Compact and Accurate Adaptive Width Radial Basis Function Neural Network with Discriminative Features for Thyroid Gland Segmentation
2023 IEEE/RSJ INTERNATIONAL CONFERENCE ON INTELLIGENT ROBOTS AND SYSTEMS (IROS)(2023)
摘要
The goal of this work is to develop a fast, compact, accurate, and robust neural network for automatic thyroid gland segmentation in medical ultrasound images when the computational resources are limited, such as portable intelligent medical diagnostic devices or robots. An adaptive width radial basis function neural network model with discriminative features is proposed in this work. The model combines the local characteristics and global context of the entire feature space, which improves the performance of the automatic thyroid gland segmentation, and enhances the data fitting capability and robustness of the neural network. A new feature selection method combining the measurements of area under the curve values and Pearson correlation coefficients is proposed to select six discriminative and low correlated features from a set of handcrafted features, which helps to construct a compact yet accurate radial basis function neural network. The proposed method is experimented on thyroid ultrasound images using five-fold cross validation. The proposed method achieves an average accuracy of 0.9770 and an average IoU of 0.7841, achieving competitive segmentation performance compared to convolutional neural networks, with thousands of times faster training speed on a CPU.
更多查看译文
AI 理解论文
溯源树
样例
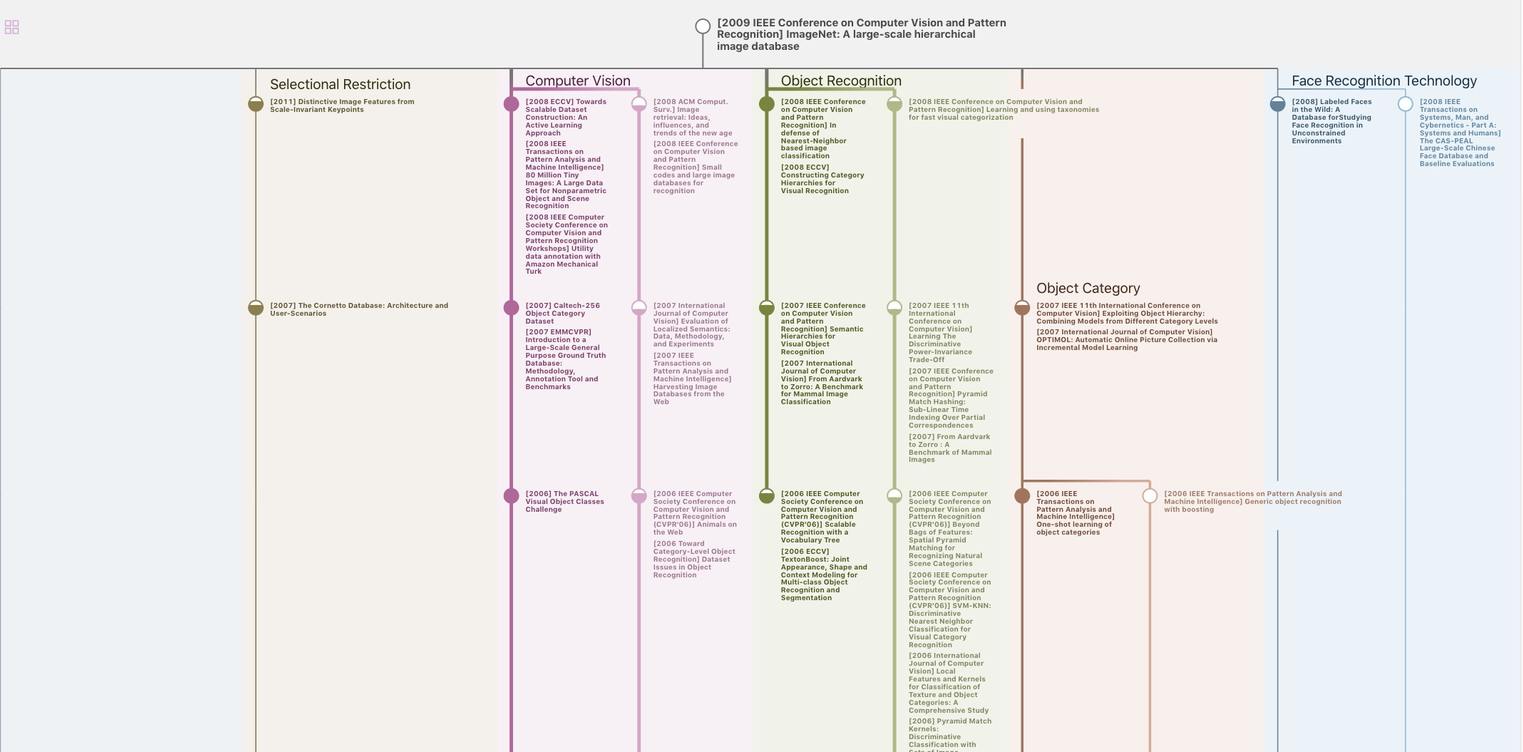
生成溯源树,研究论文发展脉络
Chat Paper
正在生成论文摘要