Gradient-aware learning for joint biases: Label noise and class imbalance
NEURAL NETWORKS(2024)
摘要
Data biases such as class imbalance and label noise always exist in large-scale datasets in real-world. These problems bring huge challenges to deep learning methods. Some previous works adopted loss re-weighting, sample re-weighting, or data-dependent regularization to mitigate the influence of these training biases. But these methods usually pay more attention to class imbalance problem when both the class imbalance and label noise exist in training set simultaneously. These methods may overfit noisy labels, which leads to a great degradation in performance. In this paper, we propose a gradient-aware learning method for the combination of the two biases. During the training process, we update only a part of crucial parameters regularly and rectify the update direction of the rest redundant parameters. This update rule is conducted both in the encoder and classifier of the deep network to decouple label noise and class imbalance implicitly. The experimental results verify the effectiveness of the proposed method on synthetic and real-world data biases.
更多查看译文
关键词
Label noise,Class imbalance,Gradient-aware,Meta-train
AI 理解论文
溯源树
样例
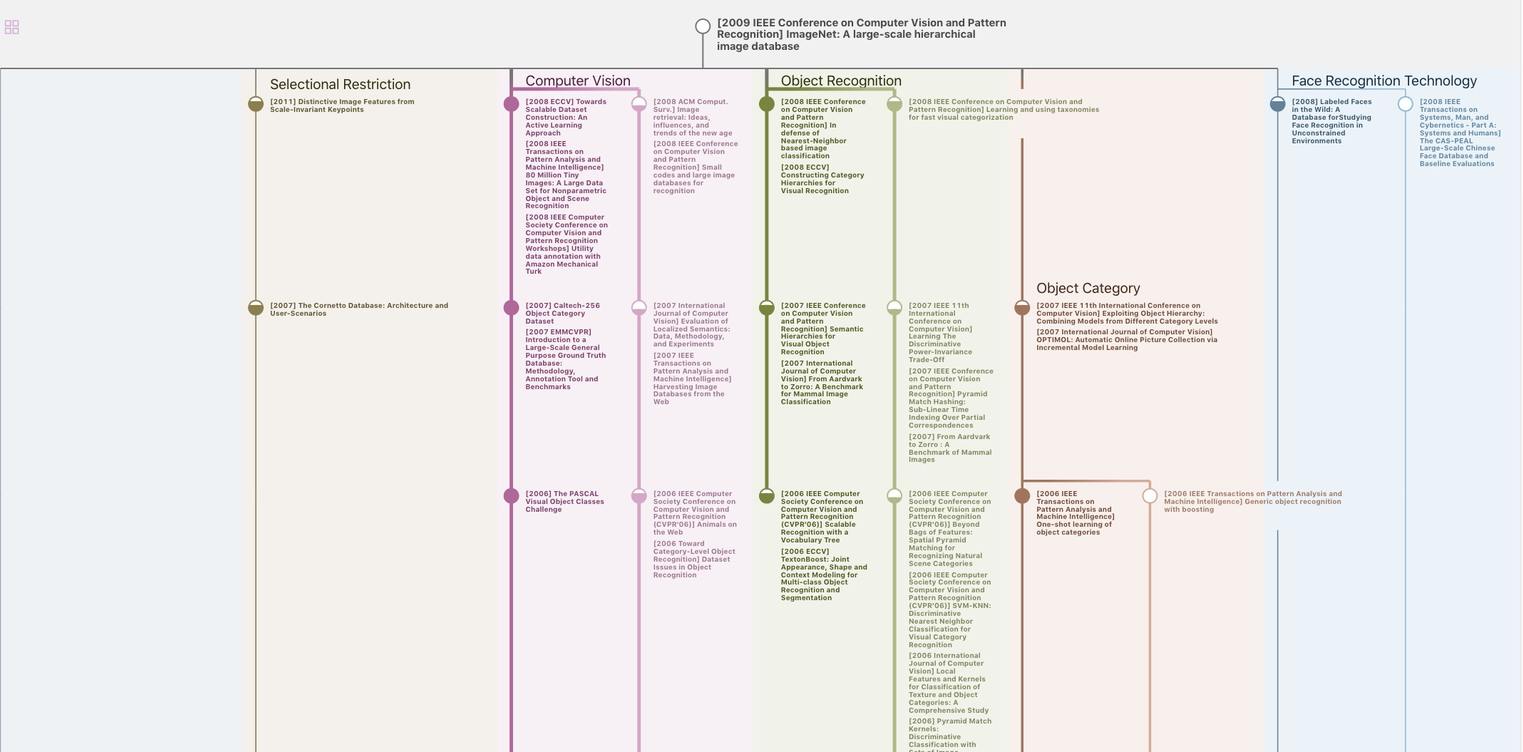
生成溯源树,研究论文发展脉络
Chat Paper
正在生成论文摘要