Portability of Hybrid machine learning based model for anomaly forecasting in mobile networks
2023 IEEE 98TH VEHICULAR TECHNOLOGY CONFERENCE, VTC2023-FALL(2023)
摘要
The management of future cellular networks can be automatically adapted by machine learning models. In this article, we present the portability of a proposed hybrid machine learning model to forecast the network congestion allowing, operators to pro-actively monitor and control future congestion before occurring. To overcome the quality of service degradation of the radio access network, the spatio-temporal hybrid model must involve automatic quick response to forecast future congestion and alert network operators to correct future congested cells to provide a continuous quality of service for the subscribers. Based on real field data of key performance indicators from operational long term evolution networks of Orange, we apply the hybrid machine learning model to ensure spatio- temporal congestion forecasting. In this paper, we present the portability of a proposed hybrid model in space and time. The idea of portability is that knowledge extracted from a specific city environment can be applied directly to another different cities environment. The hybrid model is a combination of a Latent Block Model (LBM) co-clustering technique that succeeds in grouping cells located in distant cities that have a similarity in their behaviors and a logistic regression technique to forecast future congestion in radio access networks. The experiments presented in this article confirm that the LBM co-clustering technique allows the model's portability from a city to another one. In addition, it is applied for the same cells for different months to validate its stability over time.
更多查看译文
关键词
Spatio-Temporal Portability,Congestion forecast,Radio access network,Co-clustering Functional Latent Block Model,functional logistic regression
AI 理解论文
溯源树
样例
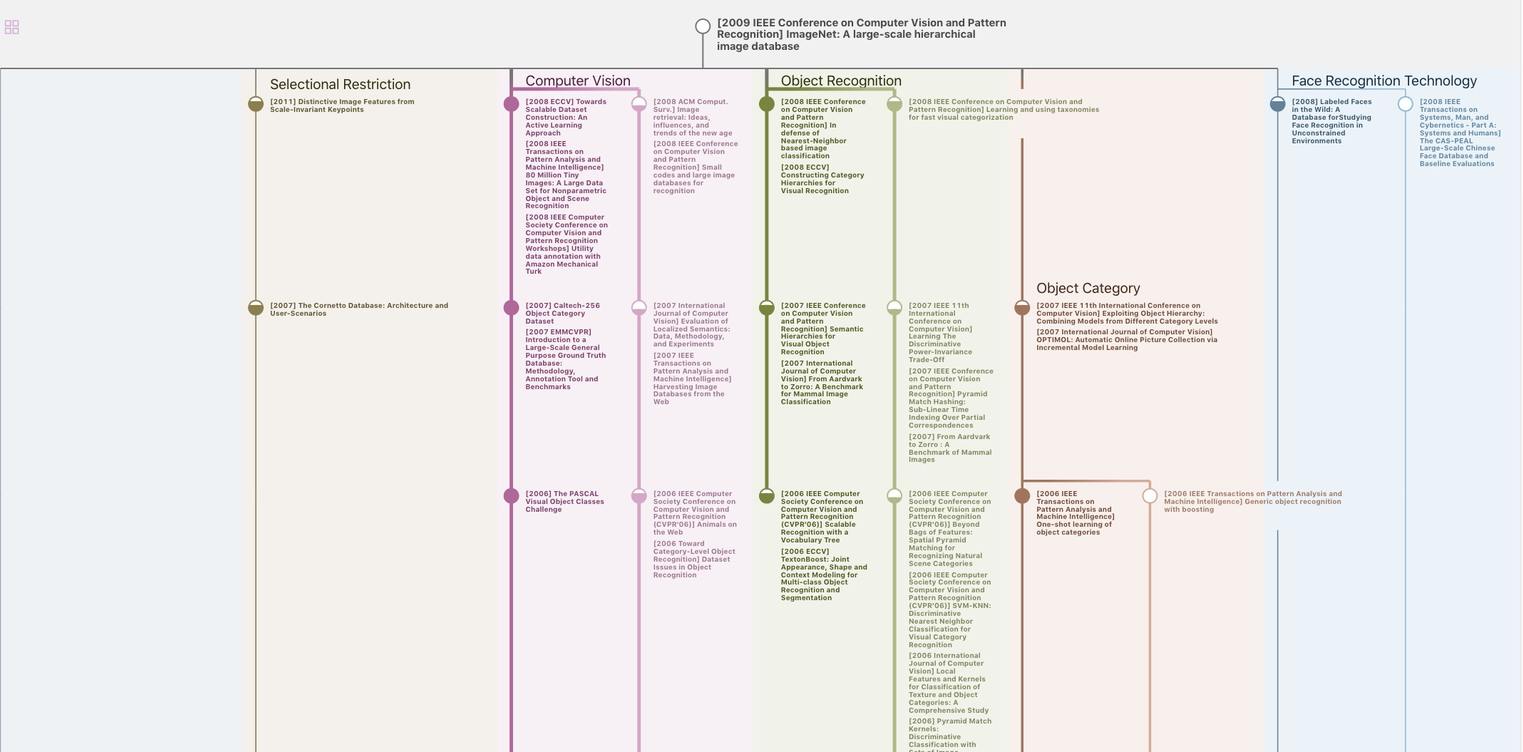
生成溯源树,研究论文发展脉络
Chat Paper
正在生成论文摘要