Aligning Human Intent from Imperfect Demonstrations with Confidence-based Inverse soft-Q Learning
IEEE Robotics and Automation Letters(2023)
摘要
Imitation learning attracts much attention for its ability to allow robots to
quickly learn human manipulation skills through demonstrations. However, in the
real world, human demonstrations often exhibit random behavior that is not
intended by humans. Collecting high-quality human datasets is both challenging
and expensive. Consequently, robots need to have the ability to learn
behavioral policies that align with human intent from imperfect demonstrations.
Previous work uses confidence scores to extract useful information from
imperfect demonstrations, which relies on access to ground truth rewards or
active human supervision. In this paper, we propose a transition-based method
to obtain fine-grained confidence scores for data without the above efforts,
which can increase the success rate of the baseline algorithm by 40.3% on
average. We develop a generalized confidence-based imitation learning framework
for guiding policy learning, called Confidence-based Inverse soft-Q Learning
(CIQL), as shown in Fig.1. Based on this, we analyze two ways of processing
noise and find that penalization is more aligned with human intent than
filtering.
更多查看译文
关键词
Imitation learning,learning from demonstration,manipulation planning
AI 理解论文
溯源树
样例
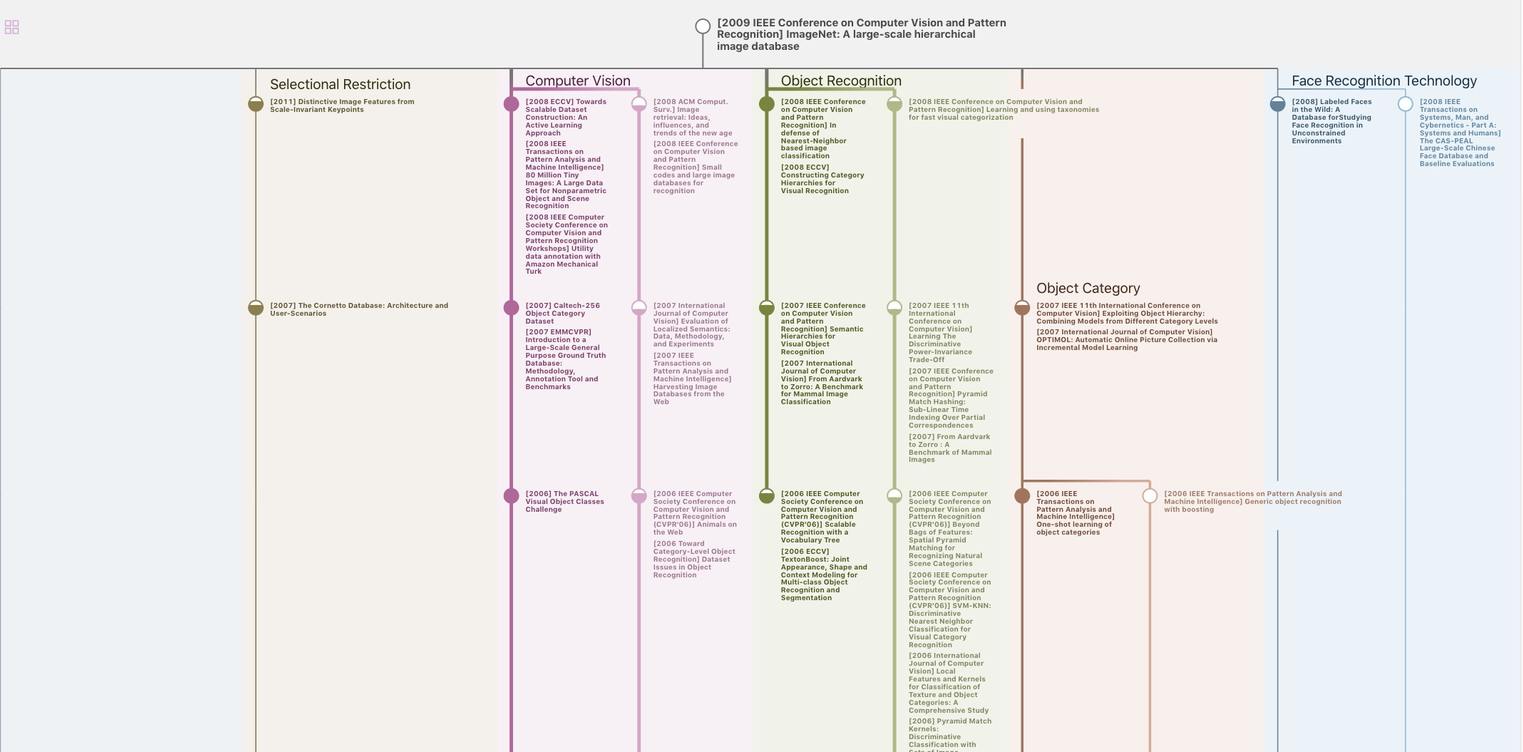
生成溯源树,研究论文发展脉络
Chat Paper
正在生成论文摘要