CDSC: Causal decomposition based on spectral clustering
INFORMATION SCIENCES(2024)
摘要
Causal discovery is pivotal across numerous scientific and engineering disciplines. General causal discovery methods often falter in accuracy and efficiency when dealing with high-dimensional data due to the curse of dimensionality. While recent advancements in recursive constraint -based methods offer some improvements for high-dimensional causal discovery, their reliance on high-order conditional independence (CI) tests in causal decomposition hinders efficiency and accuracy. To address this challenge, we introduce a novel causal decomposition method based on spectral clustering, called CDSC, tailored for enhanced high-dimensional causal discovery. Concretely, CDSC first constructs the Laplacian matrix of the original variable set based on correlation distance. Then, CDSC decomposes the original variable set into multiple subsets without CI tests and recovers the causal structure of each subset. After determining these causal structures, CDSC merges these sub-results into a complete causal structure. A notable advantage of CDSC over other causal decomposition methods lies in its use of simplified spectral clustering. CDSC preserves the connectivity of the subsets to avoid destroying the causality in partitioned subsets. Moreover, we analyze the validity and time complexity of CDSC theoretically. Exclusive experiments on various real-world causal structures show that CDSC outperforms the state-of-the -arts both in accuracy and efficiency significantly.
更多查看译文
关键词
Causal discovery,Causal decomposition,Spectral clustering,Conditional independence test
AI 理解论文
溯源树
样例
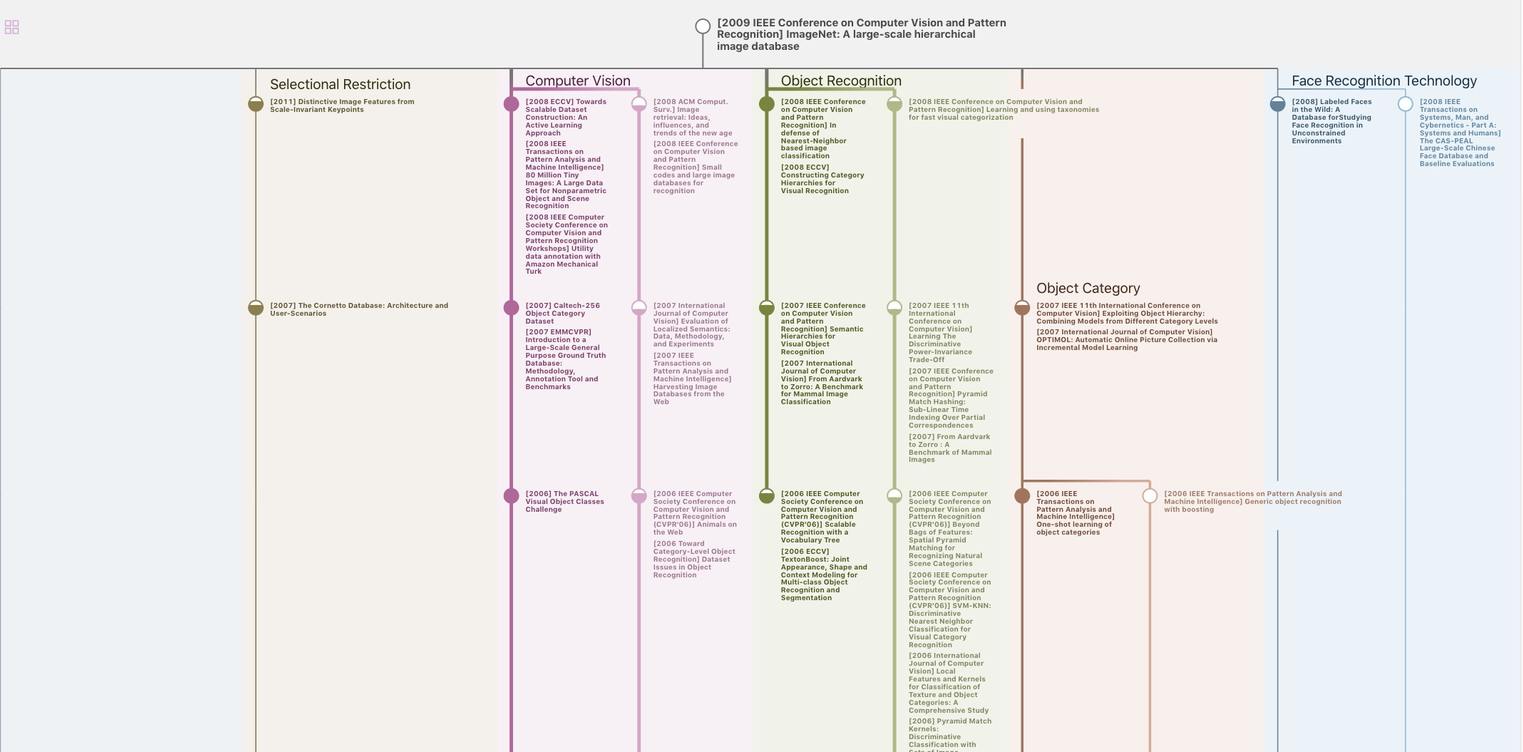
生成溯源树,研究论文发展脉络
Chat Paper
正在生成论文摘要