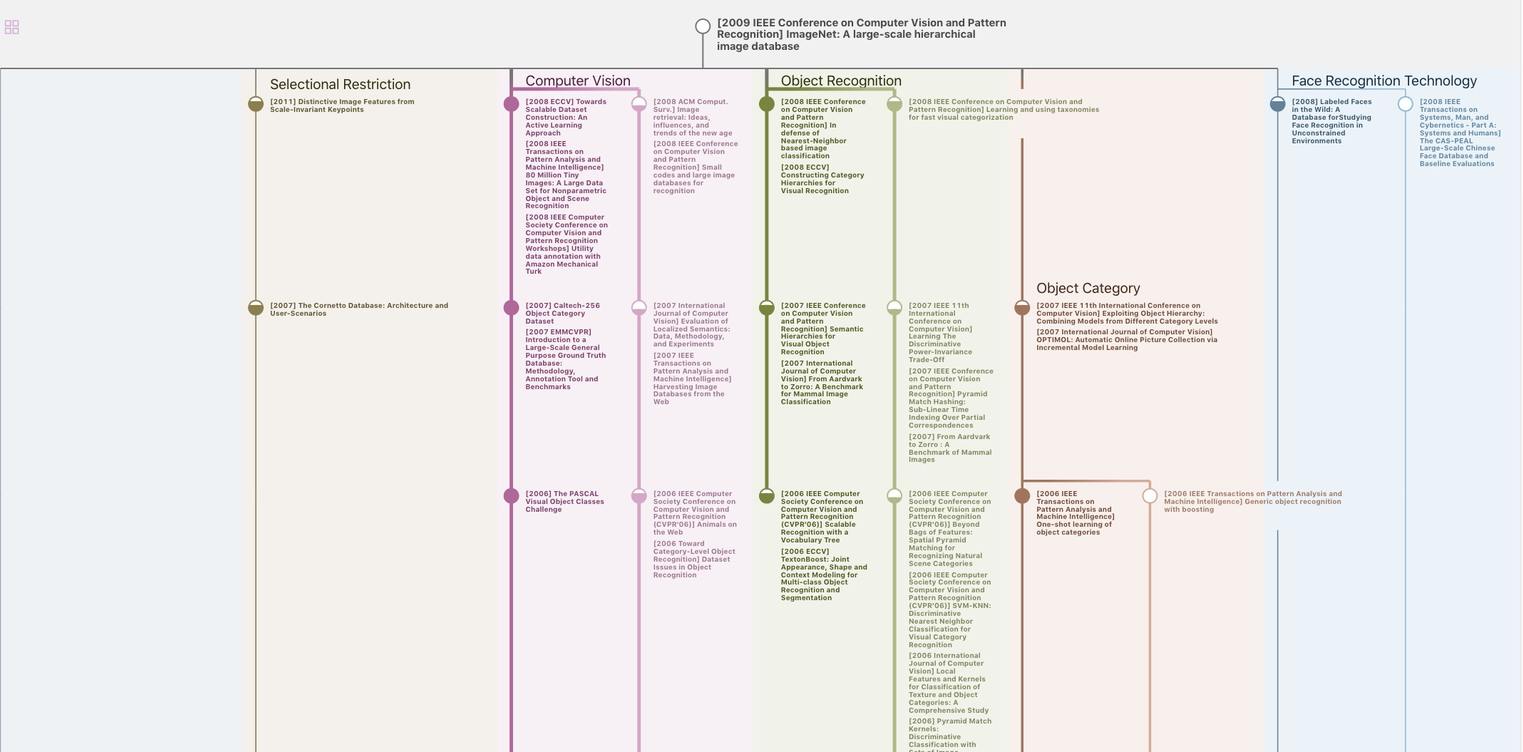
生成溯源树,研究论文发展脉络
Chat Paper
正在生成论文摘要