SUBANOM: Efficient Subgraph Anomaly Detection Framework over Dynamic Graphs
2023 23RD IEEE INTERNATIONAL CONFERENCE ON DATA MINING WORKSHOPS, ICDMW 2023(2023)
摘要
Given a dynamic graph, the efficient tracking of anomalous subgraphs via their node embeddings poses a significant challenge. Addressing this issue necessitates an effective scoring mechanism and an innovative anomalous subgraph strategy. Existing methods predominantly focus on designing scoring strategies or employing graph structures that consider nodes in isolation, resulting in ineffective capture of the anomalous subgraph structure information. In this paper, we introduce SUBANOM, a novel framework for subgraph anomaly detection that is adept at identifying anomalous subgraphs. SUBANOM has three key components: 1) We implement current state-of-the-art dynamic embedding methods to efficiently calculate node embeddings, thereby capturing all node-level anomalies successfully; 2) We devise novel subgraph identification strategies, which include k-hop and triadic-closure. These strategies form the crucial component that can proficiently differentiate between strong and weak neighbors, thus effectively capturing the anomaly structure information; 3) For qualifying the anomaly subgraphs, we propose using l(p)-norm-based score aggregation functions. These iterative steps enable us to process large-scale dynamic graphs effectively. Experiments conducted on a real-world dynamic graph underscore the efficacy of our framework in detecting anomalous subgraphs, outperforming state-of-the-art methods. Experimental results further signify that our framework is a potent tool for identifying anomalous subgraphs in real-world scenarios. For instance, the F1 score under the optimal subgraph identification strategy, can peak at 0.6679, while the highest achievable score using the corresponding baseline method is 0.5677.
更多查看译文
关键词
Subgraph Anomaly Detection,Dynamic Graph Embedding,Triadic Closure
AI 理解论文
溯源树
样例
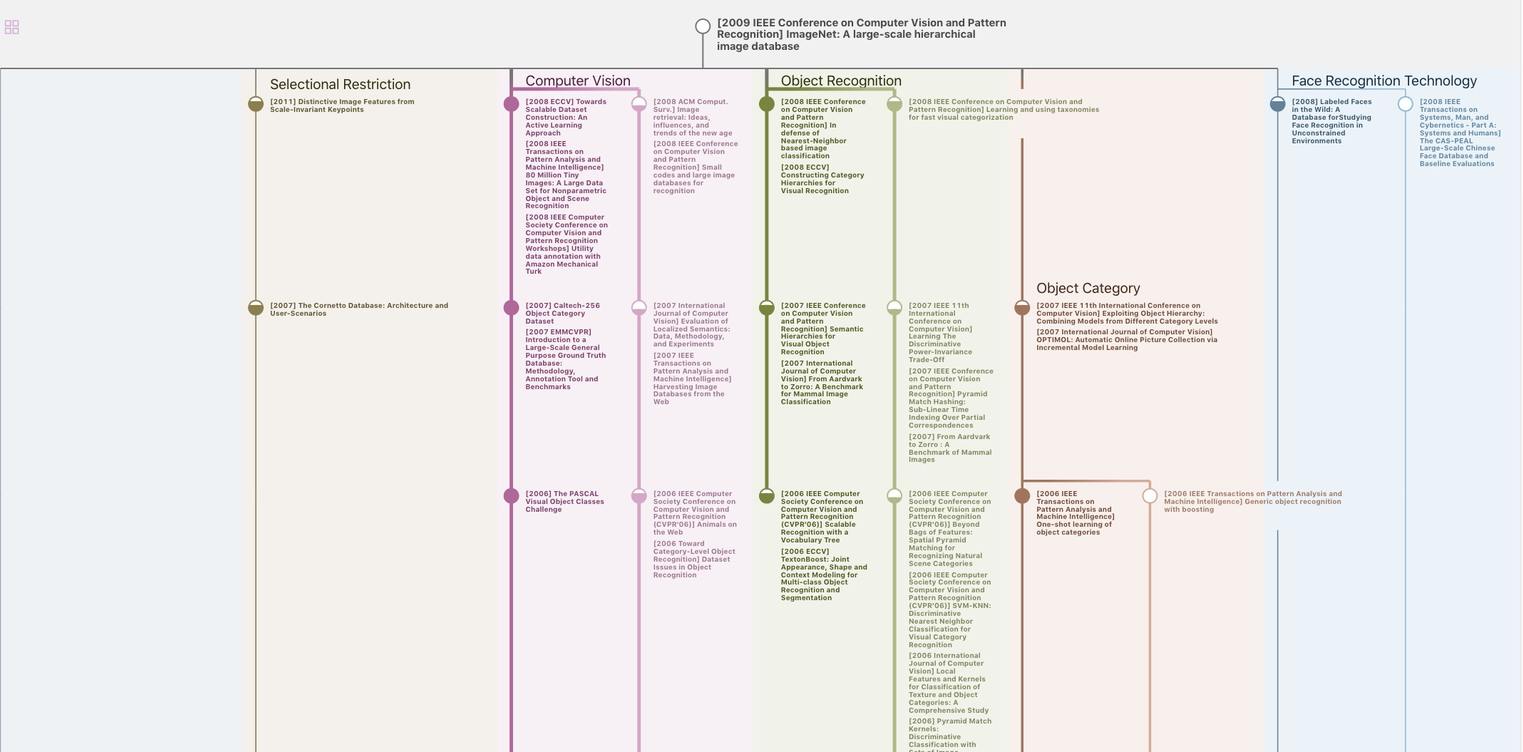
生成溯源树,研究论文发展脉络
Chat Paper
正在生成论文摘要