Exploring the Feasibility of Configured Grant for Vehicular Scenario
2023 IEEE 98TH VEHICULAR TECHNOLOGY CONFERENCE, VTC2023-FALL(2023)
摘要
Vehicular applications such as Augmented Reality (AR), Virtual Reality (VR), and High Definition Map (HD Map) are known for their latency-sensitive traits. But, dynamic scheduling at the MAC layer incurs significant signalling overhead (in terms of Scheduling Requests (SRs) in Uplink (UL)), leading to non-negligible latency in 5G NR. To address this issue, 5G NR introduces Configuration Grant (CG) for UL transmission, which pre-allocates radio resources to UEs (vehicles), thereby reducing signalling overhead between a vehicle and the Base Station (gNB). However, the high-speed mobility of vehicles results in rapid changes in channel conditions. Employing CG in a vehicular scenario can lead to incorrect assignment of transmission parameters (e.g., Modulation and Coding Scheme (MCS)), thereby adversely impacting the vehicles' Packet Delivery Ratio (PDR). To address this issue, this paper proposes a CG allocation algorithm that utilizes a Machine Learning (ML)-driven approach to predict the future MCS of vehicles. A data-driven ML model, derived from a real-world dataset, assists the radio resource scheduler and is evaluated using the NS-3 5G-LENA CG module. The ML-assisted CG allocation algorithm demonstrates significant improvements in terms of PDR and spectrum usage efficiency in vehicular scenarios.
更多查看译文
关键词
Configured Grant,Vehicular Applications,Machine Learning,Radio Resource Scheduling
AI 理解论文
溯源树
样例
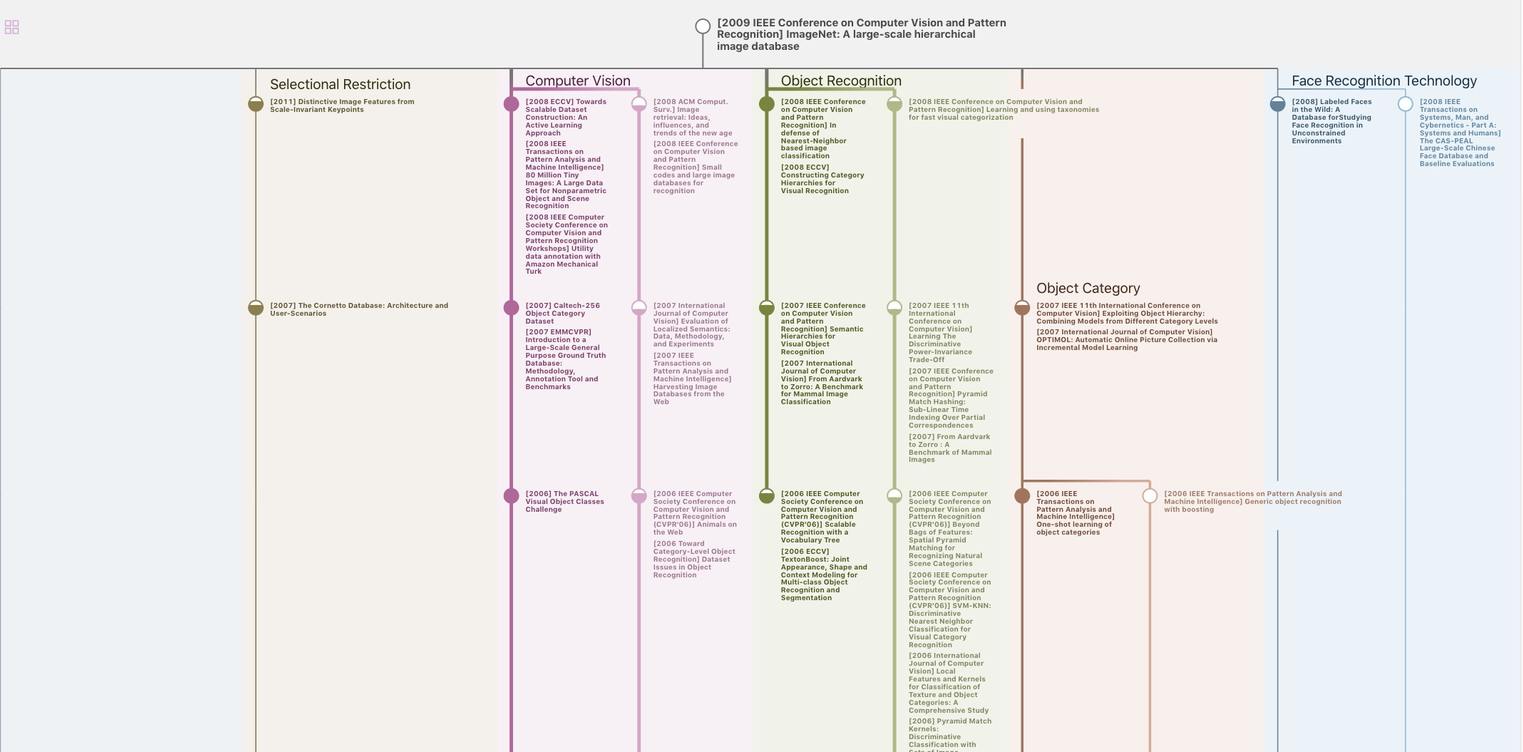
生成溯源树,研究论文发展脉络
Chat Paper
正在生成论文摘要