Predict and Interpret Health Risk using EHR through Typical Patients
CoRR(2023)
摘要
Predicting health risks from electronic health records (EHR) is a topic of recent interest. Deep learning models have achieved success by modeling temporal and feature interaction. However, these methods learn insufficient representations and lead to poor performance when it comes to patients with few visits or sparse records. Inspired by the fact that doctors may compare the patient with typical patients and make decisions from similar cases, we propose a Progressive Prototypical Network (PPN) to select typical patients as prototypes and utilize their information to enhance the representation of the given patient. In particular, a progressive prototype memory and two prototype separation losses are proposed to update prototypes. Besides, a novel integration is introduced for better fusing information from patients and prototypes. Experiments on three real-world datasets demonstrate that our model brings improvement on all metrics. To make our results better understood by physicians, we developed an application at http://ppn.ai-care.top. Our code is released at https://github.com/yzhHoward/PPN.
更多查看译文
关键词
Electronic health record,healthcare analysis,prototype learning,interpretability
AI 理解论文
溯源树
样例
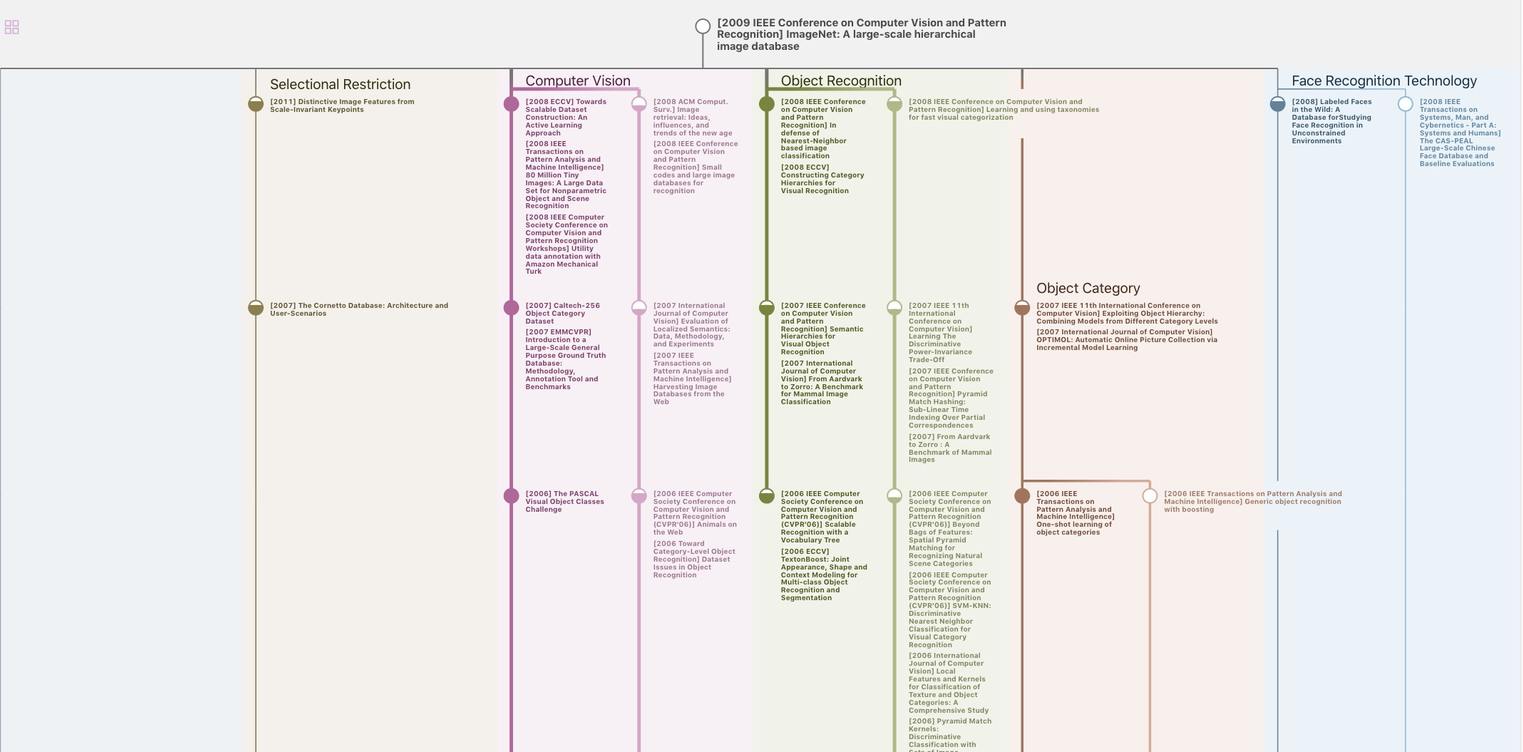
生成溯源树,研究论文发展脉络
Chat Paper
正在生成论文摘要