Fundamental Study on Image Improvement of X-ray Fluorescence Computed Tomography by Deep Image Prior
2023 IEEE Nuclear Science Symposium, Medical Imaging Conference and International Symposium on Room-Temperature Semiconductor Detectors (NSS MIC RTSD)(2023)
摘要
We are developing an X-ray fluorescence computed tomography (XFCT) system using nonradioactive contrast agents (e.g. iodine compounds) for preclinical studies as a new modality that provides biological function images like positron emission tomography (PET) and single photon emission computed tomography (SPECT). To perform in vivo imaging of small animals with XFCT, it is necessary to improve the image quality and detection limit. The aim of this study is to improve image quality of XFCT by applying deep image prior (DIP), a type of convolutional neural network, to image reconstruction. DIP can restore image with only the target image acquired by XFCT. Projection images were processed with DIP for noise reduction after scatter correction. Reconstructed images were generated by ML-EM method for multi-pinhole XFCT. To evaluate the image improvement effect, physical phantom experiments were performed using synchrotron monochromatic X-rays as excitation light. The contrast-to-noise ratio of the reconstructed image was greatly improved by 4.6 times in the 0.3 mg/ml iodine solution region, and the detection limit improved from 0.069 mg/ml to 0.035 mg/ml. This study demonstrated that reconstruction using DIP could greatly improve the image quality and detection limit of XFCT without degrading the spatial resolution.
更多查看译文
AI 理解论文
溯源树
样例
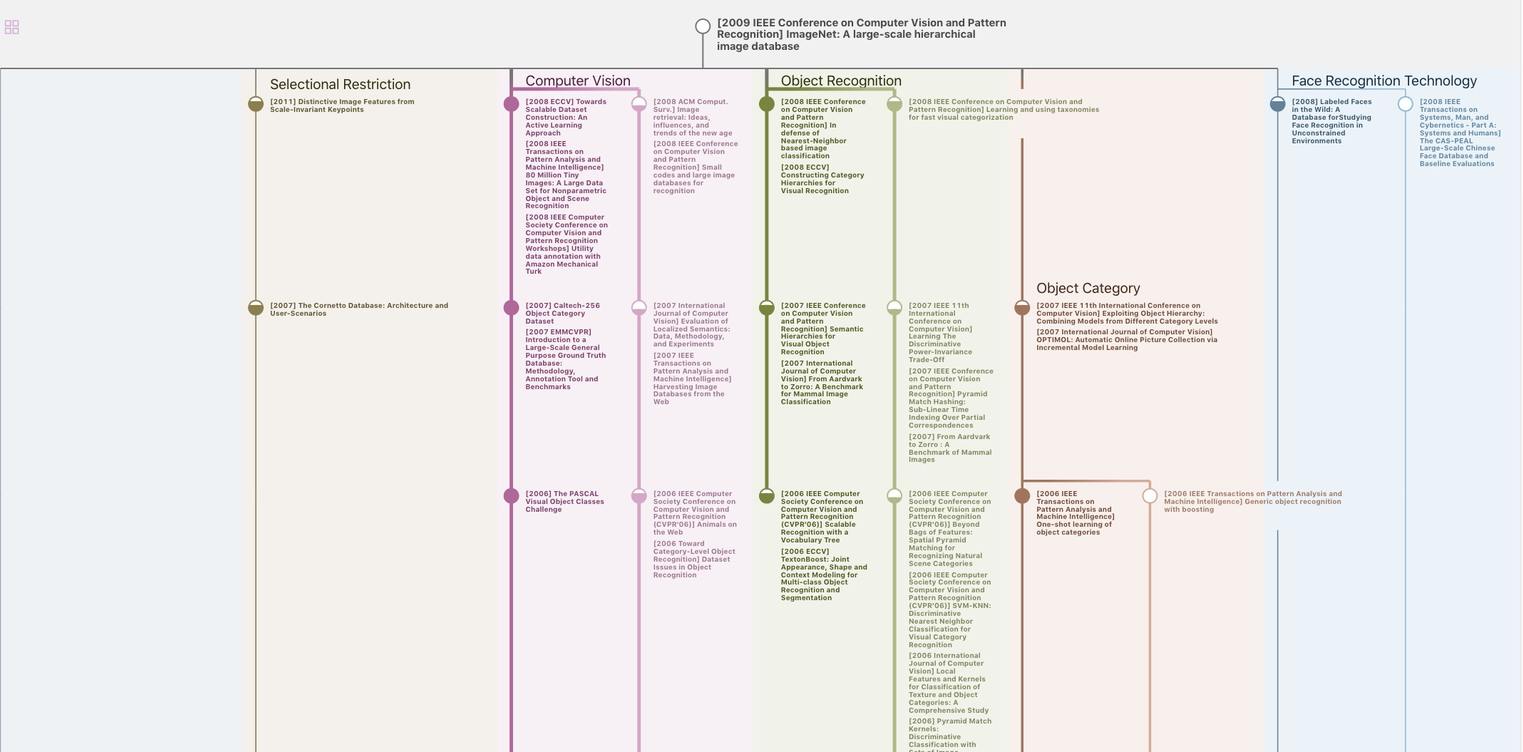
生成溯源树,研究论文发展脉络
Chat Paper
正在生成论文摘要