SGAE-MDA: Exploring the MiRNA-disease associations in herbal medicines based on semi-supervised graph autoencoder
METHODS(2024)
摘要
Research indicates that miRNAs present in herbal medicines are crucial for identifying disease markers, advancing gene therapy, facilitating drug delivery, and so on. These miRNAs maintain stability in the extracellular environment, making them viable tools for disease diagnosis. They can withstand the digestive processes in the gastrointestinal tract, positioning them as potential carriers for specific oral drug delivery. By engineering plants to generate effective, non-toxic miRNA interference sequences, it's possible to broaden their applicability, including the treatment of diseases such as hepatitis C. Consequently, delving into the miRNA-disease associations (MDAs) within herbal medicines holds immense promise for diagnosing and addressing miRNA-related diseases. In our research, we propose the SGAE-MDA model, which harnesses the strengths of a graph autoencoder (GAE) combined with a semi-supervised approach to uncover potential MDAs in herbal medicines more effectively. Leveraging the GAE framework, the SGAE-MDA model exactly integrates the inherent feature vectors of miRNAs and disease nodes with the regulatory data in the miRNA-disease network. Additionally, the proposed semi-supervised learning approach randomly hides the partial structure of the miRNA-disease network, subsequently reconstructing them within the GAE framework. This technique effectively minimizes network noise interference. Through comparison against other leading deep learning models, the results consistently highlighted the superior performance of the proposed SGAE-MDA model. Our code and dataset can be available at: https://github .com /22n9n23 /SGAE-MDA.
更多查看译文
关键词
Herbal medicines,MiRNA-disease associations (MDAs),Graph autoencoder,Semi-supervised approach,Network noise
AI 理解论文
溯源树
样例
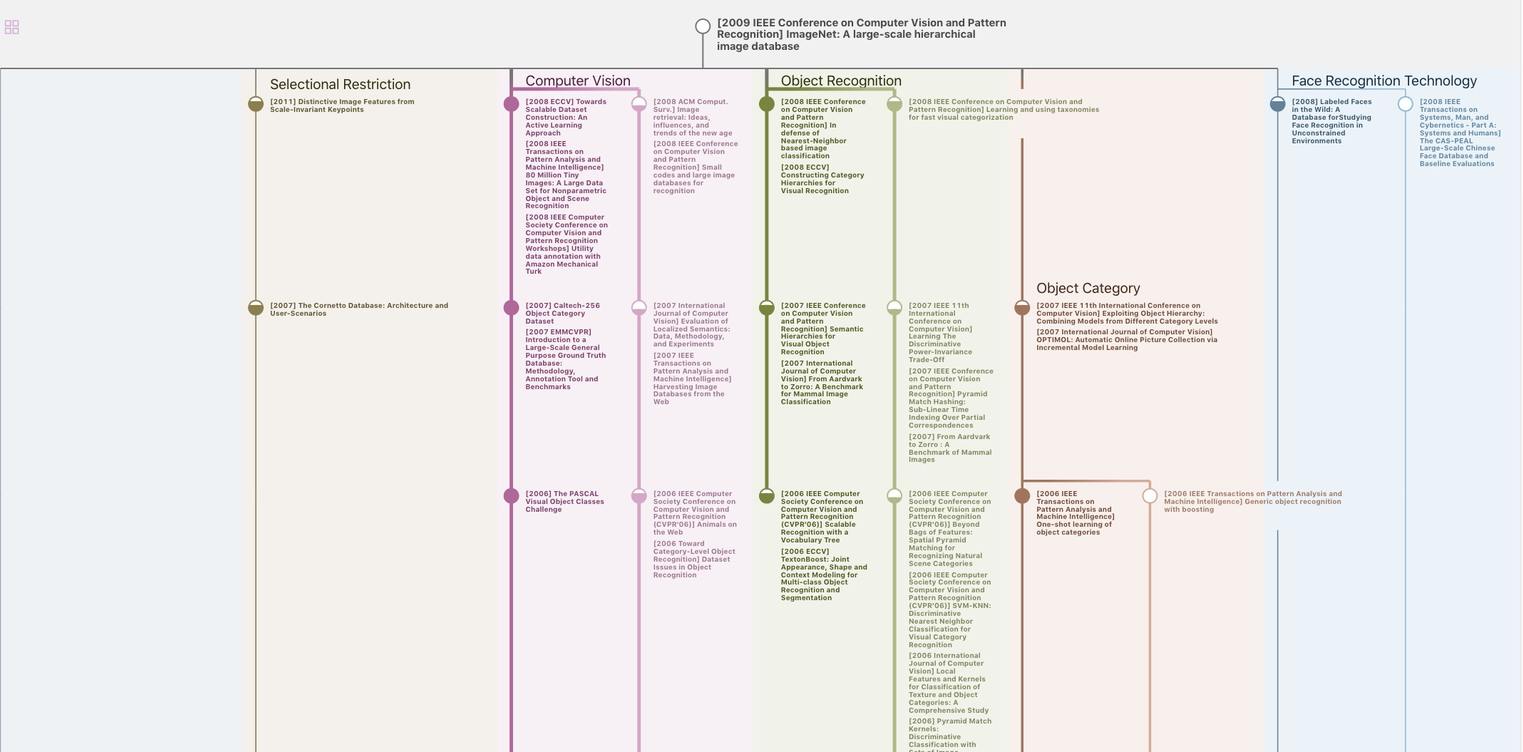
生成溯源树,研究论文发展脉络
Chat Paper
正在生成论文摘要