ReconU-Net: Direct PET Image Reconstruction Using Back Projection-induced Skip Connection
2023 IEEE Nuclear Science Symposium, Medical Imaging Conference and International Symposium on Room-Temperature Semiconductor Detectors (NSS MIC RTSD)(2023)
Abstract
Direct positron emission tomography (PET) image reconstruction methods using convolutional neural networks (CNNs) have attracted increasing attention for the next generation of PET image reconstruction. These CNN-based direct reconstruction methods generate good reconstructed images from sinograms. However, it is difficult to obtain an accurate inverse transformation from sinograms to reconstructed images using a data-driven approach, and these direct reconstruction methods may produce finer "false" PET images with different structures from the ground truth. In this study, we propose a novel back projection-induced U-Net shape architecture, called ReconU-Net, for direct PET image reconstruction. The ReconU-Net guides the connections of the features from the encoder, expressed in sinogram space, to the decoder, expressed in the reconstructed image space by back-projection operations in the skip connection. Hence, the proposed ReconU-Net architecture can retain accurate spatial information from the input sinograms, according to the physical model. We generated and trained the proposed ReconU-Net with Monte Carlo simulation data from the BrainWeb phantom and tested it using simulation data and real brain PET data. Compared with the maximum likelihood expectation maximization and the DeepPET, the proposed ReconU-Net method outperforms the reconstruction performance in peak signal-to-noise ratio and can generate finer "true" PET images. In addition, the proposed ReconU-Net method successfully reconstructed the real brain PET data. Therefore, we concluded that the proposed ReconU-Net architecture is a practical solution for CNN-based direct PET image reconstruction.
MoreTranslated text
AI Read Science
Must-Reading Tree
Example
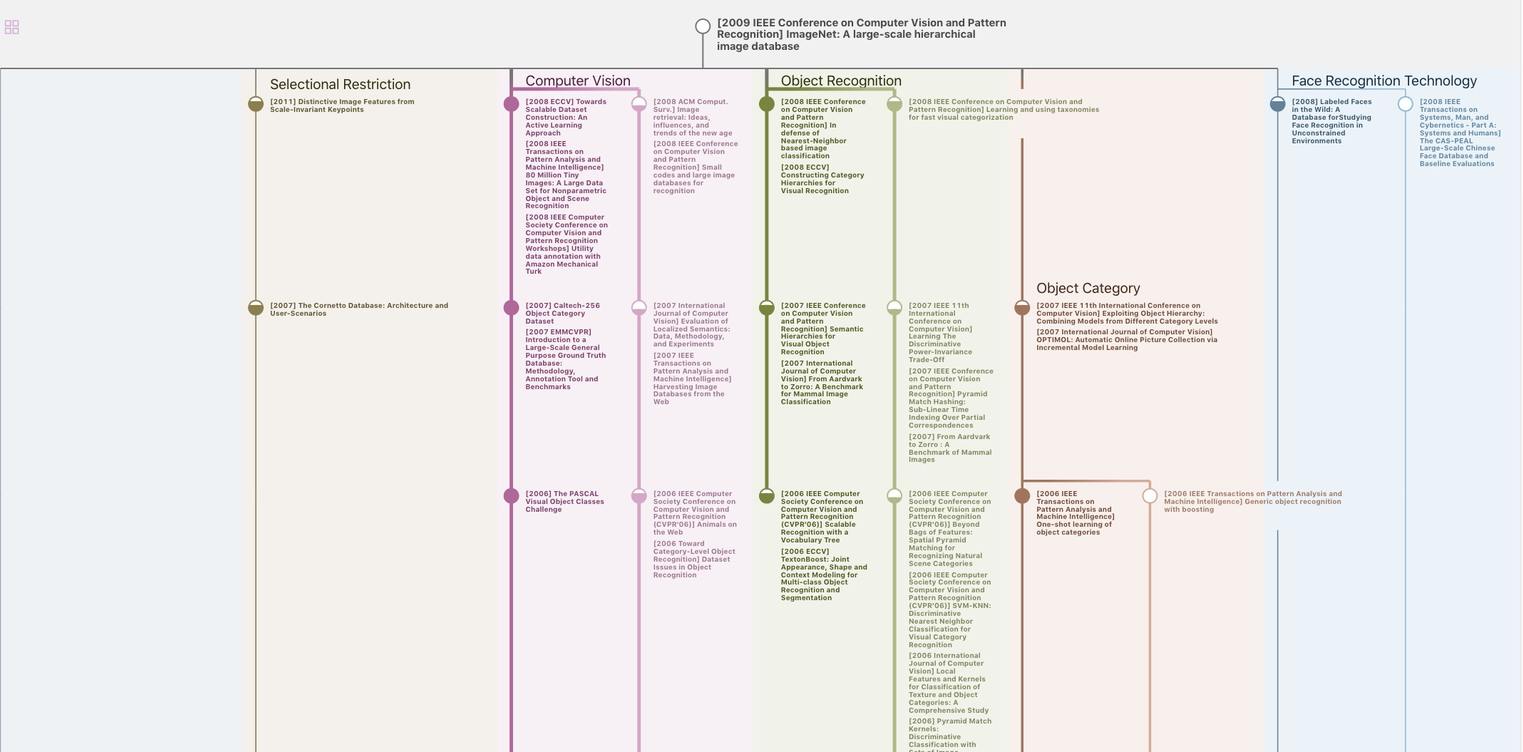
Generate MRT to find the research sequence of this paper
Chat Paper
Summary is being generated by the instructions you defined