Gender-Specific Machine Learning Models to Predict Unplanned Return to Operating Room Following Primary Total Shoulder Arthroplasty.
2023 IEEE 11th International Conference on Healthcare Informatics (ICHI)(2023)
摘要
Reoperation is one of the most significant complications following any surgical procedure. Developing machine learning strategies that can predict the need for reoperation involves building computational models to understand if patients are likely to require a second surgery after an initial surgical procedure. These models use machine learning algorithms to analyze patient data, including demographics, medical history, lab results, and prior complications, to identify those at higher risk for complications or additional procedures. Such models offer potentials to healthcare providers to take proactive measures in reducing reoperation risks and improve patient outcomes. While several machine learning models have been developed to predict the need for a return to operating room (OR) within 30-days following primary total shoulder arthroplasty (TSA), implementation of gender-specific models has been very limited so far. This study aimed to build, train, and evaluate several return to OR predictive models trained on each gender separately and compare their accuracy performance with the traditional models trained on all genders together.
更多查看译文
关键词
Total Shoulder Arthroplasty,ML Fairness,Gender-Specific Models,Reoperation Risk Analysis
AI 理解论文
溯源树
样例
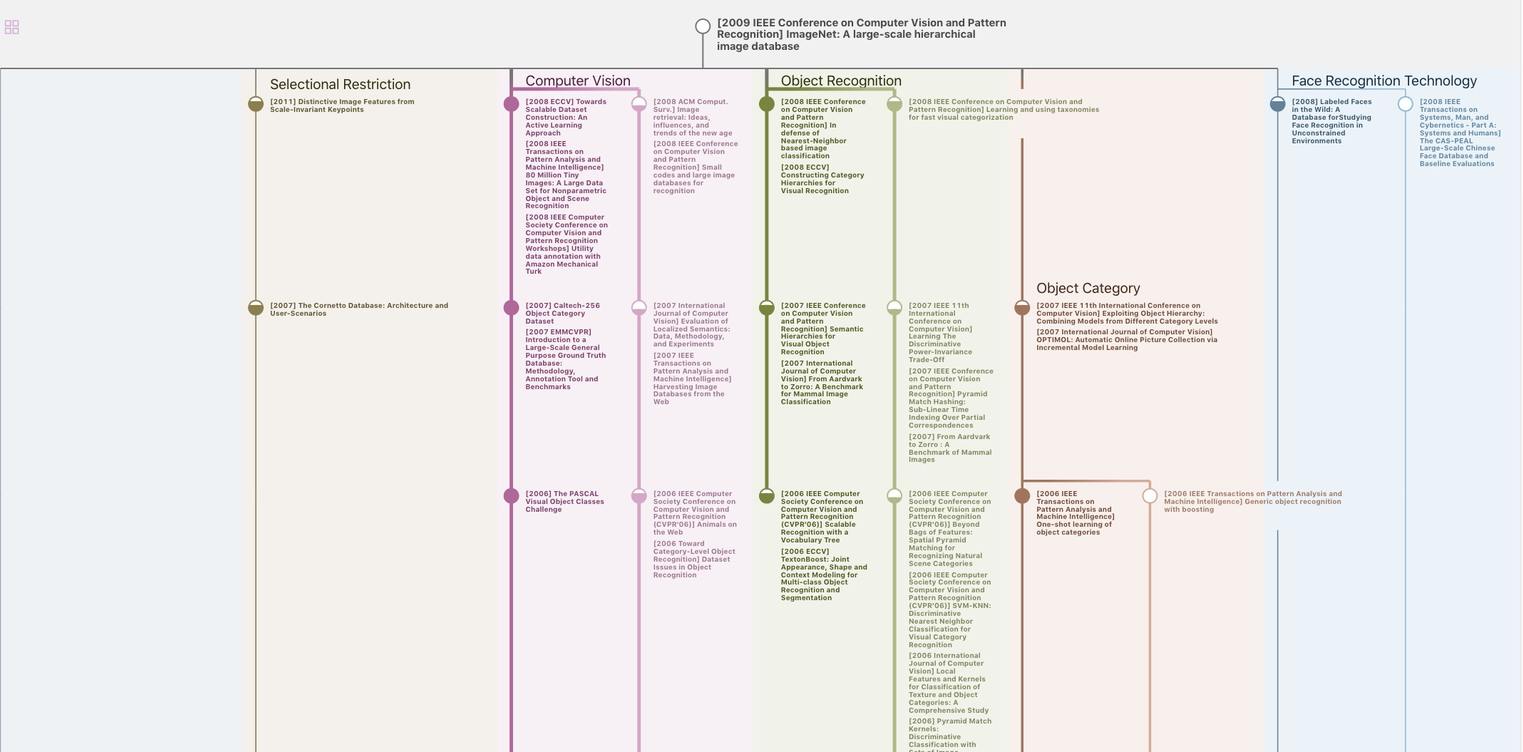
生成溯源树,研究论文发展脉络
Chat Paper
正在生成论文摘要