Spatio-temporal fusion graph convolutional network for traffic flow forecasting
INFORMATION FUSION(2024)
摘要
In most recent research, the traffic forecasting task is typically formulated as a spatiotemporal graph modeling problem. For spatial correlation, they typically learn the shared pattern (i.e., the most salient pattern) of traffic series and measure the interdependence between traffic series based on a predefined graph. On the one hand, learning a specific traffic pattern for each node (traffic series) is crucial and essential for accurate spatial correlation learning. On the other hand, most predefined graphs cannot accurately represent the interdependence between traffic series because they are unchangeable while the prediction task changes. For temporal correlation, they usually concentrate on contiguous temporal correlation. Therefore, they are insufficient due to their lack of global temporal correlation learning. To overcome these aforementioned limitations, we propose a novel method named Spatio-Temporal Fusion Graph Convolutional Network (STFGCN). In the spatial aspect, we introduce a node-specific graph convolution operation to learn the node-specific patterns of each node (traffic series). Then, an adaptive adjacent matrix is introduced to represent the interdependence between traffic series. In the temporal aspect, a contiguous temporal correlation learning module is introduced to learn the contiguous temporal correlation of traffic series. Furthermore, a transformer-based global temporal correlation learning module is introduced to learn the global dependence of the traffic series. Experimental results show that our method significantly outperforms other competitive methods on two real-world traffic datasets (PeMSD4 and PeMSD8).
更多查看译文
关键词
Graph convolutional network,Spatio-temporal data,Traffic forecasting
AI 理解论文
溯源树
样例
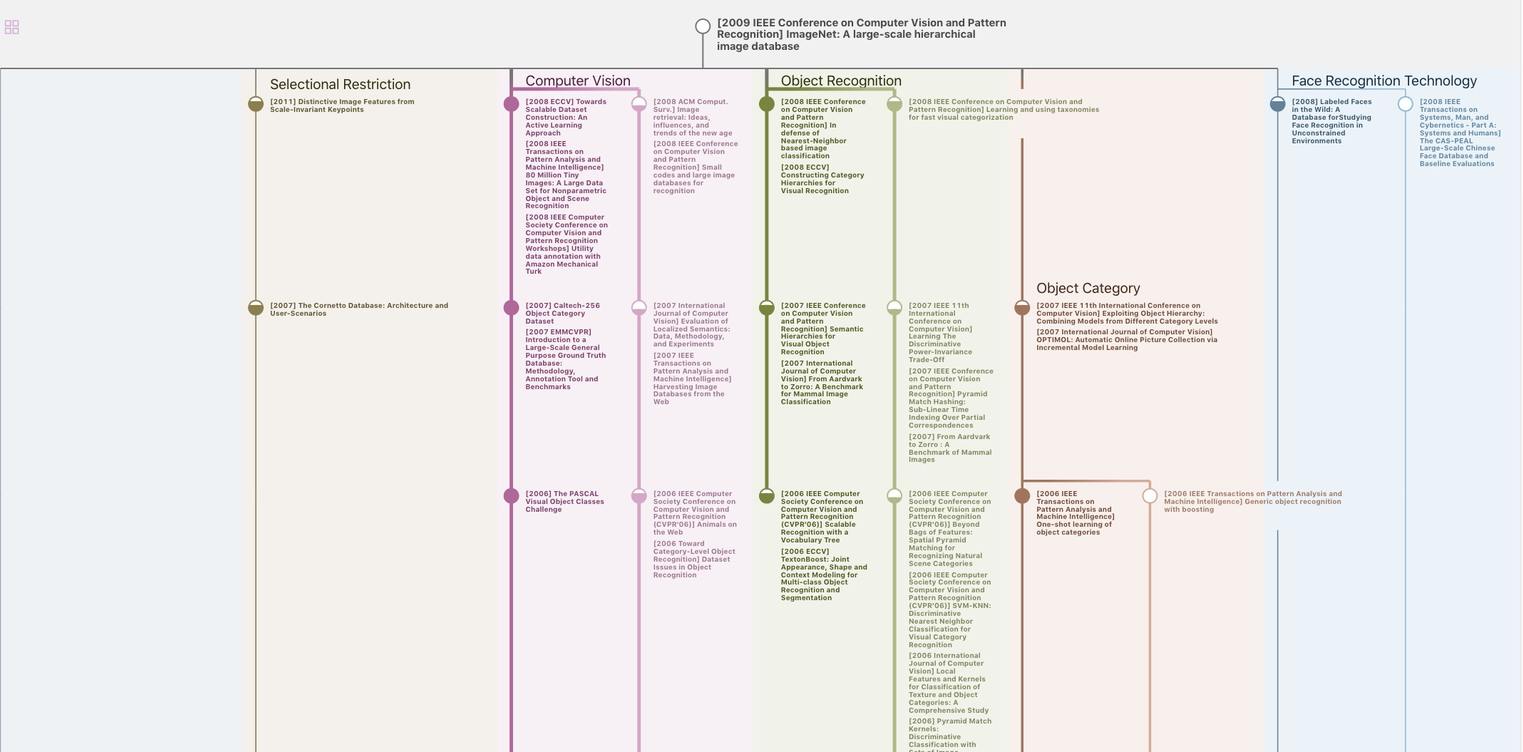
生成溯源树,研究论文发展脉络
Chat Paper
正在生成论文摘要