ADFCNN: Attention-Based Dual-Scale Fusion Convolutional Neural Network for Motor Imagery Brain-Computer Interface
IEEE TRANSACTIONS ON NEURAL SYSTEMS AND REHABILITATION ENGINEERING(2024)
Abstract
Convolutional neural networks (CNNs) have been successfully applied to motor imagery (MI)-based brain-computer interface (BCI). Nevertheless, single-scale CNN fail to extract abundant information over a wide spectrum from EEG signals, while typical multi-scale CNNs cannot effectively fuse information from different scales with concatenation-based methods. To overcome these challenges, we propose a new scheme equipped with attention-based dual-scale fusion convolutional neural network (ADFCNN), which jointly extracts and fuses EEG spectral and spatial information at different scales. This scheme also provides novel insight through self-attention for effective information fusion from different scales. Specifically, temporal convolutions with two different kernel sizes identify EEG mu and beta rhythms, while spatial convolutions at two different scales generate global and detailed spatial information, respectively, and the self-attention mechanism performs feature fusion based on the internal similarity of the concatenated features extracted by the dual-scale CNN. The proposed scheme achieves the superior performance compared with state-of-the-art methods in subject-specific motor imagery recognition on BCI Competition IV dataset 2a, 2b and OpenBMI dataset, with the cross-session average classification accuracies of 79.39% and significant improvements of 9.14% on BCI-IV2a, 87.81% and 7.66% on BCI-IV2b, 65.26% and 7.2% on OpenBMI dataset, and the within-session average classification accuracies of 86.87% and significant improvements of 10.89% on BCI-IV2a, 87.26% and 8.07% on BCI-IV2b, 84.29% and 5.17% on OpenBMI dataset, respectively. What is more, ablation experiments are conducted to investigate the mechanism and demonstrate the effectiveness of the dual-scale joint temporal-spatial CNN and self-attention modules. Visualization is also used to reveal the learning process and feature distribution of the model.
MoreTranslated text
Key words
Convolutional neural networks (CNNs),motor imagery (MI),brain-computer interface (BCI),self-attention mechanism
AI Read Science
Must-Reading Tree
Example
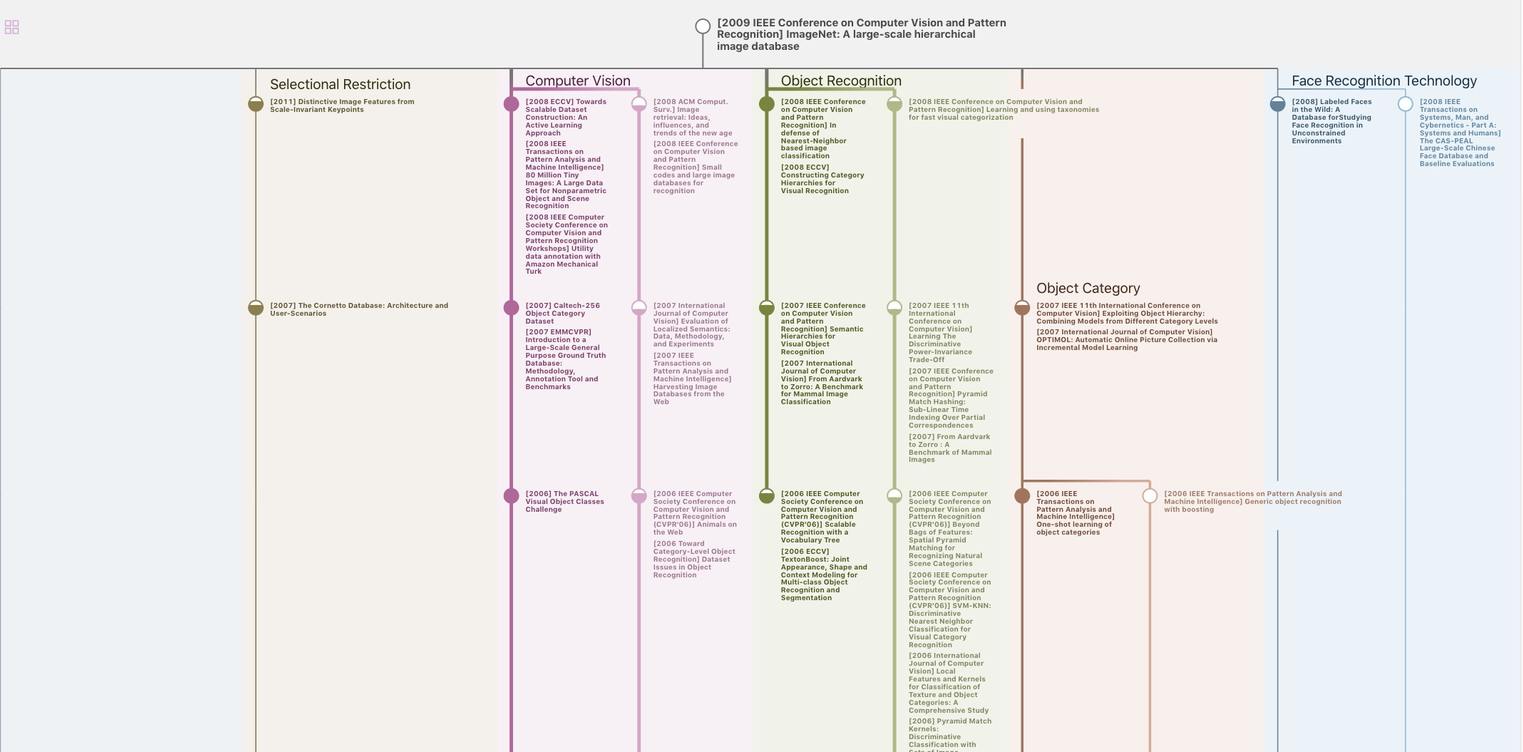
Generate MRT to find the research sequence of this paper
Chat Paper
Summary is being generated by the instructions you defined