SESOMP: A Scalable and Energy-Efficient Self-Organizing Map Processor with Computing-In-Memory and Dead Neuron Pruning.
2023 IEEE Asian Solid-State Circuits Conference (A-SSCC)(2023)
摘要
Self-organizing map (SOM), as a versatile artificial neural network, has been used in extensive fields, such as data clustering, pattern recognition, image quantization, etc. Massive parallel vector computations in the SOM necessitate specific hardware accelerators [1]–[5] for real-time data processing. However, the existing endeavors face the following limitations (Fig. 1(top)): 1) high power consumption caused by frequent memory access for massive vector processing, 2) energy waste from redundant computations in seldomly updated neurons (called “dead neurons”), and 3) mapping difficulty for various network sizes due to the unscalable architecture. To overcome the above limitations, this paper proposes a Scalable Energy-efficient SOM Processor (SESOMP) (Fig. 1(bottom)) with three key features: 1) computing-in-memory (CIM) macros for reducing memory access during SOM operation, 2) adaptive pruning to avoid redundant computation caused by dead neurons, and 3) a scalable architecture to support various network sizes.
更多查看译文
AI 理解论文
溯源树
样例
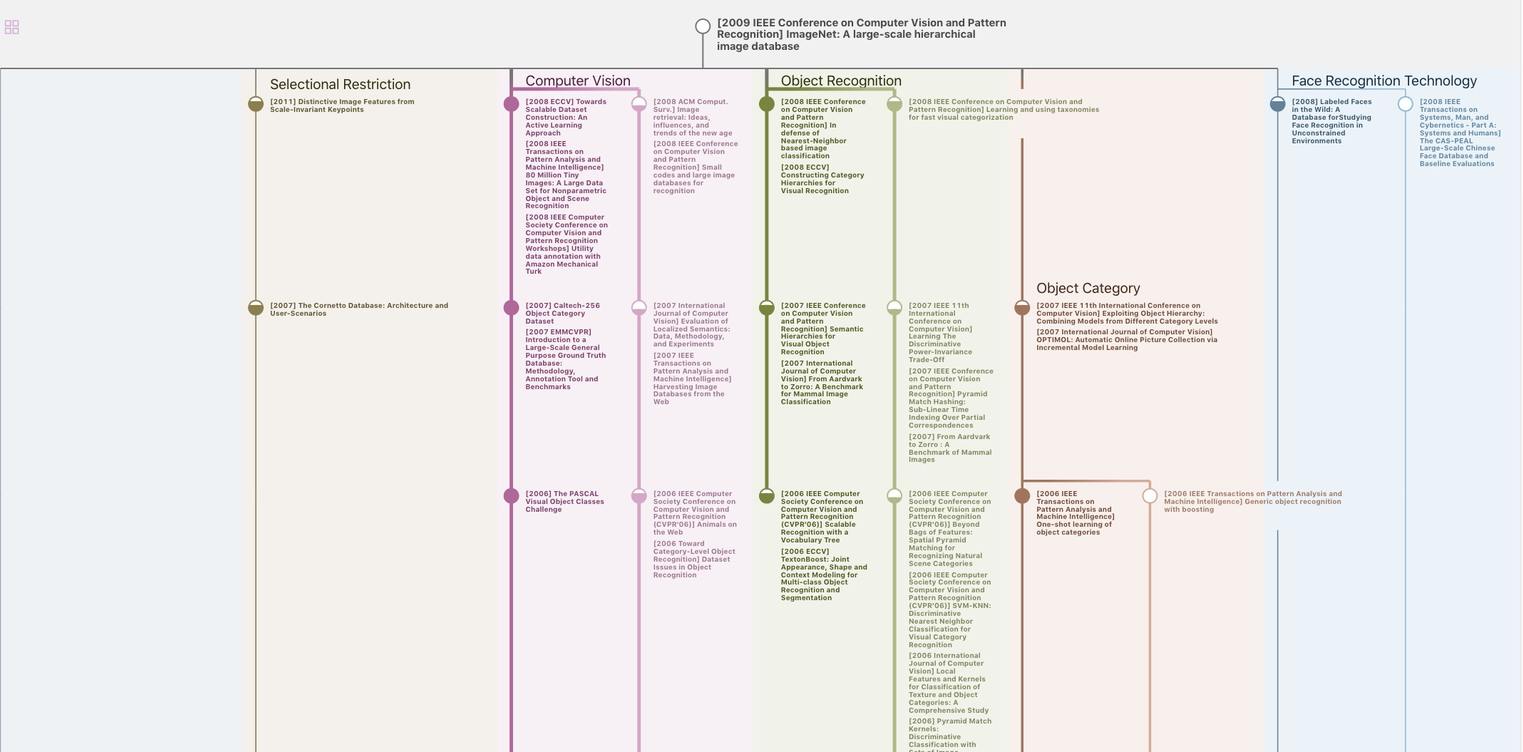
生成溯源树,研究论文发展脉络
Chat Paper
正在生成论文摘要