Autonomous Swarm Robot Coordination via Mean-Field Control Embedding Multi-Agent Reinforcement Learning
2023 IEEE/RSJ INTERNATIONAL CONFERENCE ON INTELLIGENT ROBOTS AND SYSTEMS (IROS)(2023)
摘要
The learning approaches of designing a controller to guide the collective behavior of swarm robots have gained significant attention in recent years. However, the scalability of swarm robots and their inherent stochasticity complicate the control problem due to increasing complexity, unpredictability, and non-linearity. Despite considerable progress made in swarm robotics, addressing these challenges remains a significant issue. In this work, we model the stochastic dynamics of a swarm robot system and then propose a novel control framework based on a mean-field control (MFC) embedding multi-agent reinforcement learning (MARL) approach named MF-MARL to deal with these challenges. While MARL is able to deal with stochasticity statistically, we integrate MFC, allowing MF-MARL to cope with large-scale robots. Moreover, we apply statistical moments of robots' state and control action to discretize continuous input and enable MF-MARL to be applied in continuous scenarios. To demonstrate the effectiveness of MF-MARL, we evaluate the performance of the robots on a specific swarm simulation platform. The experimental results show that our algorithm outperforms the traditional algorithms both in navigation and manipulation tasks. Finally, we demonstrate the adaptability of the proposed algorithm through the component failure test.
更多查看译文
AI 理解论文
溯源树
样例
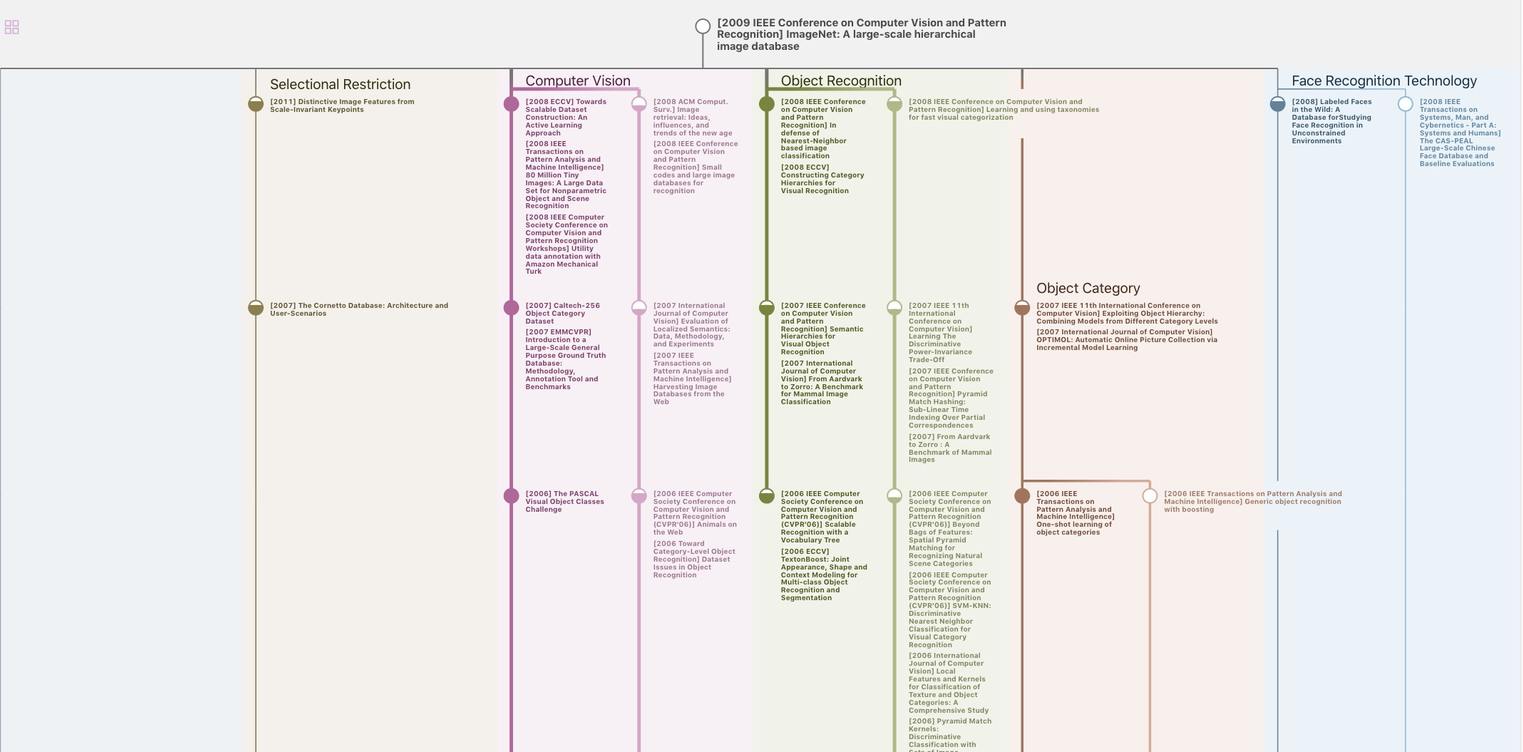
生成溯源树,研究论文发展脉络
Chat Paper
正在生成论文摘要