Hybrid Object Tracking with Events and Frames
2023 IEEE/RSJ INTERNATIONAL CONFERENCE ON INTELLIGENT ROBOTS AND SYSTEMS (IROS)(2023)
摘要
Robust object pose tracking plays an important role in robot manipulation, but it is still an open issue for quickly moving targets as motion blur and low frequency detection can reduce pose estimation accuracy even for state-of-the-art RGB-D-based methods. An event-camera is a low-latency vision sensor that can act complementary to RGB-D. Specifically, its sub-millisecond temporal resolution can be exploited to correct for pose estimation inaccuracies due to low frequency RGB-D based detection. To do so, we propose a dual Kalman filter: the first filter estimates an object's velocity from the spatio-temporal patterns of "events", the second filter fuses the tracked object velocity with a low-frequency object pose estimated from a deep neural network using RGB-D data. The full system outputs high frequency, accurate object poses also for fast moving objects. The proposed method works towards low-power robotics by replacing high-cost GPU-based optical flow used in prior work with event-cameras that inherently extract the required signal without costly processing. The proposed algorithm achieves comparable or better performance when compared to two state-of-the-art 6-DoF object pose estimation algorithms and one hybrid event/RGB-D algorithm on benchmarks with simulated and real data. We discuss the benefits and trade-offs for using the event-camera and contribute algorithm, code, and datasets to the community. The code and datasets are available at https://github.com/event-driven-robotics/Hybrid-object-tracking-with-events-and-frames.
更多查看译文
AI 理解论文
溯源树
样例
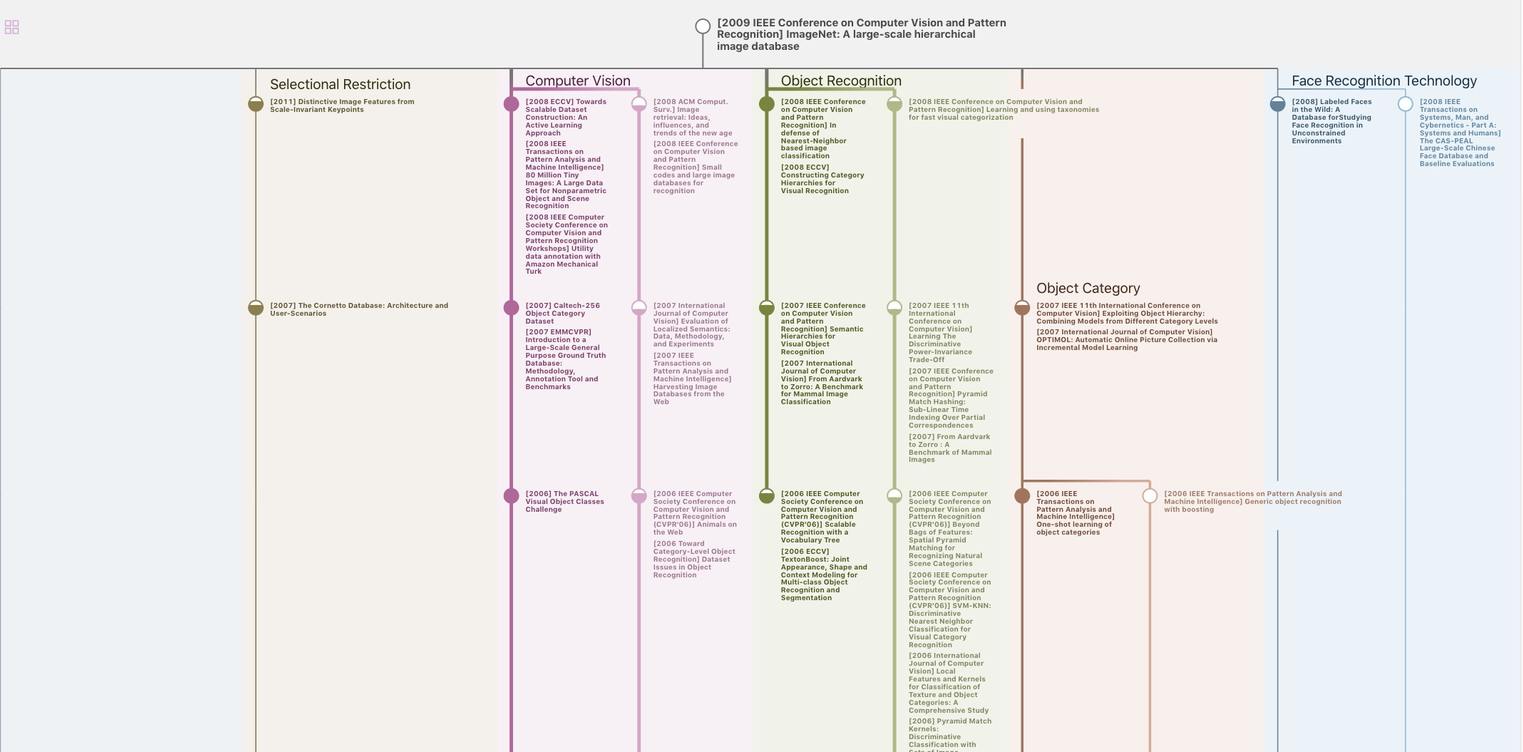
生成溯源树,研究论文发展脉络
Chat Paper
正在生成论文摘要