Automated Myocardial Infarction Screening Using Morphology-Based Electrocardiogram Biomarkers
2023 45TH ANNUAL INTERNATIONAL CONFERENCE OF THE IEEE ENGINEERING IN MEDICINE & BIOLOGY SOCIETY, EMBC(2023)
摘要
Ischemic heart disease (IHD), a critical and dreadful cardiovascular disease, is a leading cause of death globally. The steady progress of IHD leads to an irreversible condition called myocardial infarction (MI). The detection of MI can be done by observing the altered electrocardiogram (ECG) characteristics. Often, automated ECG analysis is preferred in place of visual inspection to reduce time and ensure reliable detection even when the recording quality is not very good. This paper presents an automated approach to classify recent MI, past MI, and normal sinus rhythm (NSR) classes based on the morphological features of the ECG. In clinical practice, a standard 12-lead ECG setup is typically employed to identify MI. However, acquiring a 12-lead ECG is not always convenient. Hence, in this study, we have explored the possibility of using a minimal number of ECG leads by deriving the augmented limb leads using leads I and II. A well-known and widely used ensemble machine learning tool, the random forest (RF) classifier is trained using features extracted from the derived augmented limb leads and their combinations. An RF classifier built using features extracted from all limb leads has outperformed classifiers built on combinations of them with five-fold cross-validation training accuracy of 97.9 (+/- 0.008) % and testing accuracy of 98 %.
更多查看译文
AI 理解论文
溯源树
样例
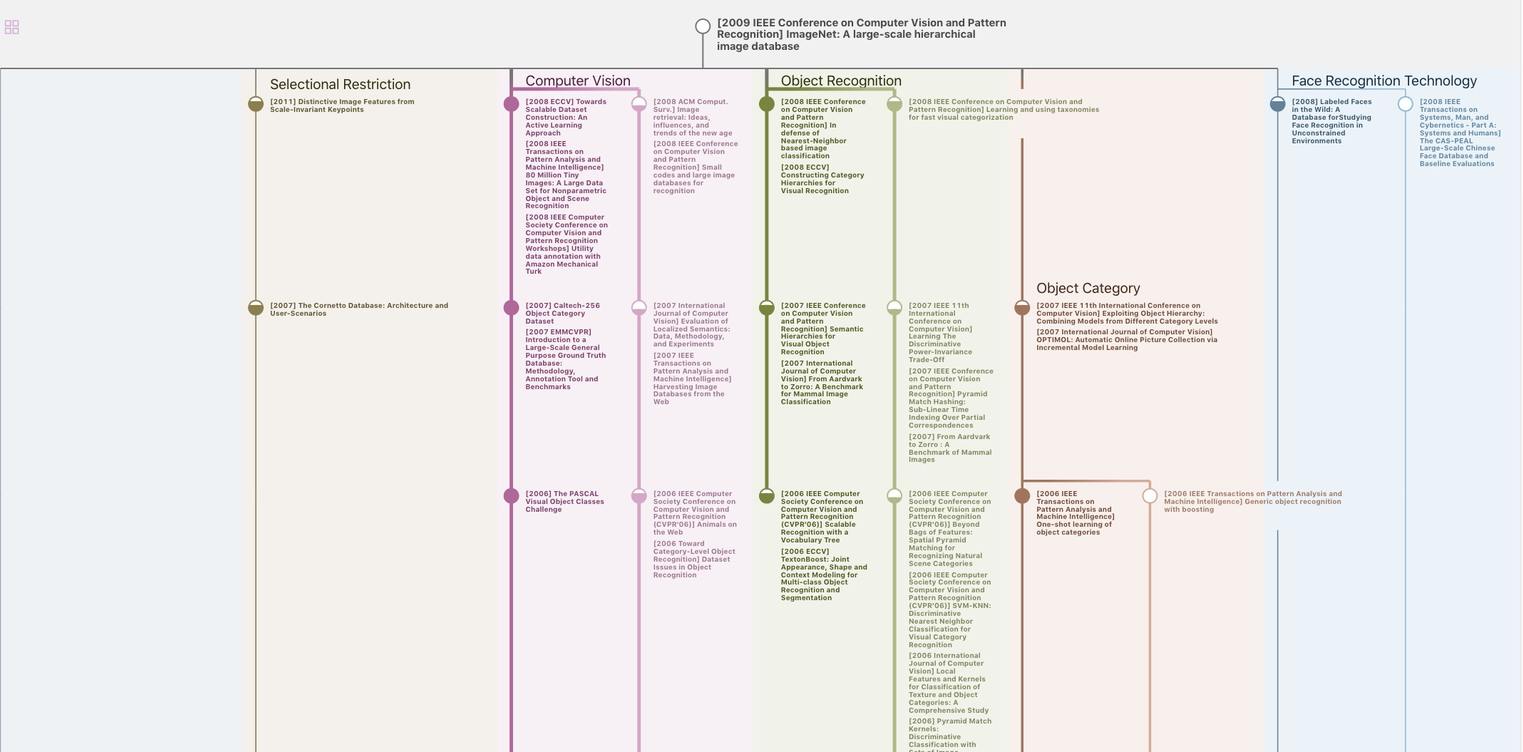
生成溯源树,研究论文发展脉络
Chat Paper
正在生成论文摘要