Revisiting Confidence Estimation: Towards Reliable Failure Prediction
IEEE TRANSACTIONS ON PATTERN ANALYSIS AND MACHINE INTELLIGENCE(2024)
摘要
Reliable confidence estimation is a challenging yet fundamental requirement in many risk-sensitive applications. However, modern deep neural networks are often overconfident for their incorrect predictions, i.e., misclassified samples from known classes, and out-of-distribution (OOD) samples from unknown classes. In recent years, many confidence calibration and OOD detection methods have been developed. In this paper, we find a general, widely existing but actually-neglected phenomenon that most confidence estimation methods are harmful for detecting misclassification errors. We investigate this problem and reveal that popular calibration and OOD detection methods often lead to worse confidence separation between correctly classified and misclassified examples, making it difficult to decide whether to trust a prediction or not. Finally, we propose to enlarge the confidence gap by finding flat minima, which yields state-of-the-art failure prediction performance under various settings including balanced, long-tailed, and covariate-shift classification scenarios. Our study not only provides a strong baseline for reliable confidence estimation but also acts as a bridge between understanding calibration, OOD detection, and failure prediction.
更多查看译文
关键词
Confidence estimation,uncertainty quantification,failure prediction,misclassification detection,selective classification,out-of-distribution detection,confidence calibration,model reliability,trustworthy,flat minima
AI 理解论文
溯源树
样例
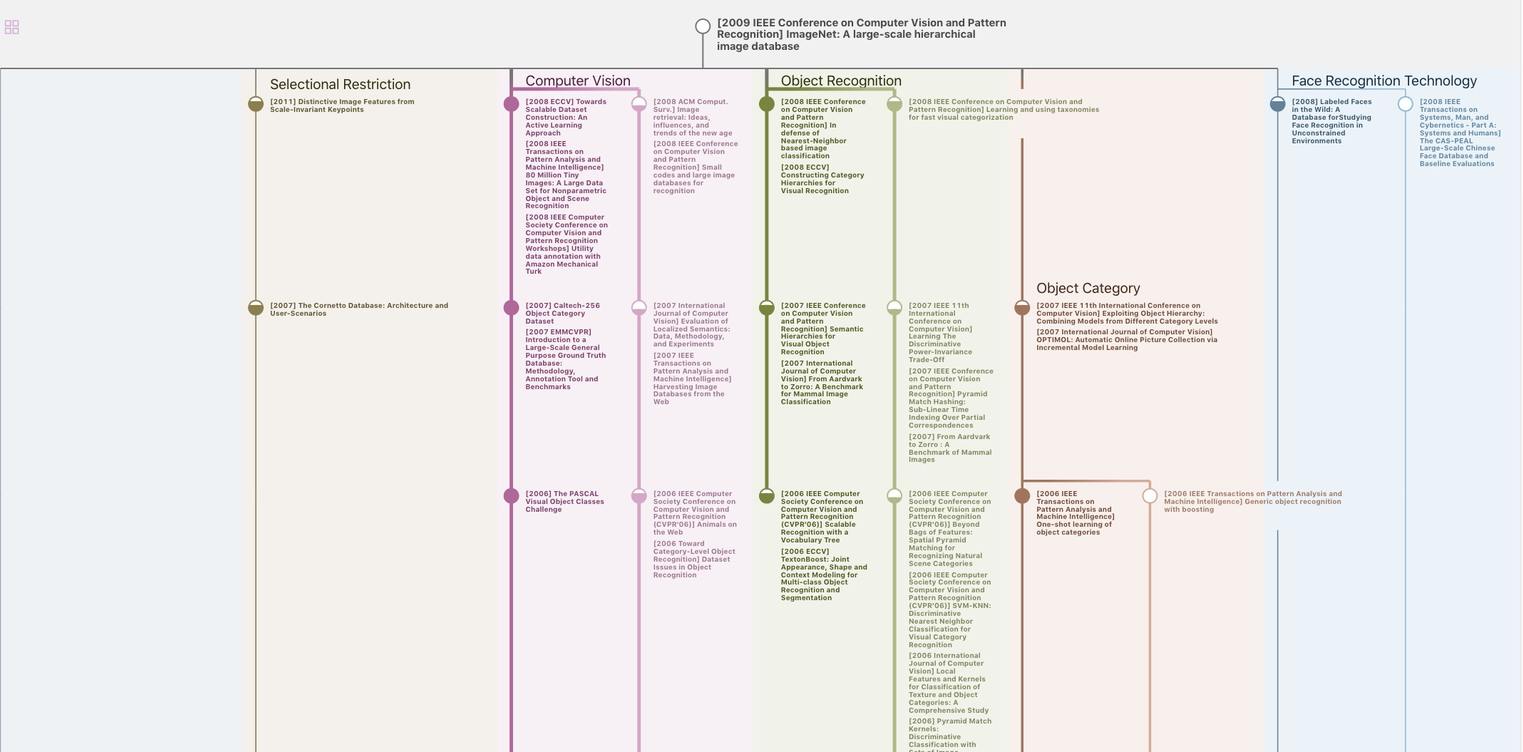
生成溯源树,研究论文发展脉络
Chat Paper
正在生成论文摘要