Enhancing Value Estimation Policies by Post-Hoc Symmetry Exploitation in Motion Planning Tasks
2023 IEEE/RSJ INTERNATIONAL CONFERENCE ON INTELLIGENT ROBOTS AND SYSTEMS, IROS(2023)
摘要
Motion planning tasks are often innately invariant to certain geometric transformations, or in other words, symmetric. This property, however, is not always reflected in learned policies that are trained on these tasks. Although this asymmetry can be addressed through data augmentation or additional training samples, doing so comes at a cost of increased training time. Instead of trying to remedy this issue during the learning process, we leverage this disparity during execution. We propose the symmetry exploitation policy, an augmentation in the post-hoc execution stage of RL policies. During the planning stage, we present the learned policy with an invariant, geometrically transformed version of the observation as an alternate perspective of the state. This allows the policy to produce multiple possible actions for a single state, and choose the action with the highest estimated value. Unlike other symmetry exploitation methods for learning solutions in motion planning, this method completely bypasses the need for additional training. We show the effect of the symmetry exploitation policy on DQN, A2C, and PPO policies, in three motion problems with different dimensions, observation types, and symmetries. The results show that by exploiting the symmetry of the task, a trained model achieves improved performance and better generalization, and can achieve comparable results to retraining, augmentation, or extended training, without incurring any additional training time. The efficacy is most prominent in more complex tasks, as 89 of the 100 models involved in the case study improve when using the method.
更多查看译文
AI 理解论文
溯源树
样例
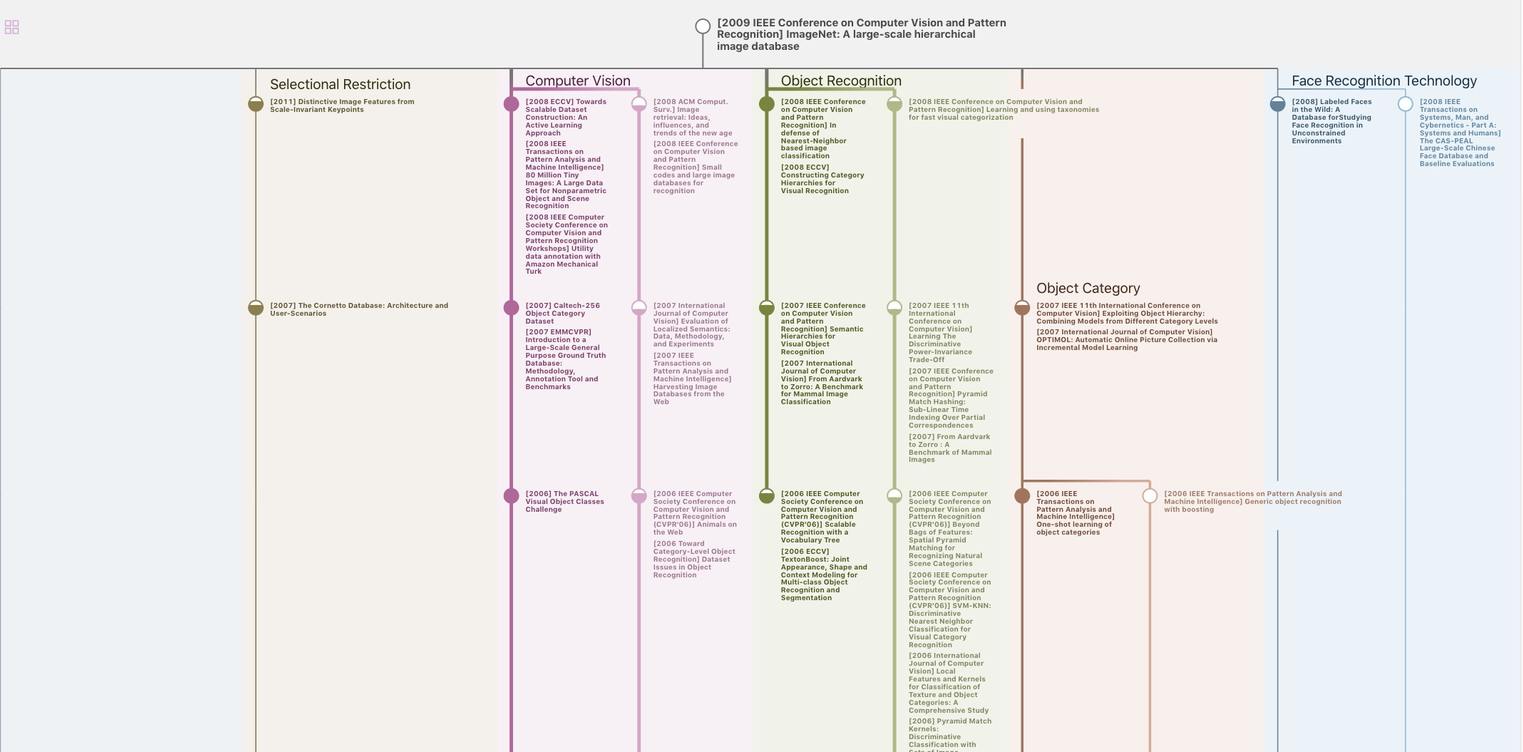
生成溯源树,研究论文发展脉络
Chat Paper
正在生成论文摘要