Multi-View Robust Collaborative Localization in High Outlier Ratio Scenes Based on Semantic Features
2023 IEEE/RSJ INTERNATIONAL CONFERENCE ON INTELLIGENT ROBOTS AND SYSTEMS (IROS)(2023)
Abstract
Filtering out outlier data associations between local maps can improve the robustness and accuracy of multi-robot localization. When the overlap is low and the field of view difference is large, it is likely to produce outlier data associations between local maps, which will reduce the matching accuracy and even lead to the failure of collaborative localization. To solve this problem, this paper proposes a novel outdoor robust collaborative localization algorithm (HORCL) capable for high outlier ratio scenes. The Mixture Probability Model (MPM) and the Hierarchical EM (Expectation Maximization) algorithm in HORCL are applied to screen two levels of outliers (loop closure constraints and point pairs) and improve localization performance. Specifically, the inlier probabilities of data associations are calculated in MPM to identify outliers by considering geometric distances, semantic consistency, and spatial consistency. Then, outlier loop closures and outlier point pairs in inlier constraints are filtered by applying the Hierarchical EM algorithm, thereby relieving the adverse effect of outliers on localization accuracy. The proposed algorithm is validated on public datasets and compared with the latest methods, demonstrating the improvement in localization accuracy and robustness. The code is available at https://github.com/BIT-TYJ/HORCL.
MoreTranslated text
AI Read Science
Must-Reading Tree
Example
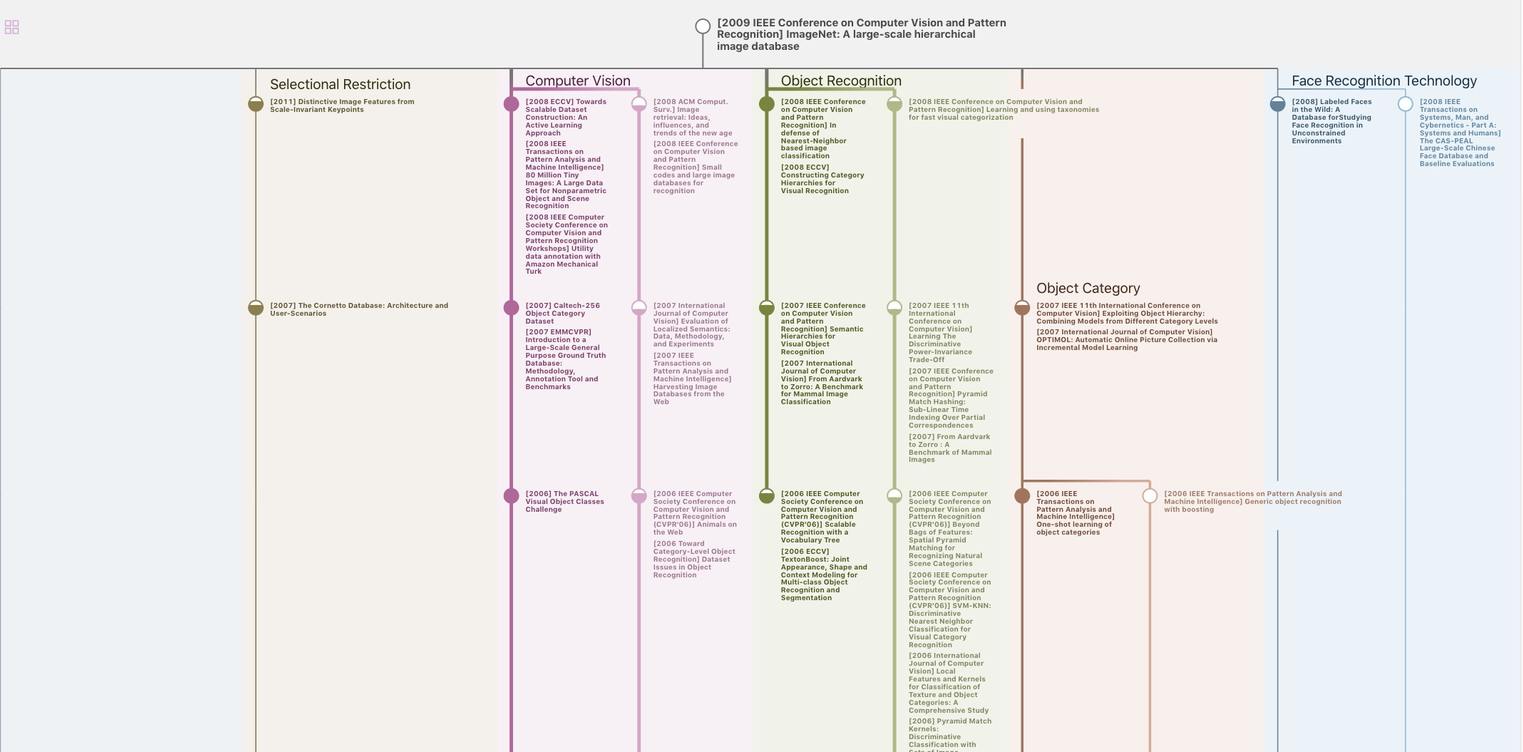
Generate MRT to find the research sequence of this paper
Chat Paper
Summary is being generated by the instructions you defined