Knowledge Distillation for Efficient Panoptic Semantic Segmentation: applied to agriculture
2023 IEEE/RSJ INTERNATIONAL CONFERENCE ON INTELLIGENT ROBOTS AND SYSTEMS, IROS(2023)
摘要
Panoptic segmentation provides both holistic and detailed image parsing information at both the pixel and the instance level. However, the computational burdens restrict its applications in real-time scenarios. A potential approach to learn more efficient models is to employ knowledge distillation. However, previous knowledge distillation schemes have focused mainly on classification with limited attention given to regression-related tasks which is key for panoptic segmentation. In this paper, we establish a logits-based, a hints-based, and a combination-based scheme for panoptic knowledge distillation by using logits from the final layers and features in the middle layers. Then we explore different combinations of balancing weights for optimal solutions according to different network structures and datasets. To validate our proposed approach, various experiments on different datasets have been conducted and efficient networks with higher performance have been obtained. We show that knowledge distillation can be applied to develop accurate ResNet-34 networks improving their panoptic quality on things by an absolute amount of 4.1 points for sweet pepper (glasshouse environment) and 2.2 points for sugar beet (arable farming environment). These student ResNet-34 networks are able to run inference at faster than a framerate of 53Hz on computing infrastructure similar to PATHoBot (a glasshouse robot). To the best of our knowledge, this is the first work to propose knowledge distillation schemes for panoptic semantic segmentation.
更多查看译文
关键词
Computer Vision for Agriculture Automation,Knowledge Distillation,Efficient Panoptic Segmentation
AI 理解论文
溯源树
样例
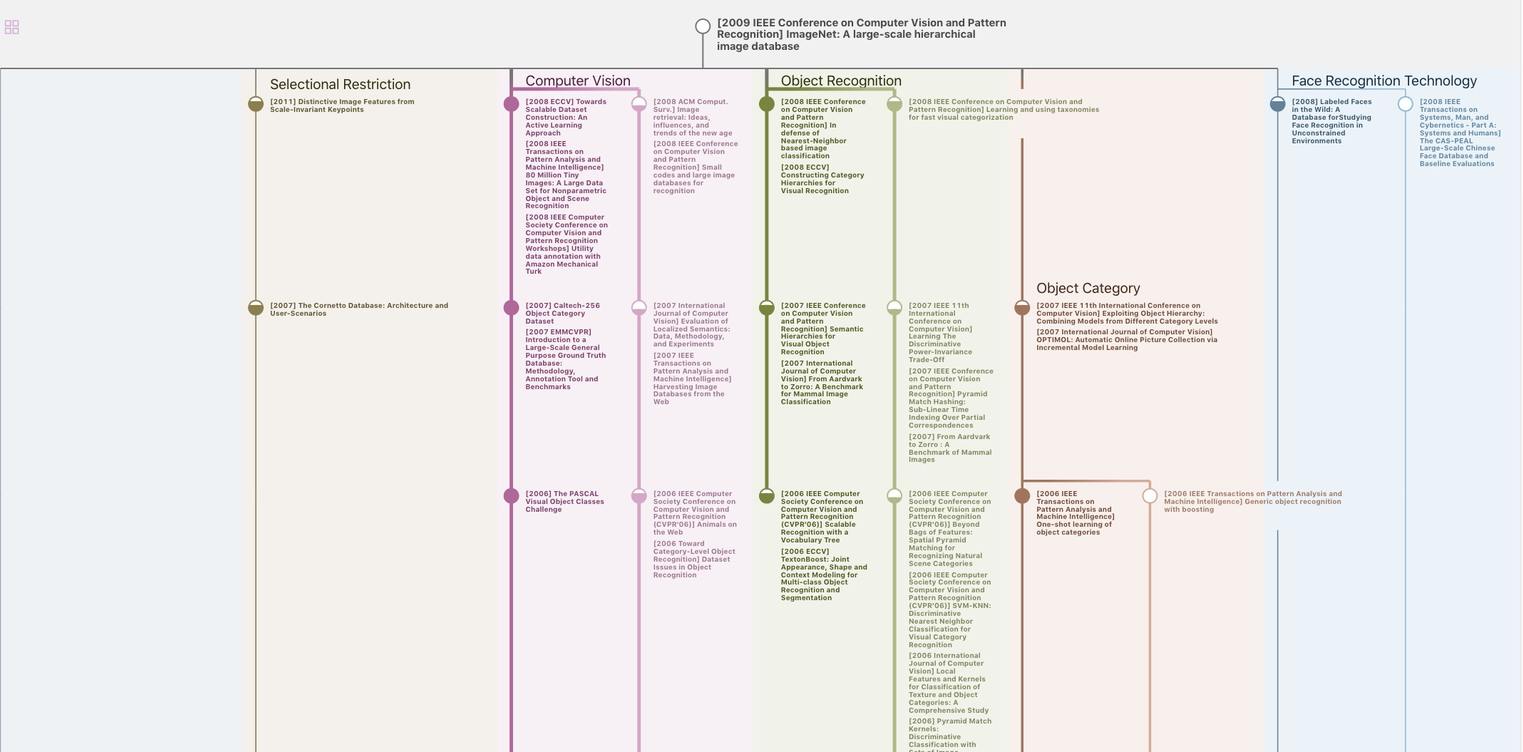
生成溯源树,研究论文发展脉络
Chat Paper
正在生成论文摘要