Comprehensive data-driven methods for estimating the thermal conductivity of biodiesels and their blends with alcohols and fossil diesels
RENEWABLE ENERGY(2024)
Abstract
Biodiesels offer viable alternatives to fossil diesels as renewable and clean energy sources. Understanding the thermal conductivity of biodiesels is crucial for engine design and heat transfer rate calculations in thermal systems. However, existing literature lacks validated predictive tools for this property. To address this, a study utilized 2552 data points from 18 published studies with uncertainties less than 2 %, covering 26 fatty acid alkyl ester biodiesels, 12 biodiesel-alcohol mixtures, and 9 biodiesel-fossil diesel blends across various pressure and temperature conditions. Three machine learning algorithms were employed, using input variables of reduced temperature, reduced pressure, and average molecular weight. Models were trained on 80 % of the data and evaluated on the remaining 20 %. Statistical error metrics and graphical analysis confirmed high precision in predicting biodiesel thermal conductivity. The Gaussian Process Regression model showed the best consistency, with an average absolute relative error (AARE) of 0.36 % and determination coefficient (R2) of 99.69 % on the testing dataset. These models were versatile, applicable to different biodiesel mixtures, accurately capturing thermal conductivity behavior under diverse operating conditions. In contrast, existing literature correlations were limited to specific conditions and required complex calculations. Additionally, a sensitivity analysis determined the relative importance of factors influencing thermal conductivity.
MoreTranslated text
Key words
Biodiesel fuels,Thermal conductivity,Intelligent approaches,Modeling
AI Read Science
Must-Reading Tree
Example
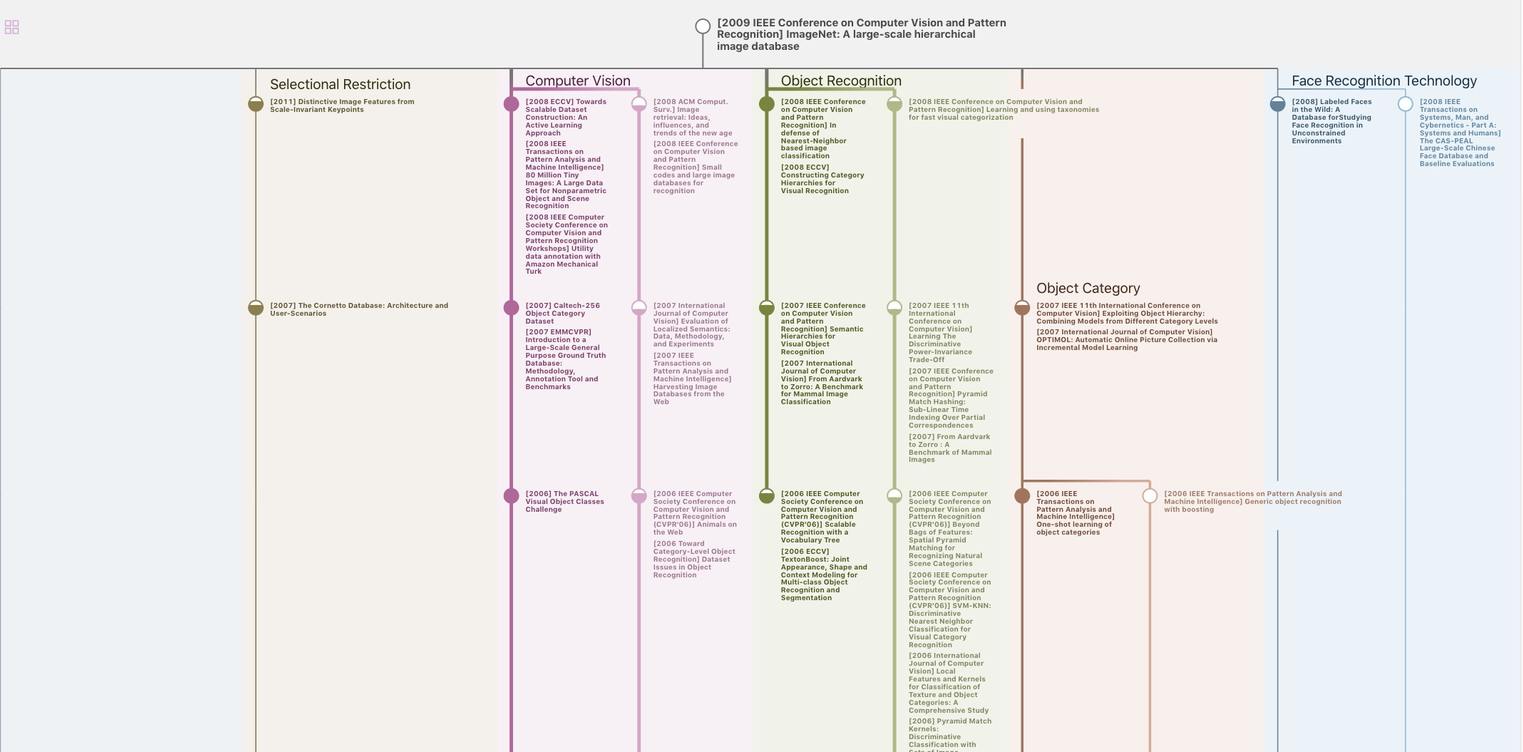
Generate MRT to find the research sequence of this paper
Chat Paper
Summary is being generated by the instructions you defined