Predicting pathological complete response based on weakly and semi-supervised joint learning from breast cancer MRI
2023 45TH ANNUAL INTERNATIONAL CONFERENCE OF THE IEEE ENGINEERING IN MEDICINE & BIOLOGY SOCIETY, EMBC(2023)
摘要
Neoadjuvant chemotherapy (NAC) is the standard treatment for breast cancer patients. Patients achieving complete pathological response (pCR) after NAC usually have a good prognosis. However, automatic pCR prediction has been a challenging problem due to lacking well annotations in 3D MRI. Thus far, unifying different annotation information to predict the tumor's early response to NAC has not been systematically addressed. This paper proposes a weakly and semi-supervised joint learning method that integrates attentional features from multi-parametric MRI with radiomic features for predicting pCR to NAC in breast cancer patients. The attention-based multi-instance learning (MIL) is first developed to generate informative MRI bag-level features and mine key instances. The mean-teacher framework is then employed to segment tumor regions in a semi-supervised setting for extracting radiomic features. We perform experiments on 442 patients' data and show that our method achieves an AUC value of 0.85 in pCR prediction, which is superior to comparative methods. It is also shown that learning from multi-parametric MRI outperforms that of single-parameter MRI in pCR prediction.
更多查看译文
关键词
Weakly supervised learning,Attention,Semi-supervised learning,Pathological complete response
AI 理解论文
溯源树
样例
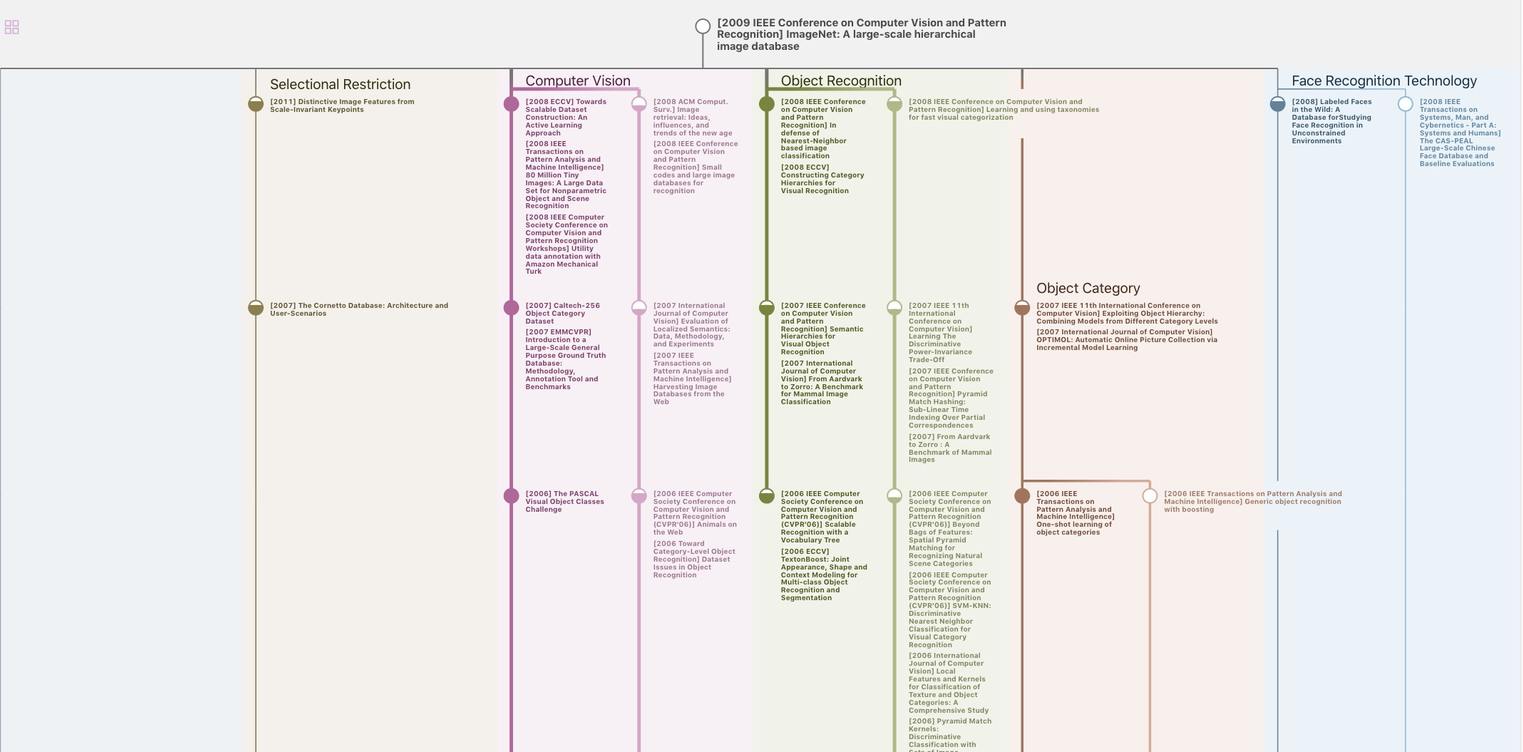
生成溯源树,研究论文发展脉络
Chat Paper
正在生成论文摘要