Automatic Contrast Generation from Contrastless Computed Tomography
2023 45TH ANNUAL INTERNATIONAL CONFERENCE OF THE IEEE ENGINEERING IN MEDICINE & BIOLOGY SOCIETY, EMBC(2023)
Abstract
The use of contrast-enhanced computed tomography (CTCA) for detection of coronary artery disease (CAD) exposes patients to the risks of iodine contrast-agents and excessive radiation, increases scanning time and healthcare costs. Deep learning generative models have the potential to artificially create a pseudo-enhanced image from non-contrast computed tomography (CT) scans. In this work, two specific models of generative adversarial networks (GANs) - the Pix2Pix-GAN and the Cycle-GAN - were tested with paired non-contrasted CT and CTCA scans from a private and public dataset. Furthermore, an exploratory analysis of the trade-off of using 2D and 3D inputs and architectures was performed. Using only the Structural Similarity Index Measure (SSIM) and the Peak Signal-to-Noise Ratio (PSNR), it could be concluded that the Pix2Pix-GAN using 2D data reached better results with 0.492 SSIM and 16.375 dB PSNR. However, visual analysis of the output shows significant blur in the generated images, which is not the case for the Cycle-GAN models. This behavior can be captured by the evaluation of the Fr ' echet Inception Distance (FID), that represents a fundamental performance metric that is usually not considered by related works in the literature.
MoreTranslated text
AI Read Science
Must-Reading Tree
Example
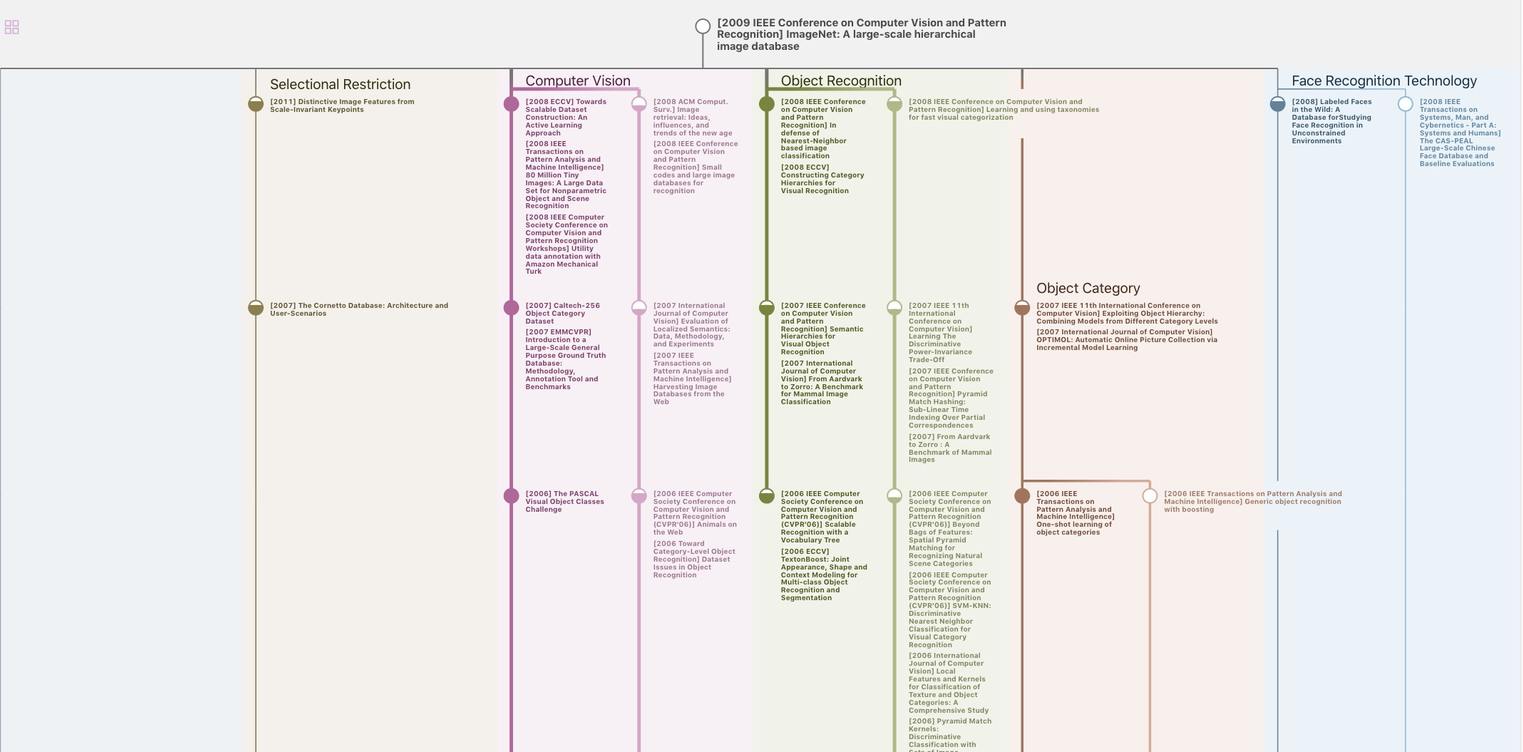
Generate MRT to find the research sequence of this paper
Chat Paper
Summary is being generated by the instructions you defined