Generalizability of Human Activity Recognition Machine Learning Models from non-Parkinson's to Parkinson's Disease Patients
2023 45TH ANNUAL INTERNATIONAL CONFERENCE OF THE IEEE ENGINEERING IN MEDICINE & BIOLOGY SOCIETY, EMBC(2023)
摘要
Recent evidence shows that high-intensity exercises reduce tremors and stiffness in Parkinson's disease (PD). However, there is insufficient evidence on the types of exercises; in effect, high-intensity may be a personalized measure. Recent progress in automated Human Activity Recognition using machine learning (ML) models shows potential for better monitoring of PD patients. However, ML models must be calibrated to ignore tremors and accurately identify activity and its intensity. We report findings from a study where we trained ML models using data from medically validated triple synchronous sensors connected to 8 non-PD subjects performing 32 exercises. We then tested the models to identify exercises performed by 8 PD patients at different stages of the disease. Our analysis shows that better data preprocessing before modeling can provide some model generalizability. However, it is extremely challenging, as the models work with high accuracy on one group (Healthy or PD patients) (F1=0.88-0.94) but not on both groups.
更多查看译文
AI 理解论文
溯源树
样例
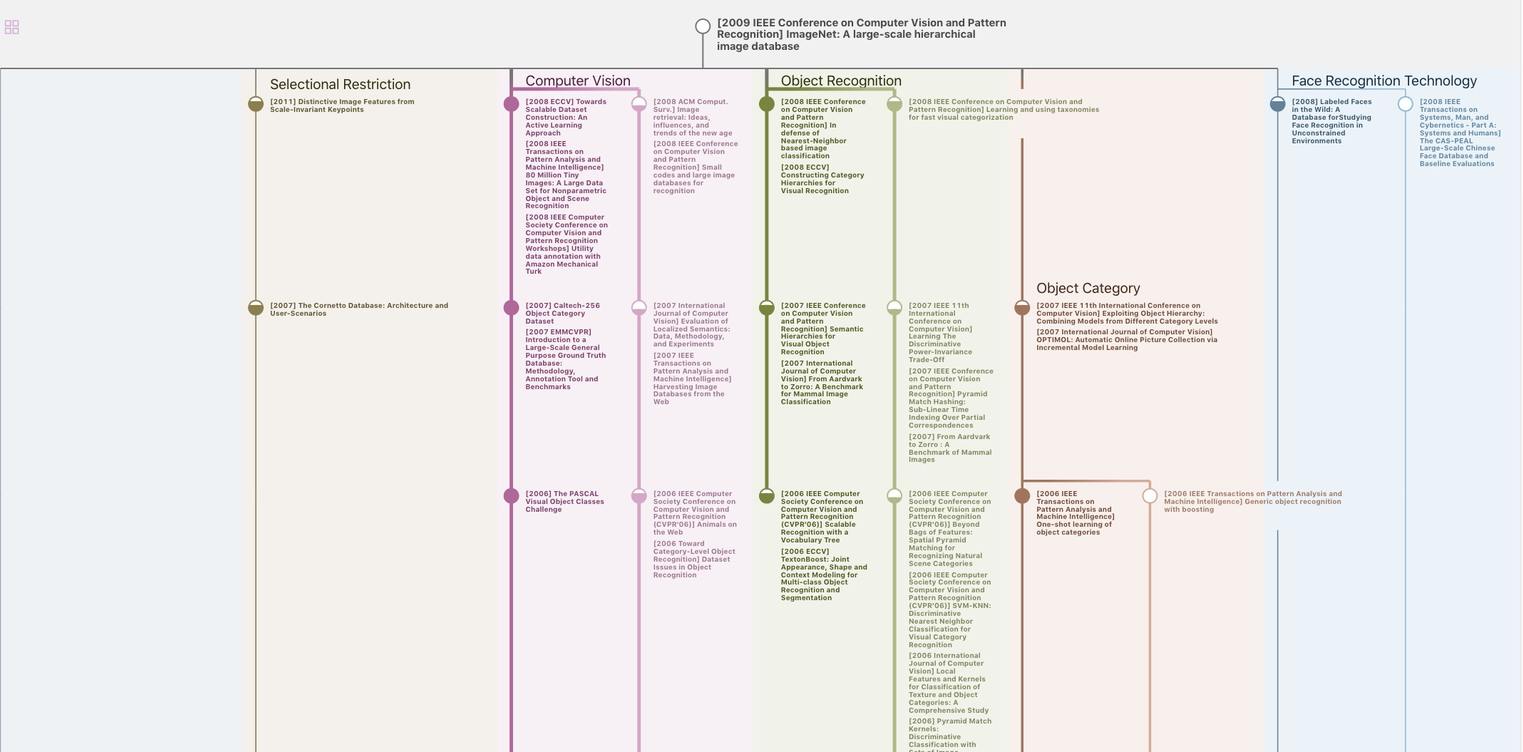
生成溯源树,研究论文发展脉络
Chat Paper
正在生成论文摘要