Self-Supervised Segmentation of 3D Fluorescence Microscopy Images Using CycleGAN
2023 45TH ANNUAL INTERNATIONAL CONFERENCE OF THE IEEE ENGINEERING IN MEDICINE & BIOLOGY SOCIETY, EMBC(2023)
摘要
In recent years, deep learning models have been extensively applied for the segmentation of microscopy images to efficiently and accurately quantify and characterize cells, nuclei, and other biological structures. However, typically these are supervised models that require large amounts of training data that are manually annotated to create the ground-truth. Since manual annotation of these segmentation masks is difficult and time-consuming, specially in 3D, we sought to develop a self-supervised segmentation method. Our method is based on an image-to-image translation model, the CycleGAN, which we use to learn the mapping from the fluorescence microscopy images domain to the segmentation domain. We exploit the fact that CycleGAN does not require paired data and train the model using synthetic masks, instead of manually labeled masks. These masks are created automatically based on the approximate shapes and sizes of the nuclei and Golgi, thus manual image segmentation is not needed in our proposed approach. The experimental results obtained with the proposed CycleGAN model are compared with two well-known supervised segmentation models: 3D U-Net [1] and Vox2Vox [2]. The CycleGAN model led to the following results: Dice coefficient of 78.07% for the nuclei class and 67.73% for the Golgi class with a difference of only 1.4% and 0.61% compared to the best results obtained with the supervised models Vox2Vox and 3D U-Net, respectively. Moreover, training and testing the CycleGAN model is about 5.78 times faster in comparison with the 3D U-Net model. Our results show that without manual annotation effort we can train a model that performs similarly to supervised models for the segmentation of organelles in 3D microscopy images.
更多查看译文
AI 理解论文
溯源树
样例
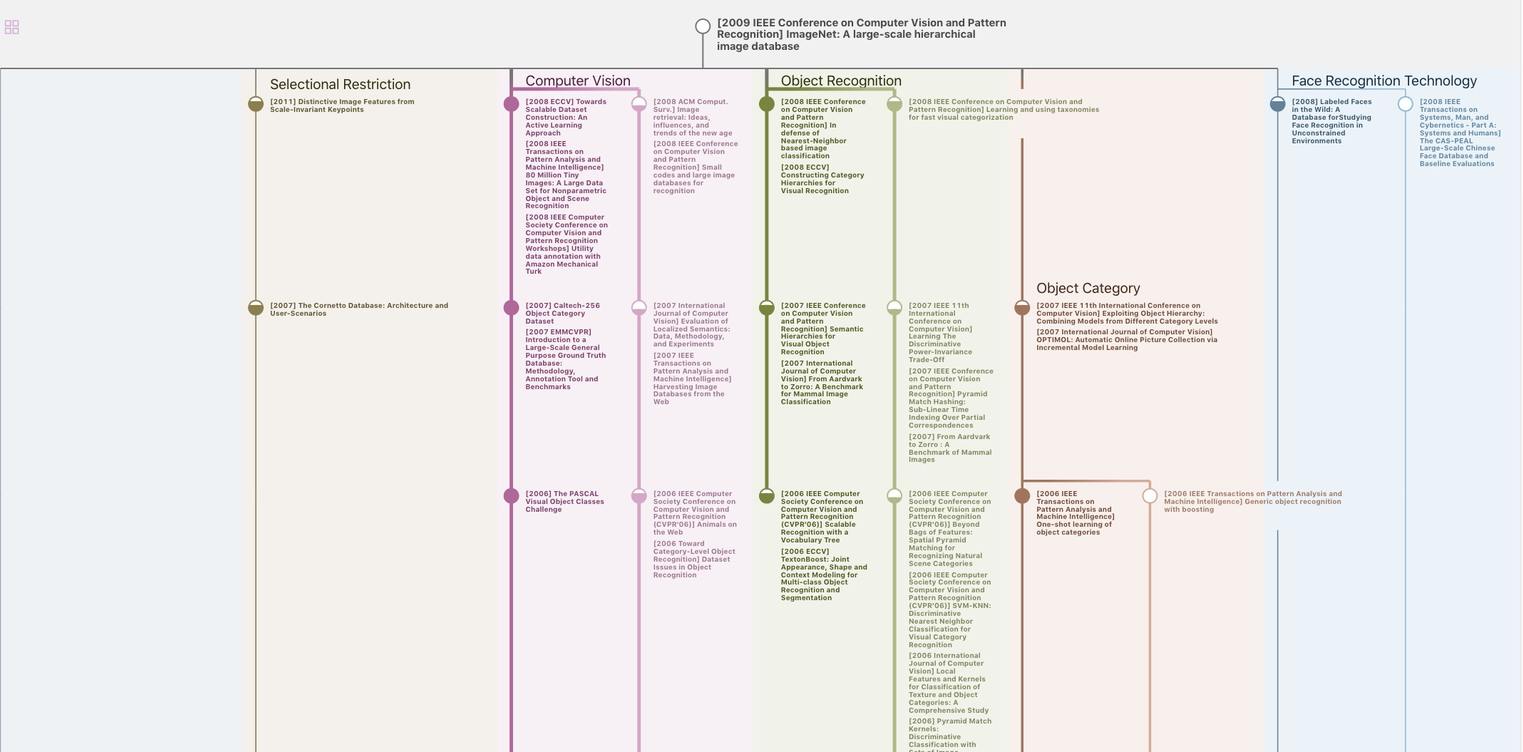
生成溯源树,研究论文发展脉络
Chat Paper
正在生成论文摘要