Efficient Multi-View K -Means for Image Clustering
IEEE TRANSACTIONS ON IMAGE PROCESSING(2024)
摘要
Nowadays, data in the real world often comes from multiple sources, but most existing multi-view K-Means perform poorly on linearly non-separable data and require initializing the cluster centers and calculating the mean, which causes the results to be unstable and sensitive to outliers. This paper proposes an efficient multi-view K -Means to solve the above-mentioned issues. Specifically, our model avoids the initialization and computation of clusters centroid of data. Additionally, our model use the Butterworth filters function to transform the adjacency matrix into a distance matrix, which makes the model is capable of handling linearly inseparable data and insensitive to outliers. To exploit the consistency and complementarity across multiple views, our model constructs a third tensor composed of discrete index matrices of different views and minimizes the tensor's rank by tensor Schatten p-norm. Experiments on two artificial datasets verify the superiority of our model on linearly inseparable data, and experiments on several benchmark datasets illustrate the performance.
更多查看译文
关键词
Multi-view clustering,unsupervised learning,tensor low-rank constraint
AI 理解论文
溯源树
样例
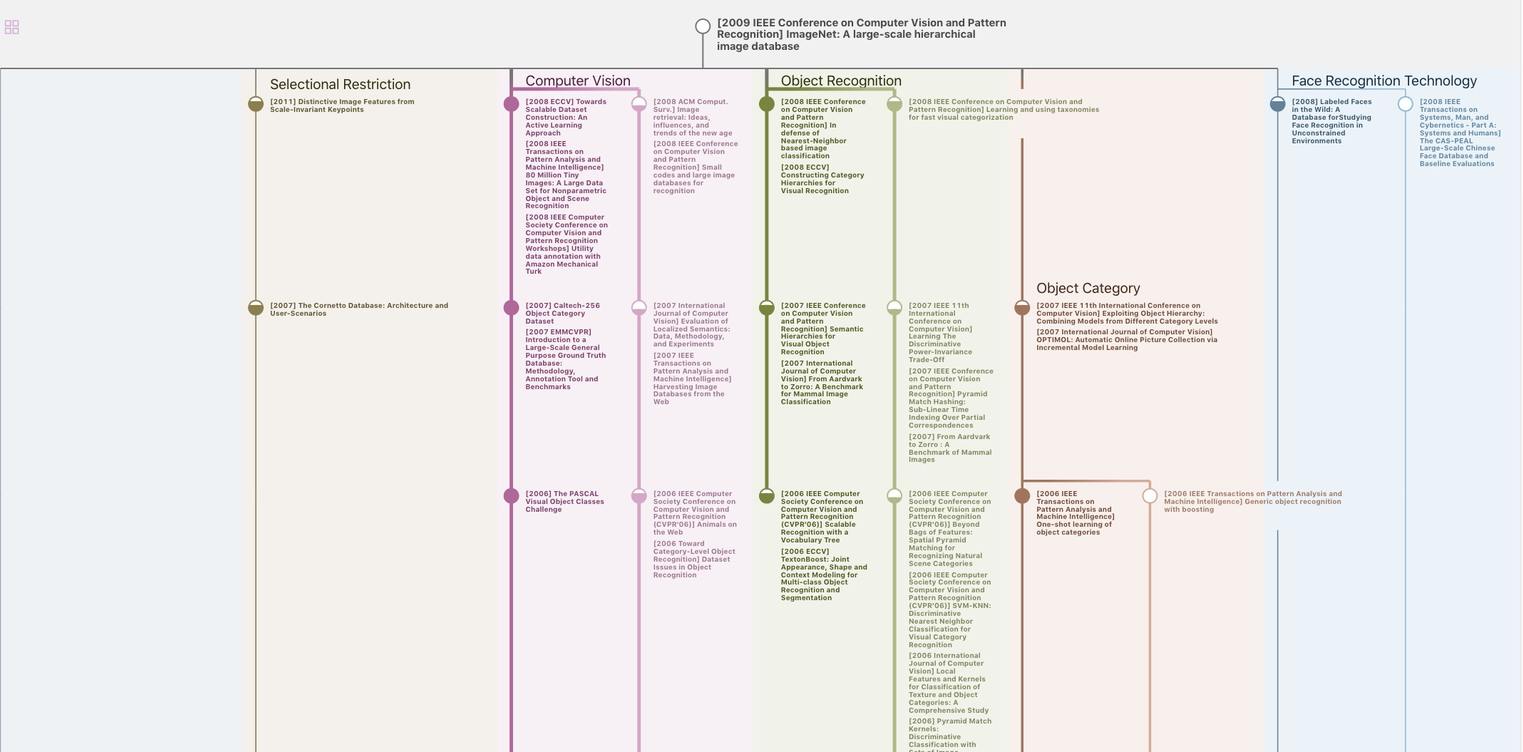
生成溯源树,研究论文发展脉络
Chat Paper
正在生成论文摘要