Cross-Domain Autonomous Driving Perception using Contrastive Appearance Adaptation
2023 IEEE/RSJ INTERNATIONAL CONFERENCE ON INTELLIGENT ROBOTS AND SYSTEMS, IROS(2023)
摘要
Addressing domain shifts for complex perception tasks in autonomous driving has long been a challenging problem. In this paper, we show that existing domain adaptation methods pay little attention to the content mismatch issue between source and target domains, thus weakening the domain adaptation performance and the decoupling of domain-invariant and domainspecific representations. To solve the aforementioned problems, we propose an image-level domain adaptation framework that aims at adapting source-domain images to the target domain with content-aligned source-target image pairs. Our framework consists of three mutually beneficial modules in a cycle: a cross-domain content alignment module to generate sourcetarget pairs with consistent content representations in a selfsupervised manner, a reference-guided image synthesis based on the generated content-aligned source-target image pairs, and a contrastive learning module to self-supervise domain-invariant feature extractor. Our contrastive appearance adaptation is taskagnostic and robust to complex perception tasks in autonomous driving. Our proposed method demonstrates state-of-the-art results in cross-domain object detection, semantic segmentation, and depth estimation as well as better image synthesis ability qualitatively and quantitatively.
更多查看译文
AI 理解论文
溯源树
样例
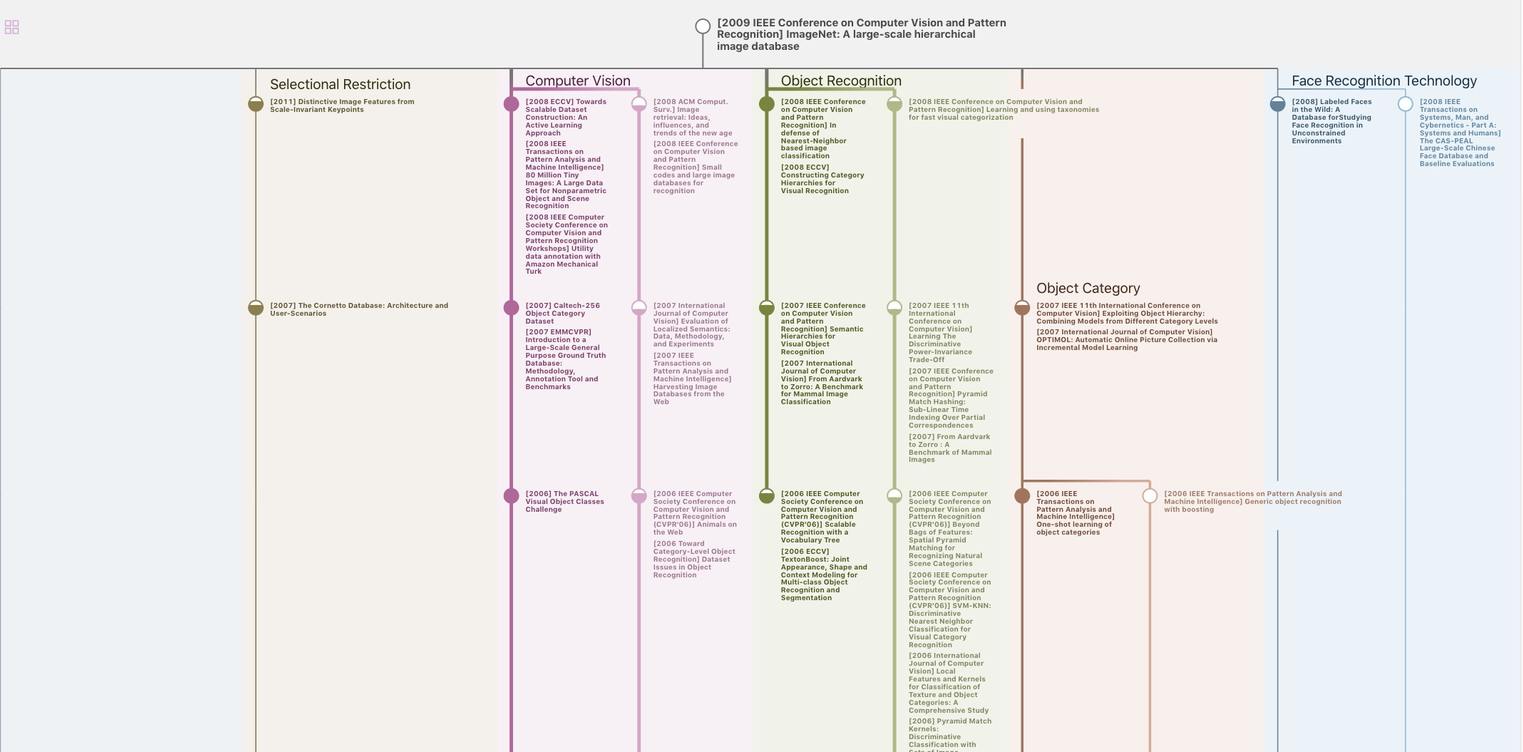
生成溯源树,研究论文发展脉络
Chat Paper
正在生成论文摘要