A comprehensive overview of graph neural network-based approaches to clustering for spatial transcriptomics
COMPUTATIONAL AND STRUCTURAL BIOTECHNOLOGY JOURNAL(2024)
摘要
Spatial transcriptomics technologies enable researchers to accurately quantify and localize messenger ribonucleic acid (mRNA) transcripts at a high resolution while preserving their spatial context. The identification of spatial domains, or the task of spatial clustering, plays a crucial role in investigating data on spatial transcriptomes. One promising approach for classifying spatial domains involves the use of graph neural networks (GNNs) by leveraging gene expressions, spatial locations, and histological images. This study provided a comprehensive overview of the most recent GNN-based methods of spatial clustering methods for the analysis of data on spatial transcriptomics. We extensively evaluated the performance of current methods on prevalent datasets of spatial transcriptomics by considering their accuracy of clustering, robustness, data stabilization, relevant requirements, computational efficiency, and memory use. To this end, we explored 60 clustering scenarios by extending the essential frameworks of spatial clustering for the selection of the GNNs, algorithms of downstream clustering, principal component analysis (PCA)-based reduction, and refined methods of correction. We comparatively analyzed the performance of the methods in terms of spatial clustering to identify their limitations and outline future directions of research in the field. Our survey yielded novel insights, and provided motivation for further investigating spatial transcriptomics.
更多查看译文
关键词
Spatial transcriptome,Cell type identification,Graph-based deep learning,Graph neural networks,Survey
AI 理解论文
溯源树
样例
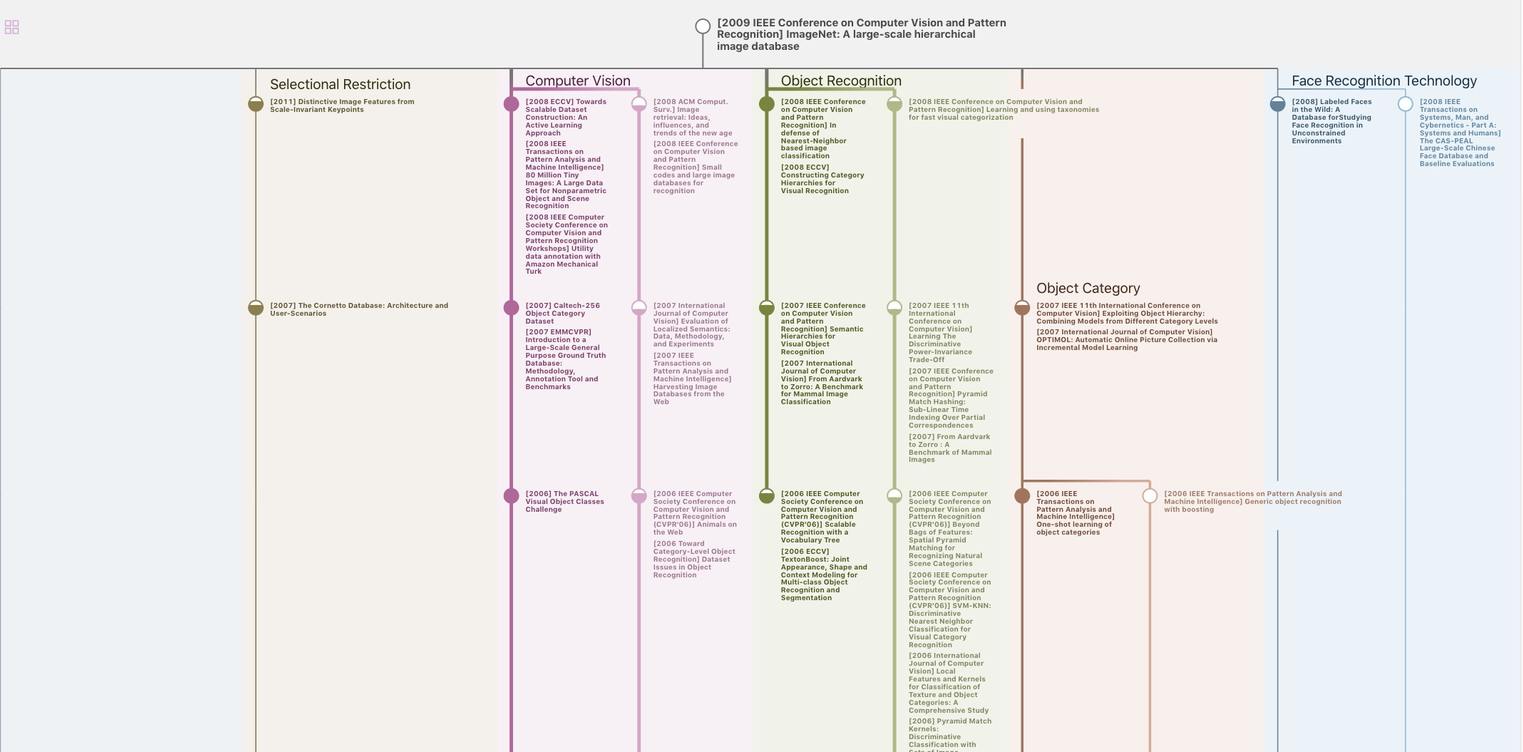
生成溯源树,研究论文发展脉络
Chat Paper
正在生成论文摘要