Memory Classifiers for Robust ECG Classification against Physiological Noise
2023 45TH ANNUAL INTERNATIONAL CONFERENCE OF THE IEEE ENGINEERING IN MEDICINE & BIOLOGY SOCIETY, EMBC(2023)
摘要
The development of sophisticated machine learning algorithms has made it possible to detect critical health conditions like cardiac arrhythmia, directly from electrocardiogram (ECG) recordings. Large-scale machine learning models, like deep neural networks, are well known to underperform when subjected to small perturbations which would not pose a challenge to physicians. This is a hurdle that needs to be removed to facilitate wide-scale adoption. We find this to be true even for models trained using data-augmentation schemes. In this paper, we show that using memory classifiers it is possible to attain a boost in robustness using expert-informed features. Memory classifiers combine standard deep neural network training with a domain knowledge-guided similarity metric to boost the robustness of classifiers. We evaluate the performance of the models against naturally occurring physiological perturbations, specifically electrode movement, muscle artifact, and baseline wander noise. Our approach demonstrates improved robustness across all evaluated noises for an average improvement in F1 score of 3.13% compared to models using data augmentation techniques.
更多查看译文
AI 理解论文
溯源树
样例
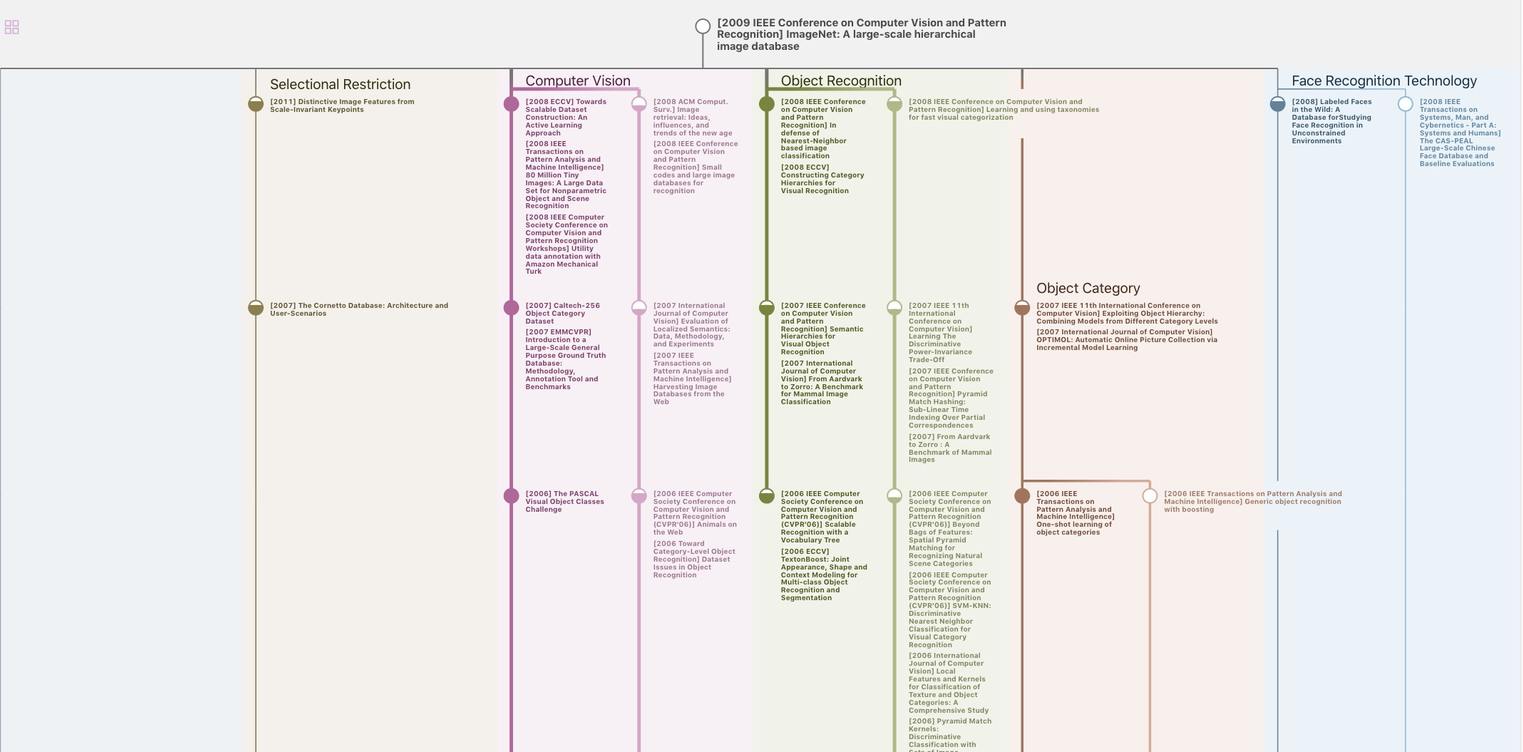
生成溯源树,研究论文发展脉络
Chat Paper
正在生成论文摘要