Multi-Type Anomaly Detection Using One Deep Neural Model for QAR Data
2023 IEEE 5th International Conference on Civil Aviation Safety and Information Technology (ICCASIT)(2023)
摘要
QAR data serves as a critical data foundation for flight quality assessment, accident investigation and maintenance management. However, raw QAR data contain various types of anomalies caused by sensor faults or abnormal flight operations. Therefore, anomaly detection from QAR data plays important roles in mining the cause of abnormality and providing a guarantee for flight safety. Although, there are several related works focusing on anomaly detection for sensor data of different industries, they cannot get the same high performance on different type of anomalies. Further more, due to the peculiar features of QAR data, the existing methods cannot performs as well as on common sensor data. Therefore, there is a need to propose a generic anomaly detection algorithm for QAR data to detect different type of anomalies efficiently in one time. In this work, we propose a general anomaly detection method, which can effectively capture complex spatial-temporal information in QAR data. The proposed method can be applied to detect multiple types of anomalies using one deep neural model. Experiments are conducted on different types of anomaly datasets. The experimental results show that the proposed new method can effectively detect multiple types of anomalies in QAR data with higher performance compared with existing methods.
更多查看译文
关键词
anomaly detection,QAR data,Multivariate time series,Spatial-temporal graph neural network
AI 理解论文
溯源树
样例
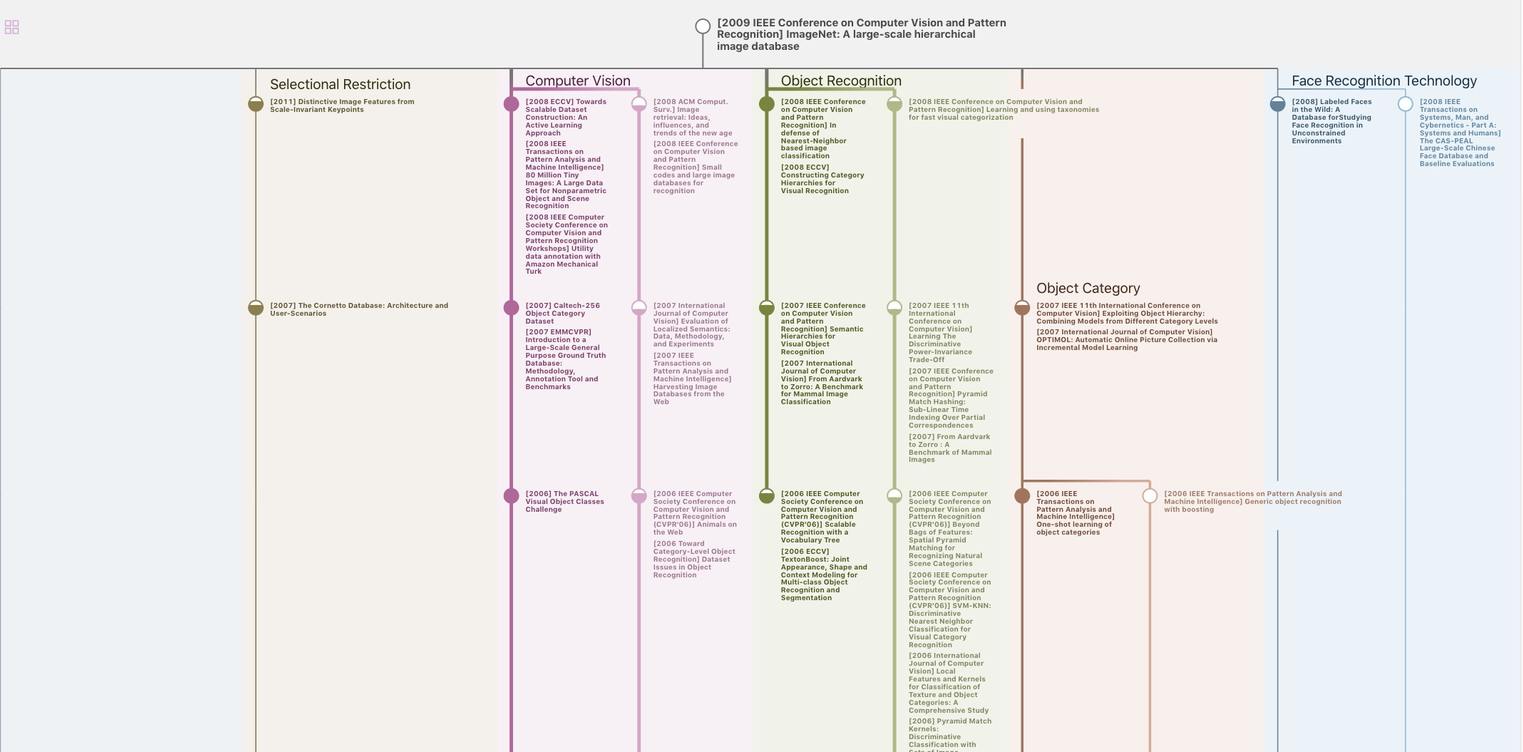
生成溯源树,研究论文发展脉络
Chat Paper
正在生成论文摘要