Robust Communicative Multi-Agent Reinforcement Learning with Active Defense
AAAI 2024(2024)
摘要
Communication in multi-agent reinforcement learning (MARL) has been proven to effectively promote cooperation among agents recently. Since communication in real-world scenarios is vulnerable to noises and adversarial attacks, it is crucial to develop robust communicative MARL technique. However, existing research in this domain has predominantly focused on passive defense strategies, where agents receive all messages equally, making it hard to balance performance and robustness. We propose an active defense strategy, where agents automatically reduce the impact of potentially harmful messages on the final decision. There are two challenges to implement this strategy, that are defining unreliable messages and adjusting the unreliable messages' impact on the final decision properly. To address them, we design an Active Defense Multi-Agent Communication framework (ADMAC), which estimates the reliability of received messages and adjusts their impact on the final decision accordingly with the help of a decomposable decision structure. The superiority of ADMAC over existing methods is validated by experiments in three communication-critical tasks under four types of attacks.
更多查看译文
关键词
MAS: Agent Communication,MAS: Adversarial Agents
AI 理解论文
溯源树
样例
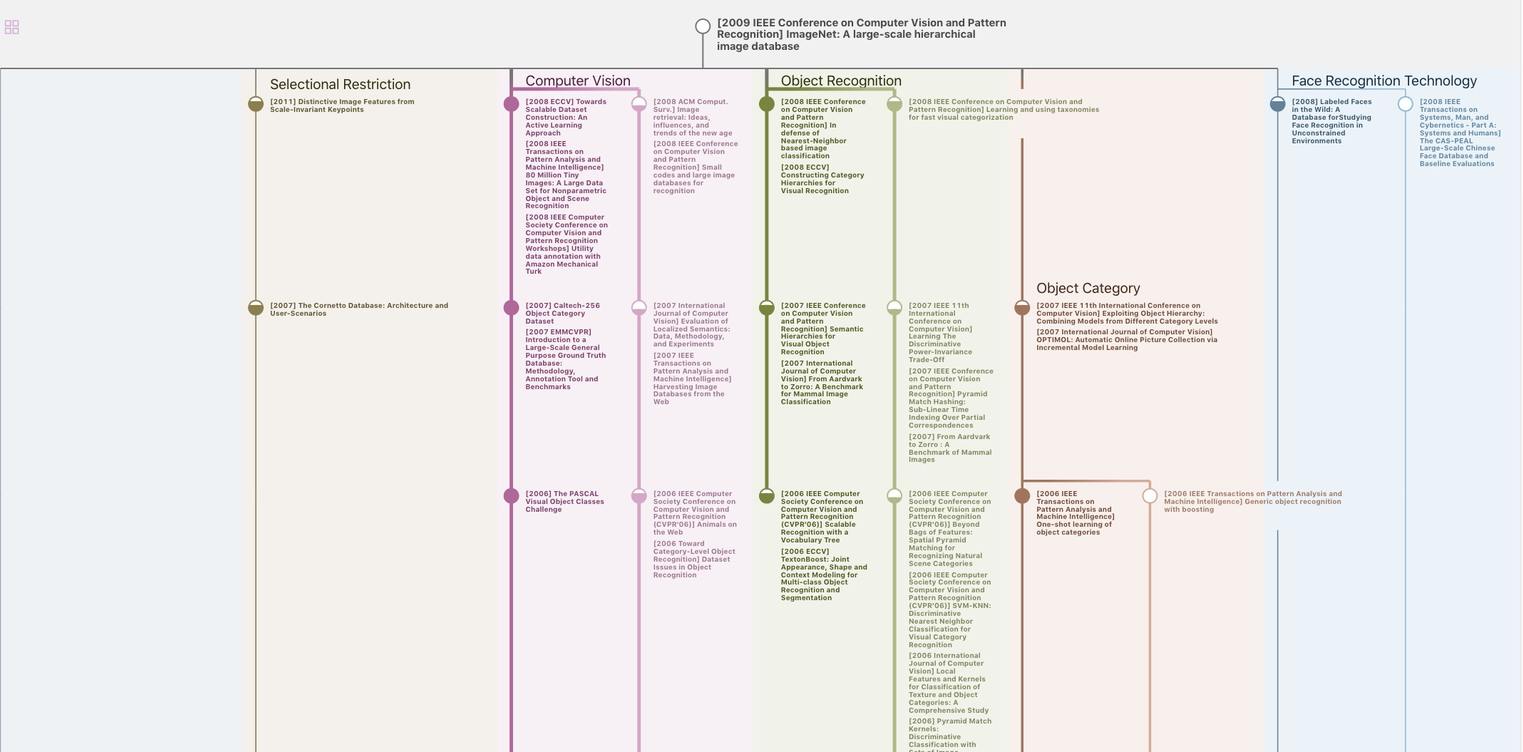
生成溯源树,研究论文发展脉络
Chat Paper
正在生成论文摘要