Global heterogeneous graph enhanced category-aware attention network for session-based recommendation
EXPERT SYSTEMS WITH APPLICATIONS(2024)
摘要
Session-based recommendation (SBR) aims at predicting the next item based on an ongoing recorded session of user's behaviors. Most of existing approaches mainly focus on capturing various item relations such as transitions, co-occurrences, via item identifiers (IDs). In such model, item attributes, such as category, are simply ignored. A few approaches try to explore items' category information to design SBR model. However, due to the dynamically changing preferences of users, it is challenging to exactly pinpoint which category of items is currently of interest to the user. We argue that since a single session faces a serious problem of category information sparsity, the category context of other similar sessions can help to model user category interests more accurately. To model user intent more accurately, the intra-category information is vital beside the inter-category user interest modeling.In this paper, we propose a Heterogeneous Graph Enhanced Category-aware Attention Network (HGCAN) as a solution model to accomplish both two tasks of estimating user's category intent and predicting items for recommendation. The architecture of HGCAN consists of a weighted heterogeneous graph attention network (WHGAT) on global graph for item and category embeddings learning and a category-aware attention network for session representations learning. Experiments on three public datasets validate our HGCAN outperforms these state-of-the-art models.1
更多查看译文
关键词
Recommender system,Session-based recommendation,Graph neural network
AI 理解论文
溯源树
样例
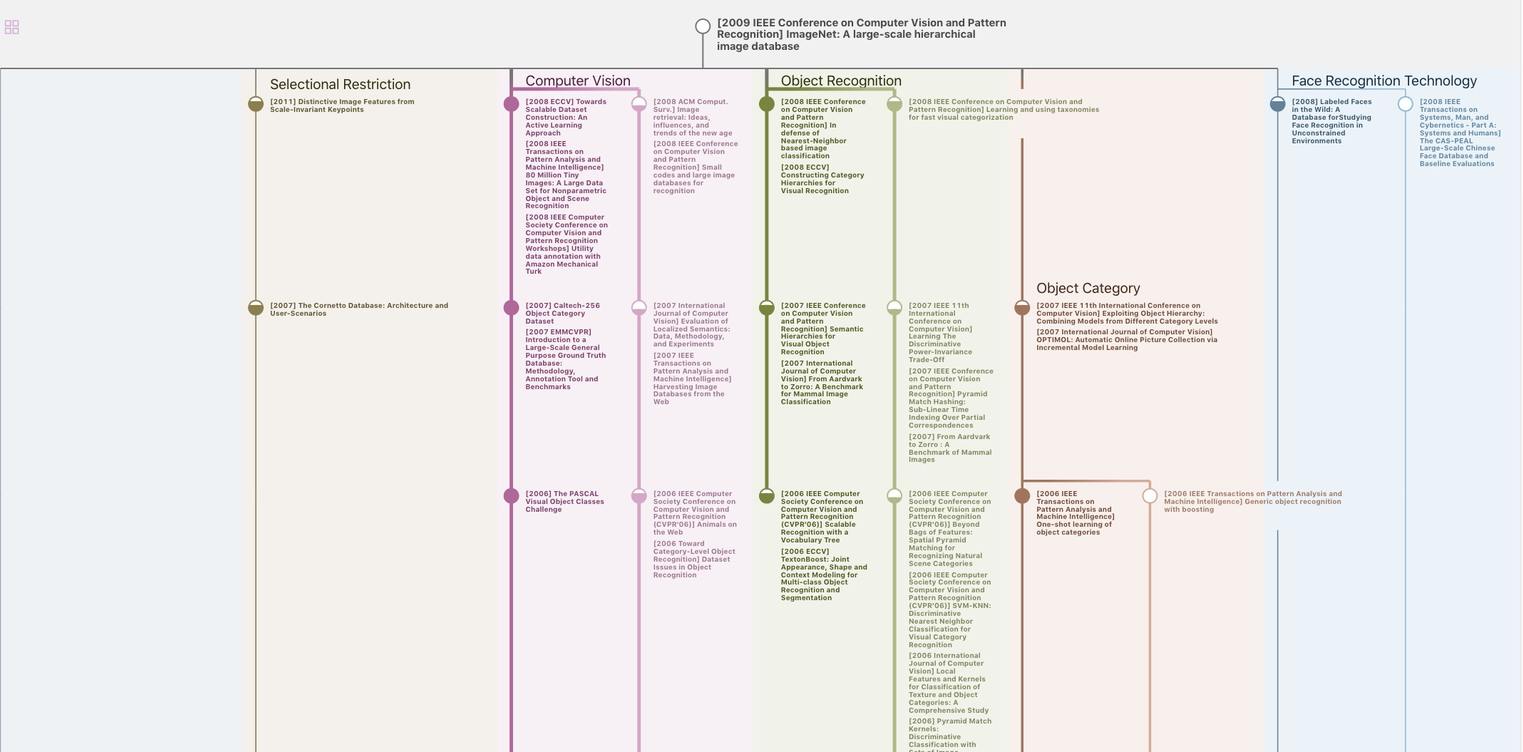
生成溯源树,研究论文发展脉络
Chat Paper
正在生成论文摘要