Anomaly detection for automated inspection of power line insulators
CoRR(2023)
摘要
Inspection of insulators is important to ensure reliable operation of the
power system. Deep learning has recently been explored to automate the
inspection process by leveraging aerial images captured by drones along with
powerful object detection models. However, a purely object detection-based
approach exhibits class imbalance-induced poor detection accuracy for faulty
insulators, especially for incipient faults. In order to address this issue in
a data-efficient manner, this article proposes a two-stage approach that
leverages object detection in conjunction with anomaly detection to reliably
detect faults in insulators. The article adopts an explainable deep neural
network-based one-class classifier for anomaly detection, that reduces the
reliance on plentifully available images of faulty insulators, that might be
difficult to obtain in real-life applications. The anomaly detection model is
trained with two datasets -- representing data abundant and data scarce
scenarios -- in unsupervised and semi-supervised manner. The results suggest
that including as few as six real anomalies in the training dataset
significantly improves the performance of the model, and enables reliable
detection of rarely occurring faults in insulators. An analysis of the
explanations provided by the anomaly detection model reveals that the model is
able to accurately identify faulty regions on the insulator disks, while also
exhibiting some false predictions.
更多查看译文
AI 理解论文
溯源树
样例
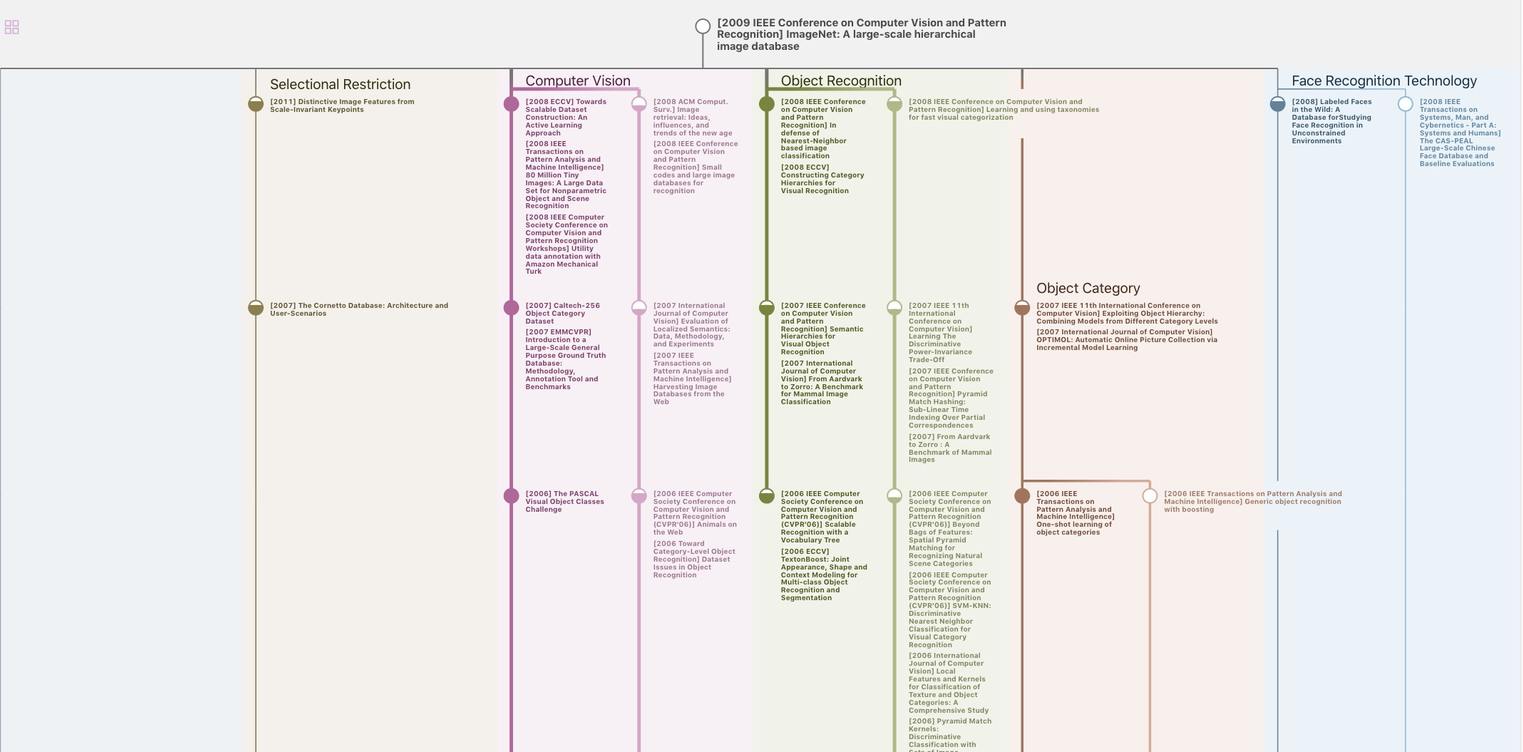
生成溯源树,研究论文发展脉络
Chat Paper
正在生成论文摘要