Tuning for fluidity using fluctuations in biological tissue models
arxiv(2023)
摘要
How do biological systems tune emergent properties at the scale of tissues? One class of such emergent behaviors, important to biological functions such as body-axis elongation, involves rigidity transitions, in which a tissue changes from a fluid-like state to a solid-like state or vice versa. Here, we explore the idea that tissues might tune ``learning degrees of freedom" to affect this emergent behavior. We study tissue fluidity in the 2D vertex model, using the vertex model energy as a learning cost function and the cell stiffnesses, target shapes, and target areas as sets of learning degrees of freedom that can be varied to minimize the energy. We show that the rigidity transition is unaffected when cell stiffnesses are treated as learning degrees of freedom. When preferred perimeters or areas are treated as learning degrees of freedom, however, energy minimization introduces spatial correlations in target cell shapes or areas that shift the rigidity transition. There is an optimal heterogeneity of target cell shapes or areas to enable learning. These observations suggest that biological tissues can learn tissue-scale behaviors by tuning their individual cell properties.
更多查看译文
AI 理解论文
溯源树
样例
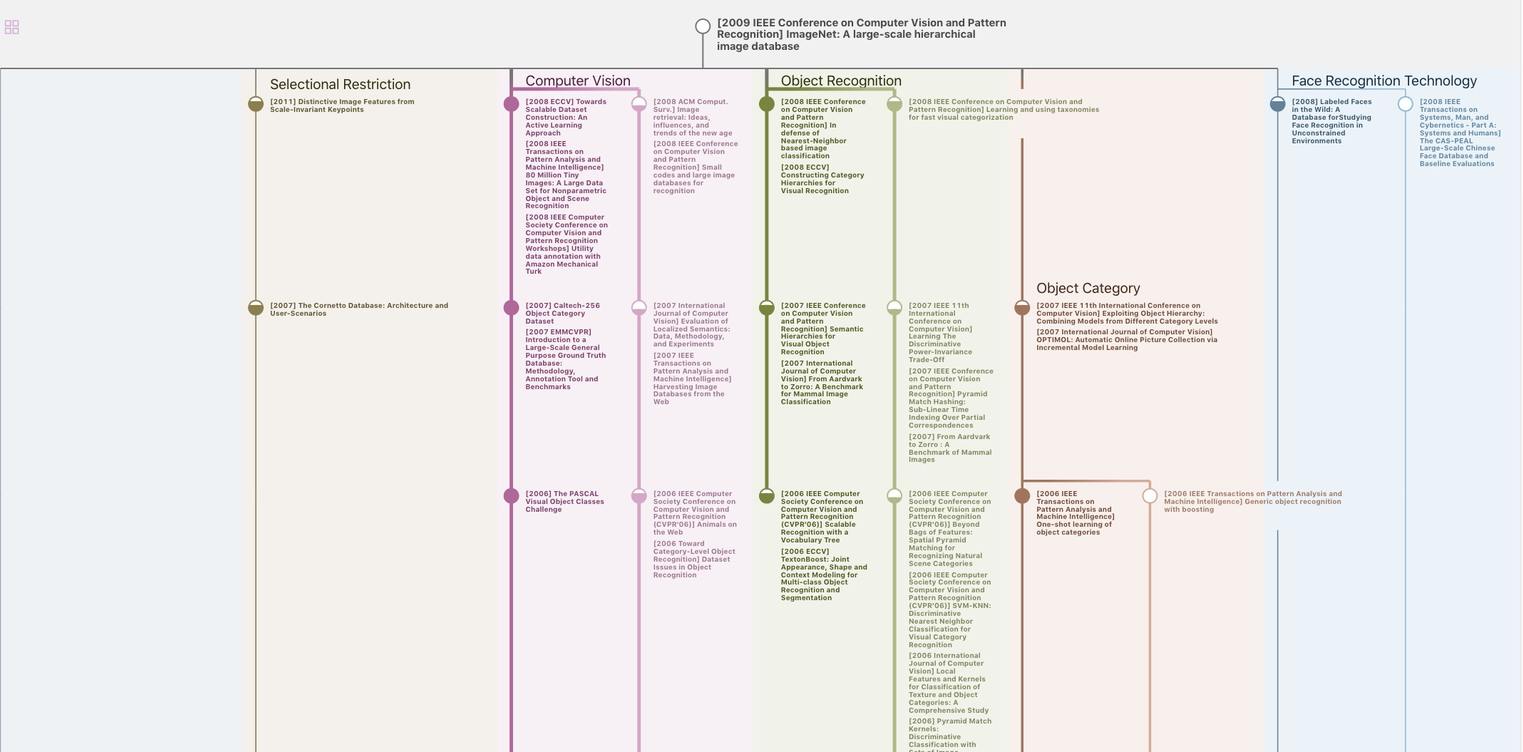
生成溯源树,研究论文发展脉络
Chat Paper
正在生成论文摘要