An integrated neural network method for landslide susceptibility assessment based on time-series InSAR deformation dynamic features
INTERNATIONAL JOURNAL OF DIGITAL EARTH(2024)
摘要
We develop an integrated neural network landslide susceptibility assessment (LSA) method that integrates temporal dynamic features of interferometry synthetic aperture radar (InSAR) deformation data and the spatial features of landslide influencing factors. We construct a time-distributed convolutional neural network (TD-CNN) and bidirectional gated recurrent unit (Bi-GRU) to better understand the temporal dynamic features of InSAR cumulative deformation, and construct a multi-scale convolutional neural network (MSCNN) to determine the spatial features of landslide influencing factors, and construct a parallel unified deep learning network model to fuse these temporal and spatial features for LSA. Compared with the traditional MSCNN method, the accuracy of the proposed model is improved by 1.20%. The performance of the proposed model is preferable to MSCNN. The area under the receiver operating characteristic curve (AUC) of the testing set reaches 0.91. Our LSA results show that the proposed model clearly depicts areas with very high susceptibility landslides. Further, only 10.18% of the study area accurately covers 84.79% of historical landslide areas. Subjective consequences and objective indicators show that the proposed model that is integrated time-series InSAR deformation dynamic features can make full use of landslide characteristics and effectively improve the reliability of LSA.
更多查看译文
关键词
Landslide susceptibility assessment,InSAR,time distributed convolutional neural network,bidirectional gated recurrent unit,remote sensing
AI 理解论文
溯源树
样例
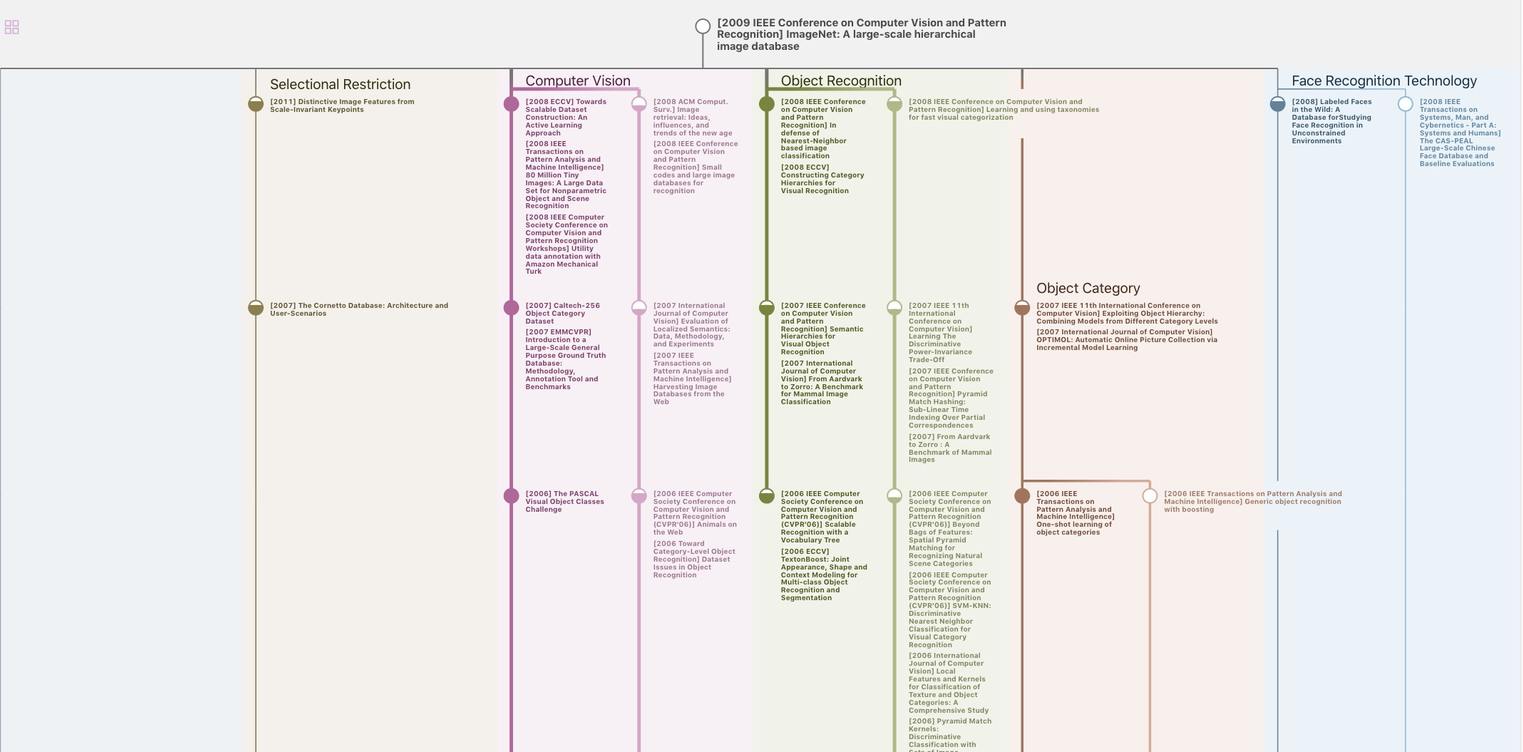
生成溯源树,研究论文发展脉络
Chat Paper
正在生成论文摘要