An Optimized Federated Learning Approach with the Data-Sharing Function to the Analysis of Cardiothoracic Time-Series Signals
2023 45TH ANNUAL INTERNATIONAL CONFERENCE OF THE IEEE ENGINEERING IN MEDICINE & BIOLOGY SOCIETY, EMBC(2023)
摘要
Machine/deep learning has been widely used for big data analysis in the field of healthcare, but it is still a question to ensure both computation efficiency and data security/confidentiality for the protection of private information. Referring to the data-sharing function of the federated learning (FedL) model, we propose an optimized data-sharing FedL (DSFedL) framework via a data-sharing hub by evaluating an accuracy-privacy loss function. When applied to the derived non-identically and independently distributed (non-IID) datasets simulated from three open-source cardiothoracic databases (i.e., ICBHI, Coswara COVID-19, MIT-BIH Arrhythmia), our optimized DSFedL works efficiently and the results show an optimal outcome of both the accuracy/efficiency and data security/confidentiality management.
更多查看译文
AI 理解论文
溯源树
样例
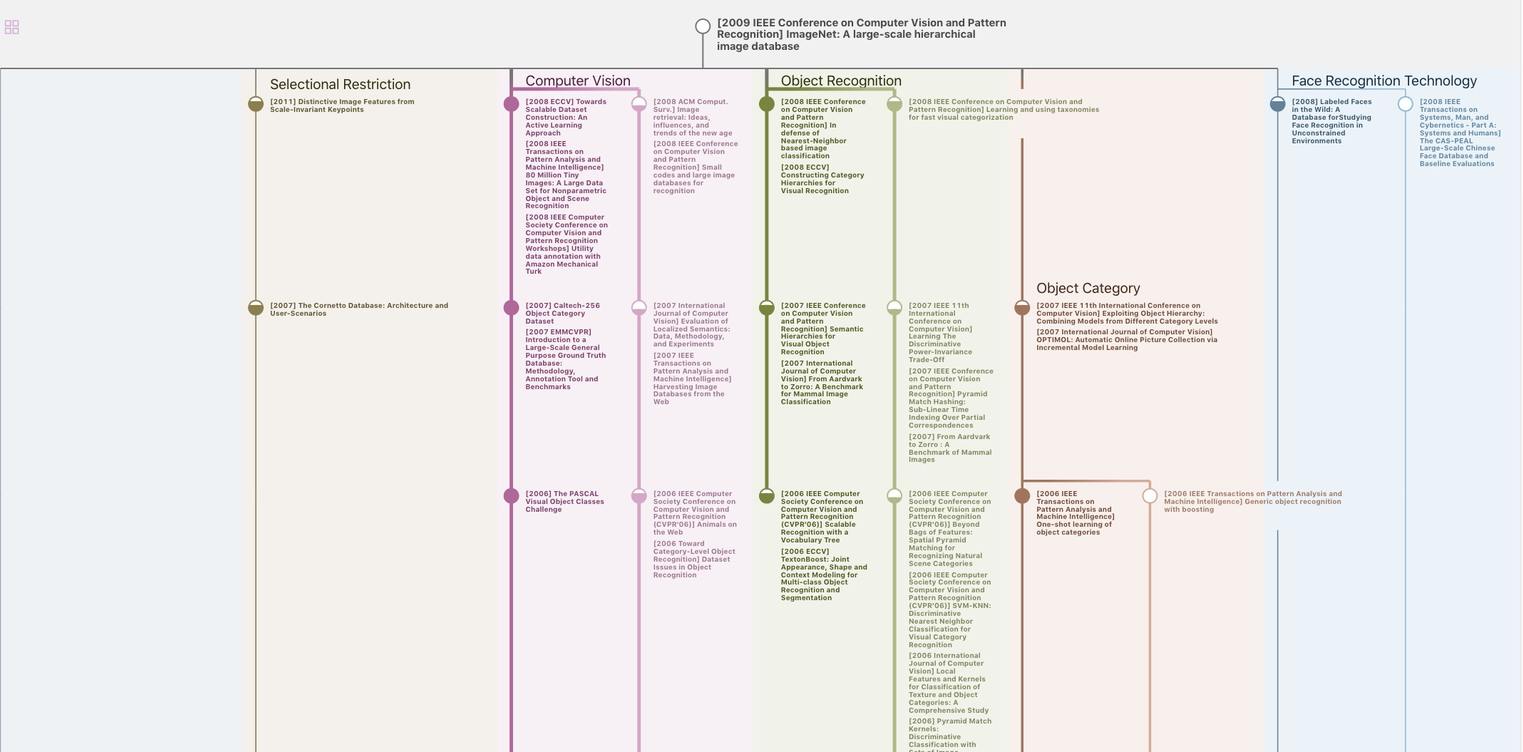
生成溯源树,研究论文发展脉络
Chat Paper
正在生成论文摘要