A novel framework for differentiating vessel-like objects in coronarography images
2023 45TH ANNUAL INTERNATIONAL CONFERENCE OF THE IEEE ENGINEERING IN MEDICINE & BIOLOGY SOCIETY, EMBC(2023)
摘要
Coronary Artery Disease is the leading cause of death worldwide. Its prevalence will grow while access to specialized medical care will be further limited due to staff shortages. Therefore, any facilitation of diagnosis or treatment is of paramount importance. The diagnosis based on Coronary Angiography can be automated to perform a quantitative evaluation of lesions. This requires precise segmentation of coronary arteries. At the moment, the state-of-the-art algorithms fail to eliminate vessel-like artifacts that are wrongly included in segmentation results (e.g. catheters, stitches). This is a bottleneck for the automatization of the diagnosis workflow that precedes clinical action. In this paper, we propose a 2-step post-segmentation refinement algorithm. A binary segmentation of the coronary arteries is used to extract image features - inputs for an XGBoost Classifier. Its predictions are improved by a neighborhood filter that leverages contextual information to assign correct labels. The algorithm is primarily concerned with differentiating vessels from other vessel-like objects and does so with a 99% accuracy rate. It takes advantage of an original local description of Tamura features, which proved to be one of the most influential factors in decision-making. As a result, the segmentation of coronary arteries is cleaned from artifacts, enabling AI-supported diagnosis workflows to be automated. After re-training, the proposed method can be used to eliminate post-segmentation artifacts in other medical domains.
更多查看译文
关键词
Coronary Artery Diseases,Coronarography,Coronary Artery Segmentation,local Tamura features,boosted trees,pixel classification,segmentation refinement
AI 理解论文
溯源树
样例
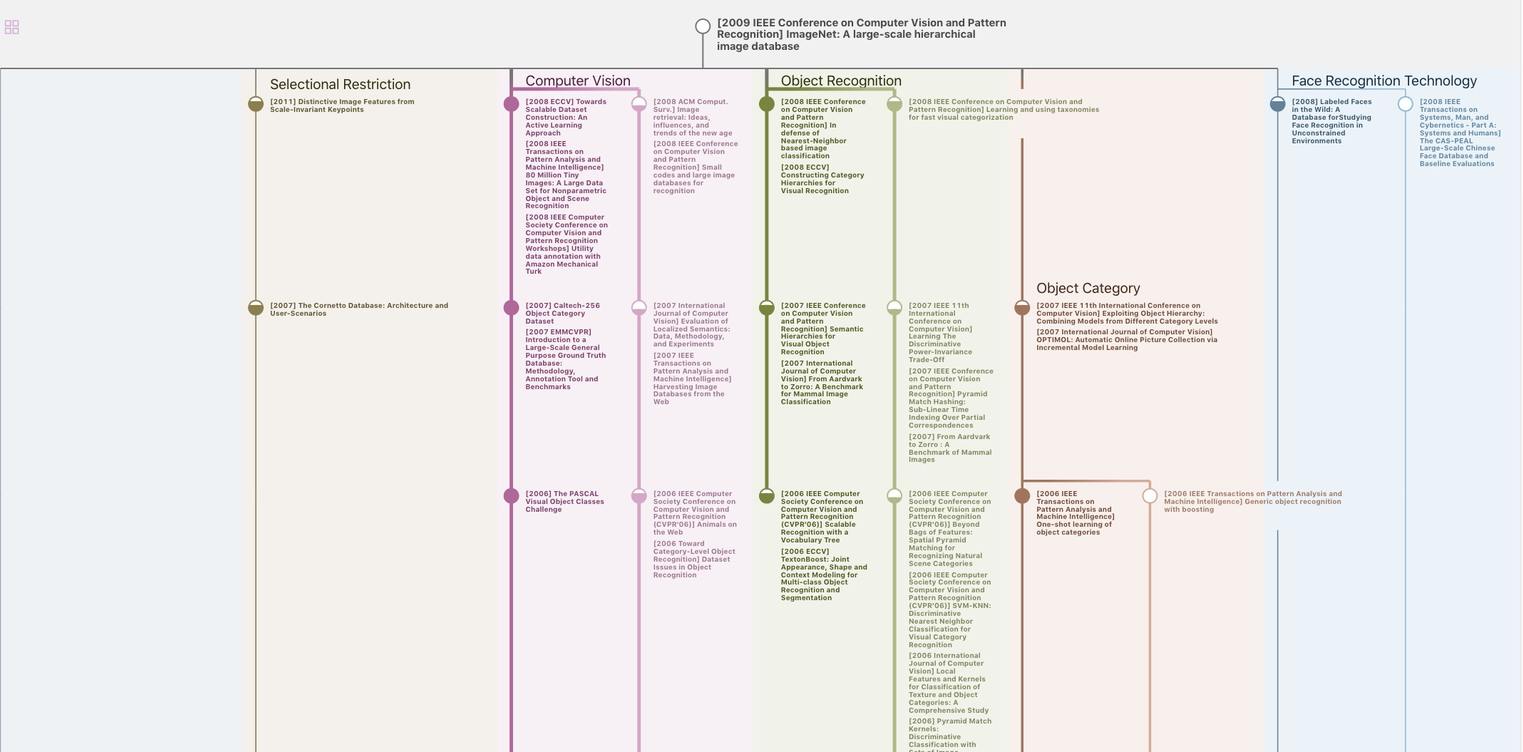
生成溯源树,研究论文发展脉络
Chat Paper
正在生成论文摘要